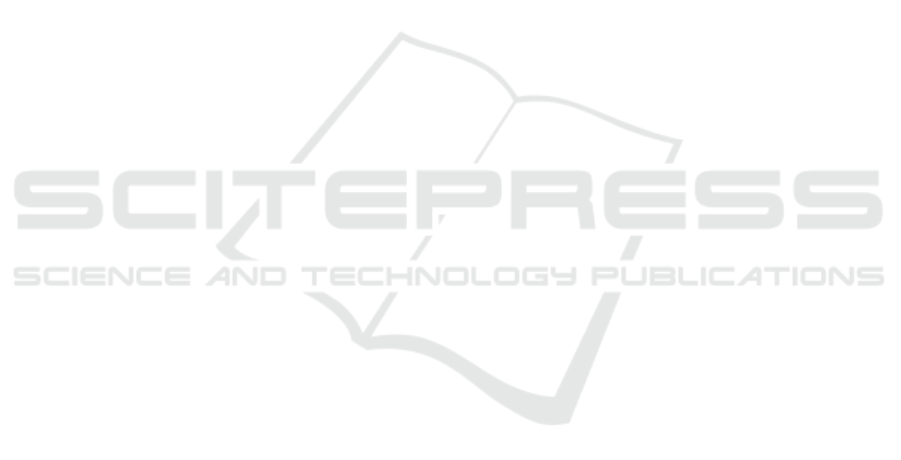
guided pull method. This allows for comparing the
two pull methods, outlining their advantages and dis-
advantages for the port.
In Figures 11 and 12, the standard seasonality
present at the port remains evident. However, larger
variations between peaks and valleys are observed in
the first figure compared to the second. This occurs
because in the second figure — where the guided pull
model carries out the pulls — the trucks enter oper-
ation more uniformly due to the consistency of the
pull method. Another important factor is the differ-
ence in the number of trucks in the “Exit Access Gate
(Client)” area, representing the number of trucks de-
livering products unloaded from the ships. This dif-
ference arises again due to the difference in pull pat-
terns. In Figure 11, where the pulls are performed by
the minimum pull model, the model determines that
the minimum required value of the “initial pull” pa-
rameter for port operations to be correctly executed
must be higher at the beginning of operations. On the
other hand, since the model considers the current state
of the port in the primary area, there is more signif-
icant fluctuation in the values of the pull parameters,
which is why there are moments with few or no trucks
in operation.
Based on the behaviors shown in both graphs, it
can be concluded that the minimum pull model serves
windows more efficiently. In Figure 11, port activities
are completed, while in Figure 12, they are still on-
going. Additionally, the significant difference in the
number of trucks delivering products to customers be-
tween the two graphs further supports this conclusion.
Figure 11 shows a considerably larger number of de-
liveries occurring in a short period. Therefore, the
minimum pull model proves to be an effective strat-
egy for managing port operations.
As the minimum queue size at the quay increases,
certain behaviors persist, as shown in Figure 13. This
figure is characterized by the minimum pull model,
while Figure 13 is characterized by the guided pull
model. There are two subtle differences between the
graphs in Figures 11 and 12 that are important to
highlight for the minimum pull model: first, in Fig-
ure 13, the number of trucks accumulating in the area
approaching the truck retention yard (TRY) is lower;
second, the time required to complete the active win-
dows is slightly shorter. These two factors suggest
that fewer trucks remain in the truck retention yard
despite minor fluctuations in the number of trucks in
the primary area and deliveries to customers. This
allows for greater continuity in the operational flow.
However, it is crucial also to analyze other factors,
such as idleness and the service distribution for active
windows.
The differences in fluctuations between the two
curves are significant, as shown in Figures 13 and
14. The first graph shows noticeable instances where
no trucks are in the primary area. This suggests that
the pull methodology used experiences various peri-
ods of idleness, indicating frequent drops in delivery
volume. In contrast, the second graph displays more
periodic and predictable behavior. It is more uniform
and shows a higher level of continuity in port activi-
ties. Therefore, although deliveries may be completed
later, the guided pull model ensures that the port re-
mains consistently active.
A significant difference between the two ap-
proaches is evident when examining how services are
distributed to the windows. The minimum pull model
completes all activities sooner but experiences sub-
stantial fluctuations in the number of trucks deliver-
ing to customers, which occur sporadically and un-
evenly, as shown in Figures 11 and 13. This indicates
that windows are served in an unequal and sequential
manner. In contrast, the guided pull model, illustrated
in Figures 12 and 14, distributes trucks more evenly
across the windows.
This analysis highlights the key factors that influ-
ence the minimum pull method, its effects on port
activities, and its advantages and disadvantages com-
pared to the guided pull model. While the minimum
pull method is more efficient regarding operational
time at the port, it often leads to idle periods and re-
sults in uneven fulfillment of port activities.
5 CONCLUSIONS
The results from the simulation of truck flow during
ship unloading at the Port of Itaqui highlight the sig-
nificance of effective resource management in reduc-
ing operational bottlenecks. Variations in factors such
as the number of trucks at docked ships and the avail-
ability of scales and loading arms directly affect the
unloading rate and the time trucks spend at the port.
It was observed that an overload of trucks, delays, and
poor distribution of activities within the port signifi-
cantly worsened congestion, negatively impacting lo-
gistical flow and overall operational efficiency. The
simulation proved to be an effective tool for predict-
ing and adjusting critical variables, allowing for de-
veloping strategies that enhance operational capacity
without compromising efficiency and safety in port
operations.
For future improvements, it would be beneficial
to include interruption events in the simulation, such
as equipment failures or adverse weather conditions,
as these factors can significantly affect the efficiency
Optimizing Truck Flow in Ship Unloading: A Real-Time Simulation Approach for the Port of Itaqui
453