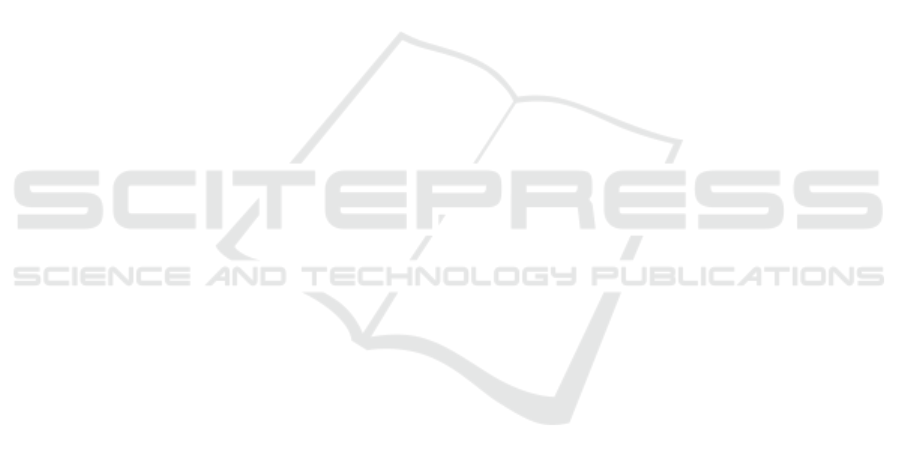
Castro-Neto, M., Jeong, Y.-S., Jeong, M.-K., and Han, L. D.
(2009). Online-svr for short-term traffic flow predic-
tion under typical and atypical traffic conditions. Ex-
pert systems with applications, 36(3):6164–6173.
Chen, W., Chen, L., Xie, Y., Cao, W., Gao, Y., and Feng,
X. (2019). Multi-range attentive bicomponent graph
convolutional network for traffic forecasting. In AAAI
Conference on Artificial Intelligence.
Cho, K., Van Merri
¨
enboer, B., Bahdanau, D., and Bengio,
Y. (2014a). On the properties of neural machine trans-
lation: Encoder-decoder approaches. arXiv preprint
arXiv:1409.1259.
Cho, K., Van Merri
¨
enboer, B., Gulcehre, C., Bahdanau, D.,
Bougares, F., Schwenk, H., and Bengio, Y. (2014b).
Learning phrase representations using rnn encoder-
decoder for statistical machine translation. arXiv
preprint arXiv:1406.1078.
Dougherty, M. S. and Cobbett, M. R. (1997). Short-term
inter-urban traffic forecasts using neural networks. In-
ternational journal of forecasting, 13(1):21–31.
Gori, M., Monfardini, G., and Scarselli, F. (2005). A new
model for learning in graph domains. In Proceedings.
2005 IEEE International Joint Conference on Neural
Networks, 2005., volume 2, pages 729–734 vol. 2.
Hochreiter, S. (1998). The vanishing gradient problem dur-
ing learning recurrent neural nets and problem solu-
tions. International Journal of Uncertainty, Fuzziness
and Knowledge-Based Systems, 6(02):107–116.
Hochreiter, S. and Schmidhuber, J. (1997). Long short-term
memory. Neural computation, 9(8):1735–1780.
Hua, J. and Faghri, A. (1994). Apphcations of artificial neu-
ral networks to intelligent vehicle-highway systems.
Transportation Research Record, 1453:83.
Huang, R., Huang, C., Liu, Y., Dai, G., and Kong, W.
(2020). Lsgcn: Long short-term traffic prediction with
graph convolutional networks. In IJCAI, volume 7,
pages 2355–2361.
Jiang, W. and Luo, J. (2022). Graph neural network for
traffic forecasting: A survey. Expert Systems with Ap-
plications, 207:117921.
Jin, X., Zhang, Y., and Yao, D. (2007). Simultaneously pre-
diction of network traffic flow based on pca-svr. In
Advances in Neural Networks–ISNN 2007: 4th Inter-
national Symposium on Neural Networks, ISNN 2007,
Nanjing, China, June 3-7, 2007, Proceedings, Part II
4, pages 1022–1031. Springer.
Kaffash, S., Nguyen, A. T., and Zhu, J. (2021). Big data al-
gorithms and applications in intelligent transportation
system: A review and bibliometric analysis. Interna-
tional Journal of Production Economics, 231:107868.
Kamarianakis, Y. and Prastacos, P. (2003). Forecasting traf-
fic flow conditions in an urban network: Compari-
son of multivariate and univariate approaches. Trans-
portation Research Record, 1857(1):74–84.
Karlaftis, M. G. and Vlahogianni, E. I. (2011). Statisti-
cal methods versus neural networks in transportation
research: Differences, similarities and some insights.
Transportation Research Part C: Emerging Technolo-
gies, 19(3):387–399.
Kipf, T. N. and Welling, M. (2017). Semi-Supervised Clas-
sification with Graph Convolutional Networks. In
Proceedings of the 5th International Conference on
Learning Representations, ICLR ’17.
Lecun, Y., Bottou, L., Bengio, Y., and Haffner, P. (1998).
Gradient-based learning applied to document recogni-
tion. Proceedings of the IEEE, 86(11):2278–2324.
Ledoux, C. (1997). An urban traffic flow model integrat-
ing neural networks. Transportation Research Part C:
Emerging Technologies, 5(5):287–300.
Lee, S. and Fambro, D. B. (1999). Application of subset
autoregressive integrated moving average model for
short-term freeway traffic volume forecasting. Trans-
portation research record, 1678(1):179–188.
Levin, M. and Tsao, Y.-D. (1980). On forecasting freeway
occupancies and volumes (abridgment). Transporta-
tion Research Record, (773).
Levy, J. I., Buonocore, J. J., and Von Stackelberg, K. (2010).
Evaluation of the public health impacts of traffic con-
gestion: a health risk assessment. Environmental
health, 9:1–12.
Li, M. and Zhu, Z. (2021). Spatial-temporal fusion graph
neural networks for traffic flow forecasting. Proceed-
ings of the AAAI Conference on Artificial Intelligence,
35(5):4189–4196.
Li, Y. and Shahabi, C. (2018). A brief overview of machine
learning methods for short-term traffic forecasting and
future directions. Sigspatial Special, 10(1):3–9.
Li, Y., Yu, R., Shahabi, C., and Liu, Y. (2018). Diffusion
convolutional recurrent neural network: Data-driven
traffic forecasting. In International Conference on
Learning Representations.
Lu, H., Huang, D., Song, Y., Jiang, D., Zhou, T., and Qin, J.
(2020). St-trafficnet: A spatial-temporal deep learning
network for traffic forecasting. Electronics, 9(9).
Luong, M.-T., Pham, H., and Manning, C. D. (2015). Ef-
fective approaches to attention-based neural machine
translation. arXiv preprint arXiv:1508.04025.
Qi, J., Zhao, Z., Tanin, E., Cui, T., Nassir, N., and Sarvi,
M. (2022). A graph and attentive multi-path convo-
lutional network for traffic prediction. IEEE Transac-
tions on Knowledge and Data Engineering.
Rahmani, S., Baghbani, A., Bouguila, N., and Patterson, Z.
(2023). Graph neural networks for intelligent trans-
portation systems: A survey. IEEE Transactions on
Intelligent Transportation Systems, 24(8):8846–8885.
Roy, A., Roy, K. K., Ahsan Ali, A., Amin, M. A., and Rah-
man, A. K. M. M. (2021). Sst-gnn: Simplified spatio-
temporal traffic forecasting model using graph neural
network. In Karlapalem, K., Cheng, H., Ramakrish-
nan, N., Agrawal, R. K., Reddy, P. K., Srivastava, J.,
and Chakraborty, T., editors, Advances in Knowledge
Discovery and Data Mining, pages 90–102, Cham.
Springer International Publishing.
Rumelhart, D. E., Hinton, G. E., and Williams, R. J. (1986).
Learning representations by back-propagating errors.
nature, 323(6088):533–536.
Su, H., Zhang, L., and Yu, S. (2007). Short-term traffic
flow prediction based on incremental support vector
VEHITS 2025 - 11th International Conference on Vehicle Technology and Intelligent Transport Systems
52