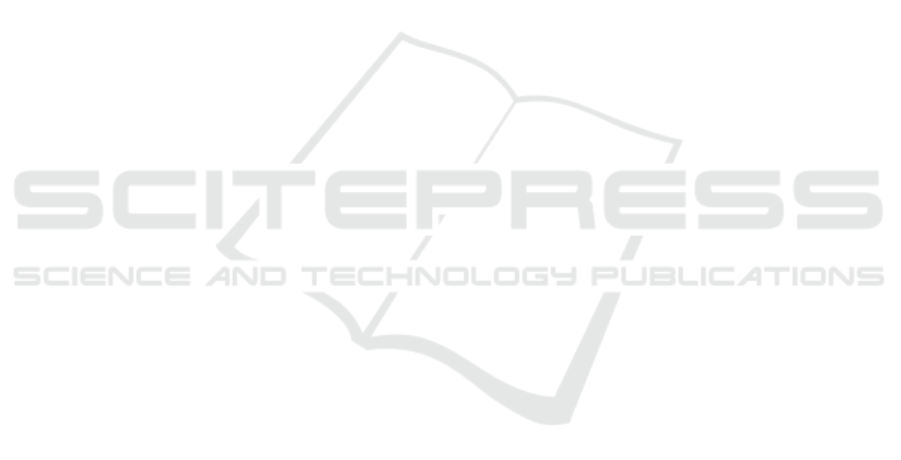
tentially providing stronger insights into the common
features that should be considered when estimating
velocity. Therefore, further research with additional
datasets from industrial projects is necessary to deter-
mine whether our findings are also applicable in an
industrial context.
6 CONCLUSION
Agile methodologies are widely applied, being one of
the most adopted practices the iterative development.
In this work, we have proposed the use of feature sub-
set selection to reduce the complexity of the machine
learning models often used in the literature to predict
software effort estimation. We have statistically tested
that reducing the feature subset to 10% of its orig-
inal size can be done without incrementing the ob-
tained error measures. With a minimal subset of fea-
tures, we have applied state-of-art global explanabil-
ity methods. Specifically, we made use of SHAP val-
ues to analyze the topmost important features that in-
fluence the difference between the committed and the
actual velocity of an iteration. We find out that there
are common features that appear in multiple projects,
which can be grouped into categories that help us un-
derstand their effects on the predicted velocity.
In future works, we plan to expand this study with
datasets from industrial projects, to assess whether
our findings can be extended to wider contexts. Fur-
thermore, it might be interesting to explore the effect
on vel
di f f
through the interaction of two or more fea-
tures, rather than considering only univariate effects.
ACKNOWLEDGEMENTS
This work has been partially funded by the
Government of Castilla-La Mancha and “ERDF
A way of making Europe” under project SB-
PLY/21/180225/000062 and by the Universidad de
Castilla-La Mancha and “ERDF A Way of Making
Europe” under project 2022-GRIN-34437.
REFERENCES
Abadeer, M. and Sabetzadeh, M. (2021). Machine learning-
based estimation of story points in agile development:
Industrial experience and lessons learned. In 2021
IEEE 29th International Requirements Engineering
Conference Workshops (REW), pages 106–115.
Abrahamsson, P., Fronza, I., Moser, R., Vlasenko, J., and
Pedrycz, W. (2011). Predicting development effort
from user stories. 2011 International Symposium on
Empirical Software Engineering and Measurement,
pages 400–403.
Abrahamsson, P., Moser, R., Pedrycz, W., Sillitti, A.,
and Succi, G. (2007). Effort prediction in iterative
software development processes – incremental versus
global prediction models. In First International Sym-
posium on Empirical Software Engineering and Mea-
surement (ESEM 2007), pages 344–353.
Aledo, J. A., G
´
amez, J. A., and Rosete, A. (2017). Utopia
in the solution of the bucket order problem. Decision
Support Systems, 97:69–80.
Ali, A. and Meil
˘
a, M. (2012). Experiments with kemeny
ranking: What works when? Mathematical Social
Sciences, 64(1):28–40. Computational Foundations of
Social Choice.
Alsaadi, B. and Saeedi, K. (2022). Data-driven effort esti-
mation techniques of agile user stories: a systematic
literature review, volume 55. Springer Netherlands.
Anderson, D. (2010). Kanban: Successful Evolutionary
Change for Your Technology Business. Blue Hole
Press.
Arcuri, A. and Briand, L. (2014). A hitchhiker’s guide to
statistical tests for assessing randomized algorithms in
software engineering. Software Testing, Verification
and Reliability, 24(3):219–250.
Bermejo, P., De La Ossa, L., and Puerta, J. M. (2011).
Global feature subset selection on high-dimensional
datasets using re-ranking-based edas. In Proceedings
of the 14th International Conference on Advances in
Artificial Intelligence: Spanish Association for Artifi-
cial Intelligence, page 54–63. Springer-Verlag.
Bhattacharya, P. and Neamtiu, I. (2011). Bug-fix time pre-
diction models: can we do better? In Proceedings
of the 8th Working Conference on Mining Software
Repositories, MSR ’11, page 207–210, New York,
NY, USA. Association for Computing Machinery.
Choetkiertikul, M., Dam, H. K., Tran, T., Ghose, A., and
Grundy, J. (2018). Predicting delivery capability in
iterative software development. IEEE Transactions on
Software Engineering, 44:551–573.
Choetkiertikul, M., Dam, H. K., Tran, T., Pham, T., Ghose,
A., and Menzies, T. (2019). A deep learning model for
estimating story points. IEEE Transactions on Soft-
ware Engineering, 45:637–656.
Cohn, M. (2004). User Stories Applied: For Agile Software
Development. Addison Wesley Longman Publishing
Co., Inc., USA.
Cohn, M. (2005). Agile Estimating and Planning. Prentice
Hall PTR, USA.
Digital.ai (2023). The 17th state of agile report.
Fu, M. and Tantithamthavorn, C. (2023). Gpt2sp: A
transformer-based agile story point estimation ap-
proach. IEEE Transactions on Software Engineering,
49(2):611–625.
Guyon, I. and Elisseeff, A. (2003). An introduction to
variable and feature selection. J. Mach. Learn. Res.,
3(null):1157–1182.
Hearty, P., Fenton, N., Marquez, D., and Neil, M. (2009).
Predicting project velocity in xp using a learning dy-
Agile Effort Estimation Improved by Feature Selection and Model Explainability
65