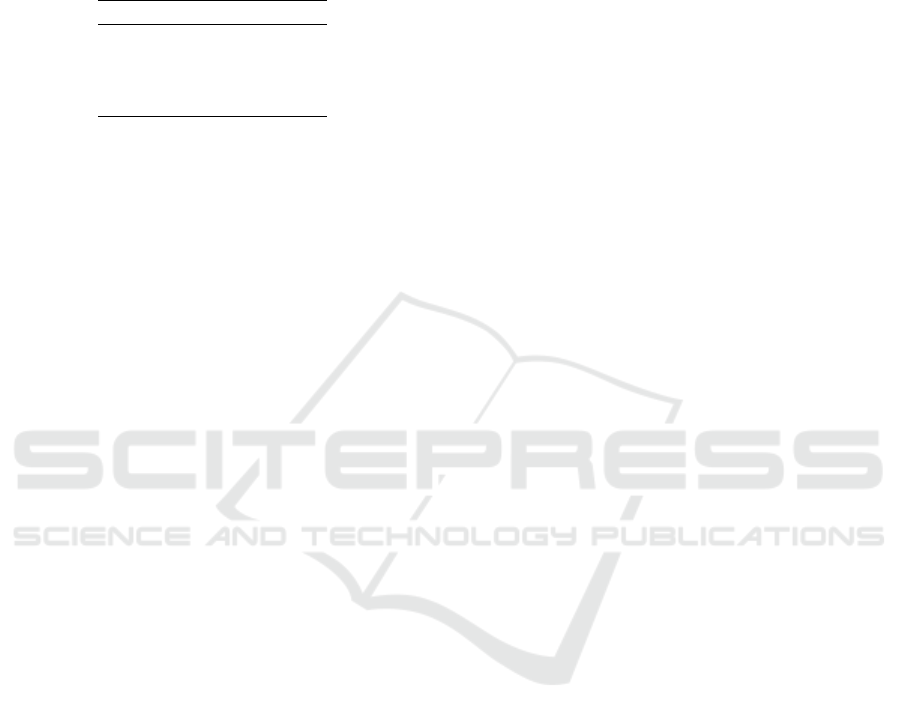
for reliable classification outcomes. However, con-
sidering the substantial sample size of 714,000 exam-
ples, there exists still work for improvement in overall
performance.
Table 3: Performance metrics with 95% confidence inter-
vals for the LOSO-CV evaluation.
Metric Value (%)
Accuracy 79.65 ± 0.09
Precision 80.63 ± 0.09
Recall 79.65 ± 0.09
F1 Score 79.33 ± 0.09
The confusion matrix related to the results is
shown in Figure 5 and highlights some of the best and
worst classified hand poses.
Considering that there are 42,000 examples per
class, One hand pose is the best classified, achieving
39,719 correct predictions with minimal misclassifi-
cation. Spiderman also performs well, with 36,827
correctly identified instances, although it is occasion-
ally confused with Stop (2,886 times). This confu-
sion likely arises from their similar configurations,
both involving extended fingers, making them harder
to distinguish when occluded or viewed from certain
angles. Open Palm is another well-recognized pose,
with 36,685 correct classifications, though some in-
stances are misclassified as Tiger (3,359 times), re-
flecting the slight overlap in appearance when fingers
are not fully straightened or curled.
On the other hand, some poses show poor per-
formance, with quite misclassifications. OK is one
of the worst classified, with 28,770 correct predic-
tions. It is frequently confused with OpenPalm (3,735
times), likely because both involve the extension of
several fingers, and the circular thumb-index gesture
in OK can sometimes appear flattened or ambiguous
from certain angles. Rock also struggles, achieving
only 28,910 correct classifications. It is often con-
fused with Tiger (6,574 times) and Spiderman (2,279
times), as these poses share similar elements, such as
the partial extension of specific fingers, which can be
difficult to distinguish under certain viewpoints.
These results indicate that subtle differences in
finger arrangements and slight variations in curva-
ture contribute to misclassifications. For example, the
similarity between Rock and Spiderman, with their
partially extended fingers, highlights the difficulty of
accurately distinguishing between such poses. Like-
wise, OpenPalm being misclassified as Tiger suggests
that some pose may lack enough distinct visual cues
when viewed from certain perspectives.
These findings suggest future research directions,
including the need to explore the impact of camera
perspective. Identifying which camera—horizontal or
vertical—provides the clearest view of each instance
may improve recognition by leveraging the most in-
formative viewpoint.
5 CONCLUSIONS
In order to establish a baseline for future research,
we evaluated our proposed method on the Multi-view
Leap2 Hand Pose Dataset using a LOSO-CV strategy
in order to provide an accurate system capable to gen-
eralize across different individuals. To the best of our
knowledge, this is the first result achieved using this
dataset. The proposed architecture used ViT to extract
features from images, demonstrating the advantages
of a multimodal approach that combines image data
with hand landmark information. This integration of-
fers a robust performance, even when in some cases
hand pose may be occluded. The system offers a F1
Score of 79.33 ± 0.09 %, which indicates strong clas-
sification performance across the dataset. In particu-
lar, the confusion matrix reveals specific poses that
are frequently misclassified, such as Tiger and Open
Palm, suggesting a need for enhanced strategies o dis-
tinguish between similar poses.
Future work could explore alternative partitioning
strategies for the ML2HP dataset, such as separating
training and testing based on distinct camera orien-
tations or hand dominance. Additionally, detecting
which camera (horizontal or vertical) the hand is pri-
marily oriented towards for each instance and evaluat-
ing performance using data from only that viewpoint
would provide insights into how much information
can be effectively extracted from a single perspec-
tive. In addition, it could be possible to investigate
the impact of using only one modality—either the
image data or the hand landmarks—rather than both.
This would help determine whether the visual features
alone or the landmark information is enough for ac-
curate hand pose recognition in certain scenarios. Ex-
ploring these configurations would provide a clearer
understanding of the individual contributions of each
modality and help develop more efficient models that
optimize either visual or landmark-based recognition.
Furthermore, applying the proposed model to new
datasets will provide deeper insights into its function-
ality and improve the understanding of its practical
performance.
ACKNOWLEDGEMENTS
Sergio Esteban-Romero research was supported
by the Spanish Ministry of Education (FPI grant
Towards Multi-View Hand Pose Recognition Using a Fusion of Image Embeddings and Leap 2 Landmarks
923