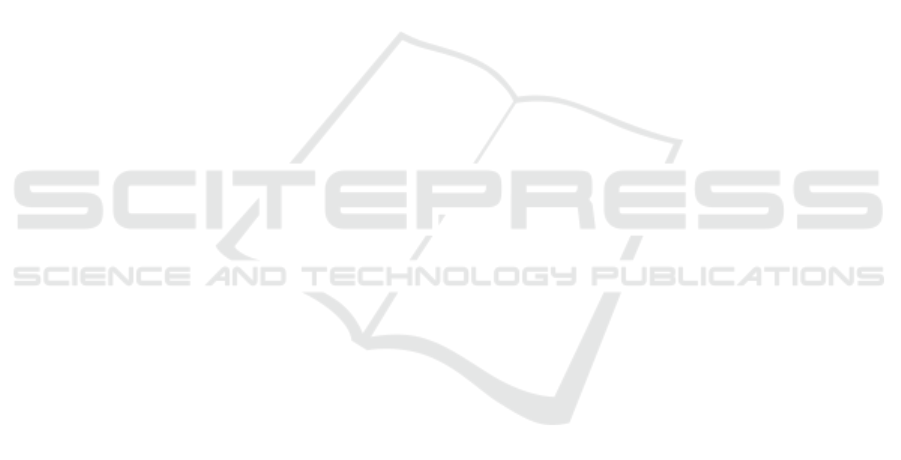
4 Health: Digital Driven Diagnostics, prognostics
and therapeutics for sustainable Health care, Pro-
getto MolisCTe, Ministero delle Imprese e del Made
in Italy, Italy, CUP: D33B22000060001, FORE-
SEEN: FORmal mEthodS for attack dEtEction in au-
tonomous driviNg systems CUP N.P2022WYAEW,
ALOHA: a framework for monitoring the physical
and psychological health status of the Worker through
Object detection and federated machine learning, Call
for Collaborative Research BRiC -2024, INAIL, and
by Fondazione Intesa SanPaolo Onlus in the “Doctor-
ates in Humanities Disciplines” for the “Artificial In-
telligence for the Analysis of Archaeological Finds”
topic.
REFERENCES
Adebiyi, M. O., Olaniyan, D., Adebiyi, A. A., Olaniyan,
J., Amrevuawho, O. F., et al. (2024). Random
forest-based approach for integrating blood profile in
metastatic breast cancer classification. In 2024 In-
ternational Conference on Science, Engineering and
Business for Driving Sustainable Development Goals
(SEB4SDG), pages 1–6. IEEE.
Ahmed, F., Abdel-Salam, R., Hamnett, L., Adewunmi,
M., and Ayano, T. (2023). Improved breast cancer
diagnosis through transfer learning on hematoxylin
and eosin stained histology images. arXiv preprint
arXiv:2309.08745.
Brancati, N., Anniciello, A. M., Pati, P., Riccio, D., Scog-
namiglio, G., Jaume, G., De Pietro, G., Di Bonito, M.,
Foncubierta, A., Botti, G., et al. (2022). Bracs: A
dataset for breast carcinoma subtyping in h&e histol-
ogy images. Database, 2022:baac093.
Brunese, L., Brunese, M. C., Carbone, M., Ciccone, V.,
Mercaldo, F., and Santone, A. (2022a). Automatic
pi-rads assignment by means of formal methods. La
radiologia medica, pages 1–7.
Brunese, L., Mercaldo, F., Reginelli, A., and Santone, A.
(2022b). A neural network-based method for respira-
tory sound analysis and lung disease detection. Ap-
plied Sciences, 12(8):3877.
Di Giammarco, M., Dukic, B., Martinelli, F., Cesarelli, M.,
Ravelli, F., Santone, A., and Mercaldo, F. (2024). Re-
liable leukemia diagnosis and localization through ex-
plainable deep learning. In 2024 Fifth International
Conference on Intelligent Data Science Technologies
and Applications (IDSTA), pages 68–75. IEEE.
Di Giammarco, M., Mercaldo, F., Zhou, X., Huang, P., San-
tone, A., Cesarelli, M., and Martinelli, F. (2023). A
robust and explainable deep learning method for cer-
vical cancer screening. In International Conference
on Applied Intelligence and Informatics, pages 111–
125. Springer.
Gonz
´
alez-Castro, L., Ch
´
avez, M., Duflot, P., Bleret, V.,
Martin, A. G., Zobel, M., Nateqi, J., Lin, S., Pazos-
Arias, J. J., Del Fiol, G., et al. (2023). Machine
learning algorithms to predict breast cancer recurrence
using structured and unstructured sources from elec-
tronic health records. Cancers, 15(10):2741.
He, H., Yang, H., Mercaldo, F., Santone, A., and Huang, P.
(2024). Isolation forest-voting fusion-multioutput: A
stroke risk classification method based on the multidi-
mensional output of abnormal sample detection. Com-
puter Methods and Programs in Biomedicine, page
108255.
Huang, P., Li, C., He, P., Xiao, H., Ping, Y., Feng, P., Tian,
S., Chen, H., Mercaldo, F., Santone, A., et al. (2024).
Mamlformer: Priori-experience guiding transformer
network via manifold adversarial multi-modal learn-
ing for laryngeal histopathological grading. Informa-
tion Fusion, 108:102333.
ICAR, Istituto di Calcolo e Reti ad Alte Prestazioni. Bracs:
Breast carcinoma subtyping. https://www.bracs.icar.
cnr.it/.
Martinelli, F., Mercaldo, F., and Santone, A. (2022). Wa-
ter meter reading for smart grid monitoring. Sensors,
23(1):75.
Mercaldo, F., Di Giammarco, M., Ravelli, F., Martinelli, F.,
Santone, A., and Cesarelli, M. (2024). Alzheimer’s
disease evaluation through visual explainability by
means of convolutional neural networks. Interna-
tional Journal of Neural Systems, 34(2):2450007–
2450007.
Pan, H. and Xin, L. (2024). Fdts: A feature disentangled
transformer for interpretable squamous cell carcinoma
grading. IEEE/CAA Journal of Automatica Sinica,
12(JAS-2024-1027).
Sukhadia, S. S., Muller, K. E., Workman, A. A., and Na-
garaj, S. H. (2023). Machine learning-based predic-
tion of distant recurrence in invasive breast carcinoma
using clinicopathological data: a cross-institutional
study. Cancers, 15(15):3960.
Zhou, X., Tang, C., Huang, P., Tian, S., Mercaldo, F., and
Santone, A. (2023). Asi-dbnet: an adaptive sparse
interactive resnet-vision transformer dual-branch net-
work for the grading of brain cancer histopathological
images. Interdisciplinary Sciences: Computational
Life Sciences, 15(1):15–31.
A Proposal for Explainable Breast Cancer Detection from Histological Images
685