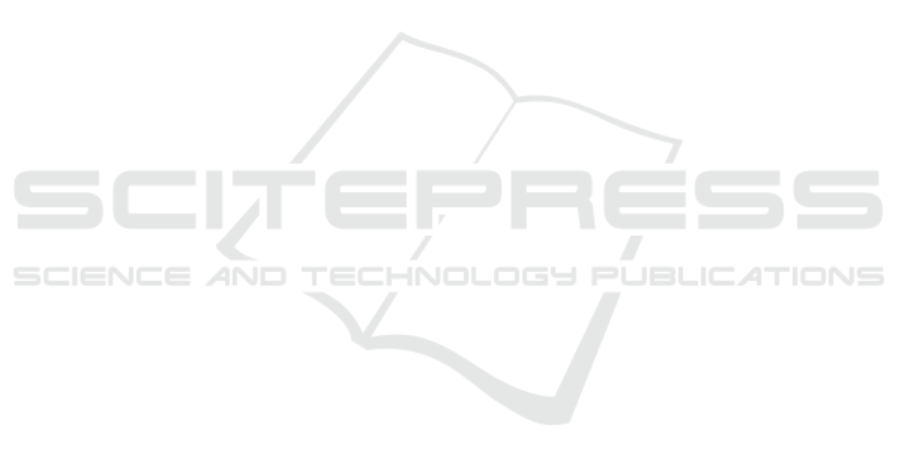
highlight the need for more sophisticated approaches
to accurately characterize non-boundary regions.
The training times for NVFi and the proposed
method using an RTX 3090 are shown in Table 2. In
the proposed method, the computation time increases
compared to NVFi because it requires calculating the
density values of neighboring regions. However, the
loss function based on the divergence theorem is ap-
plied only to 3D points with density values above a
threshold, assuming the presence of an object. Ex-
periments show that about 1% of all 3D points in the
scene exceed this threshold. As a result, the density
values of neighboring regions are calculated only for
3D points exceeding the threshold among the 262,144
sampled points. Therefore, the increase in computa-
tion time is less than initially expected.
6 CONCLUSION
This paper proposes a method to improve the quality
of synthesized frames by learning the geometry, ap-
pearance, and velocity of dynamic 3D scenes through
edge-aware sampling. We identified potential limita-
tions in accurately representing non-boundary char-
acteristics due to the min-max normalization process.
Future work will explore alternative approaches for
more accurate representation of non-boundary char-
acteristics. Furthermore, the extension of our method
to incorporate distance fields, enabling precise com-
putation of object boundary distances, presents a
promising avenue for future investigation.
REFERENCES
Cao, A. and Johnson, J. (2023). Hexplane: A fast repre-
sentation for dynamic scenes. In Proceedings of the
IEEE/CVF Conference on Computer Vision and Pat-
tern Recognition, pages 130–141.
Chen, R. T., Rubanova, Y., Bettencourt, J., and Duvenaud,
D. K. (2018). Neural ordinary differential equations.
Advances in neural information processing systems,
31.
Choy, C. B., Xu, D., Gwak, J., Chen, K., and Savarese,
S. (2016). 3d-r2n2: A unified approach for single
and multi-view 3d object reconstruction. In Computer
Vision–ECCV 2016: 14th European Conference, Am-
sterdam, The Netherlands, October 11-14, 2016, Pro-
ceedings, Part VIII 14, pages 628–644. Springer.
Chu, M., Liu, L., Zheng, Q., Franz, E., Seidel, H.-P.,
Theobalt, C., and Zayer, R. (2022). Physics informed
neural fields for smoke reconstruction with sparse
data. ACM Transactions on Graphics (ToG), 41(4):1–
14.
Fan, H., Su, H., and Guibas, L. J. (2017). A point set gener-
ation network for 3d object reconstruction from a sin-
gle image. In Proceedings of the IEEE conference on
computer vision and pattern recognition, pages 605–
613.
Fridovich-Keil, S., Meanti, G., Warburg, F. R., Recht, B.,
and Kanazawa, A. (2023). K-planes: Explicit radiance
fields in space, time, and appearance. In Proceedings
of the IEEE/CVF Conference on Computer Vision and
Pattern Recognition, pages 12479–12488.
Groueix, T., Fisher, M., Kim, V. G., Russell, B. C., and
Aubry, M. (2018). A papier-m
ˆ
ach
´
e approach to learn-
ing 3d surface generation. In Proceedings of the IEEE
conference on computer vision and pattern recogni-
tion, pages 216–224.
Kingma, D. P. and Ba, J. (2014). Adam: A
method for stochastic optimization. arXiv preprint
arXiv:1412.6980.
Li, J., Song, Z., and Yang, B. (2024). Nvfi: neural velocity
fields for 3d physics learning from dynamic videos.
Advances in Neural Information Processing Systems,
36.
Li, Z., Niklaus, S., Snavely, N., and Wang, O. (2021). Neu-
ral scene flow fields for space-time view synthesis of
dynamic scenes. In Proceedings of the IEEE/CVF
Conference on Computer Vision and Pattern Recog-
nition, pages 6498–6508.
Mildenhall, B., Srinivasan, P. P., Tancik, M., Barron, J. T.,
Ramamoorthi, R., and Ng, R. (2021). Nerf: Repre-
senting scenes as neural radiance fields for view syn-
thesis. Communications of the ACM, 65(1):99–106.
Park, K., Sinha, U., Barron, J. T., Bouaziz, S., Goldman,
D. B., Seitz, S. M., and Martin-Brualla, R. (2021).
Nerfies: Deformable neural radiance fields. In Pro-
ceedings of the IEEE/CVF International Conference
on Computer Vision, pages 5865–5874.
Pumarola, A., Corona, E., Pons-Moll, G., and Moreno-
Noguer, F. (2021). D-nerf: Neural radiance fields
for dynamic scenes. In Proceedings of the IEEE/CVF
Conference on Computer Vision and Pattern Recogni-
tion, pages 10318–10327.
Raissi, M., Perdikaris, P., and Karniadakis, G. (2019).
Physics-informed neural networks: A deep learn-
ing framework for solving forward and inverse prob-
lems involving nonlinear partial differential equations.
Journal of Computational Physics, 378:686–707.
Tatarchenko, M., Dosovitskiy, A., and Brox, T. (2017). Oc-
tree generating networks: Efficient convolutional ar-
chitectures for high-resolution 3d outputs. In Proceed-
ings of the IEEE international conference on com-
puter vision, pages 2088–2096.
Wang, Z., Bovik, A. C., Sheikh, H. R., and Simoncelli, E. P.
(2004). Image quality assessment: from error visi-
bility to structural similarity. IEEE transactions on
image processing, 13(4):600–612.
Xian, W., Huang, J.-B., Kopf, J., and Kim, C. (2021).
Space-time neural irradiance fields for free-viewpoint
video. In Proceedings of the IEEE/CVF conference on
computer vision and pattern recognition, pages 9421–
9431.
Zhang, R., Isola, P., Efros, A. A., Shechtman, E., and Wang,
O. (2018). The unreasonable effectiveness of deep
features as a perceptual metric. In Proceedings of
the IEEE conference on computer vision and pattern
recognition, pages 586–595.
VISAPP 2025 - 20th International Conference on Computer Vision Theory and Applications
706