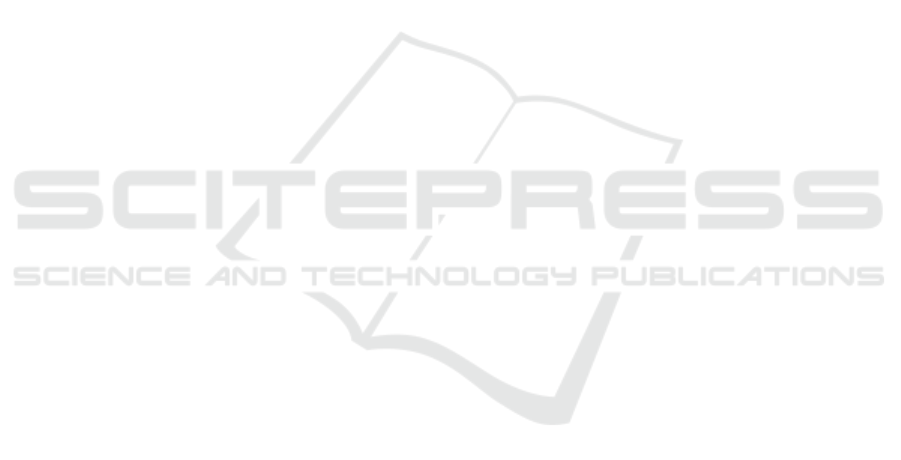
F., Ravelli, F., and Santone, A. (2022a). Explainable
deep learning for alzheimer disease classification and
localisation. In International Conference on Applied
Intelligence and Informatics, 1-3 September, Reggio
Calabria, Italy. in press.
Di Giammarco, M., Iadarola, G., Martinelli, F., Mercaldo,
F., and Santone, A. (2022b). Explainable retinopathy
diagnosis and localisation by means of class activation
mapping. In 2022 International Joint Conference on
Neural Networks (IJCNN), pages 1–8. IEEE.
Di Giammarco, M., Santone, A., Cesarelli, M., Martinelli,
F., and Mercaldo, F. (2024b). Evaluating deep learn-
ing resilience in retinal fundus classification with gen-
erative adversarial networks generated images. Elec-
tronics, 13(13):2631.
Diaz-Pinto, A., Colomer, A., Naranjo, V., Morales, S., Xu,
Y., and Frangi, A. F. (2019). Retinal image syn-
thesis and semi-supervised learning for glaucoma as-
sessment. IEEE transactions on medical imaging,
38(9):2211–2218.
Fang, W., Zhang, F., Sheng, V. S., and Ding, Y. (2018).
A method for improving cnn-based image recogni-
tion using dcgan. Computers, Materials & Continua,
57(1).
Goodfellow, I., Pouget-Abadie, J., Mirza, M., Xu, B.,
Warde-Farley, D., Ozair, S., Courville, A., and Ben-
gio, Y. (2020). Generative adversarial networks. Com-
munications of the ACM, 63(11):139–144.
He, K., Zhang, X., Ren, S., and Sun, J. (2016). Deep resid-
ual learning for image recognition. In Proceedings of
the IEEE conference on computer vision and pattern
recognition, pages 770–778.
Howard, A. G., Zhu, M., Chen, B., Kalenichenko, D.,
Wang, W., Weyand, T., Andreetto, M., and Adam,
H. (2017). Mobilenets: Efficient convolutional neu-
ral networks for mobile vision applications. arXiv
preprint arXiv:1704.04861.
Huang, P., Xiao, H., He, P., Li, C., Guo, X., Tian, S.,
Feng, P., Chen, H., Sun, Y., Mercaldo, F., et al.
(2024). La-vit: A network with transformers con-
strained by learned-parameter-free attention for in-
terpretable grading in a new laryngeal histopathol-
ogy image dataset. IEEE Journal of Biomedical and
Health Informatics.
Liu, B., Lv, J., Fan, X., Luo, J., and Zou, T. (2022). Ap-
plication of an improved dcgan for image generation.
Mobile information systems, 2022(1):9005552.
Martinelli, F., Mercaldo, F., and Santone, A. (2022). Wa-
ter meter reading for smart grid monitoring. Sensors,
23(1):75.
Mercaldo, F., Ciaramella, G., Iadarola, G., Storto, M.,
Martinelli, F., and Santone, A. (2022). Towards ex-
plainable quantum machine learning for mobile mal-
ware detection and classification. Applied Sciences,
12(23):12025.
Mercaldo, F., Di Giammarco, M., Apicella, A., Di Iadarola,
G., Cesarelli, M., Martinelli, F., and Santone, A.
(2023). Diabetic retinopathy detection and diagno-
sis by means of robust and explainable convolutional
neural networks. Neural Computing and Applications,
35(23):17429–17441.
Mercaldo, F., Di Giammarco, M., Ravelli, F., Martinelli, F.,
Santone, A., and Cesarelli, M. (2024a). Alzheimer’s
disease evaluation through visual explainability by
means of convolutional neural networks. Interna-
tional Journal of Neural Systems, 34(2):2450007–
2450007.
Mercaldo, F., Martinelli, F., and Santone, A. (2024b).
Deep convolutional generative adversarial networks in
image-based android malware detection. Computers,
13(6).
Mirsky, Y., Mahler, T., Shelef, I., and Elovici, Y. (2019).
{CT-GAN}: Malicious tampering of 3d medical im-
agery using deep learning. In 28th USENIX Security
Symposium (USENIX Security 19), pages 461–478.
Nagaraju, R. and Stamp, M. (2022). Auxiliary-classifier
gan for malware analysis. In Artificial Intelligence for
Cybersecurity, pages 27–68. Springer.
Pan, H. and Xin, L. (2024). Fdts: A feature disentangled
transformer for interpretable squamous cell carcinoma
grading. IEEE/CAA Journal of Automatica Sinica,
12(JAS-2024-1027).
Singh, N. K. and Raza, K. (2020). Medical image gen-
eration using generative adversarial networks. arXiv
preprint arXiv:2005.10687.
Singh, N. K. and Raza, K. (2021). Medical image genera-
tion using generative adversarial networks: A review.
Health informatics: A computational perspective in
healthcare, pages 77–96.
Wang, X., Guo, H., Hu, S., Chang, M.-C., and Lyu, S.
(2023). Gan-generated faces detection: A survey and
new perspectives. ECAI 2023, pages 2533–2542.
Xia, X., Xu, C., and Nan, B. (2017). Inception-v3 for flower
classification. In 2017 2nd international conference
on image, vision and computing (ICIVC), pages 783–
787. IEEE.
You, A., Kim, J. K., Ryu, I. H., and Yoo, T. K. (2022).
Application of generative adversarial networks (gan)
for ophthalmology image domains: a survey. Eye and
Vision, 9(1):6.
Zhou, X., Tang, C., Huang, P., Tian, S., Mercaldo, F., and
Santone, A. (2023). Asi-dbnet: an adaptive sparse
interactive resnet-vision transformer dual-branch net-
work for the grading of brain cancer histopathological
images. Interdisciplinary Sciences: Computational
Life Sciences, 15(1):15–31.
BIOINFORMATICS 2025 - 16th International Conference on Bioinformatics Models, Methods and Algorithms
618