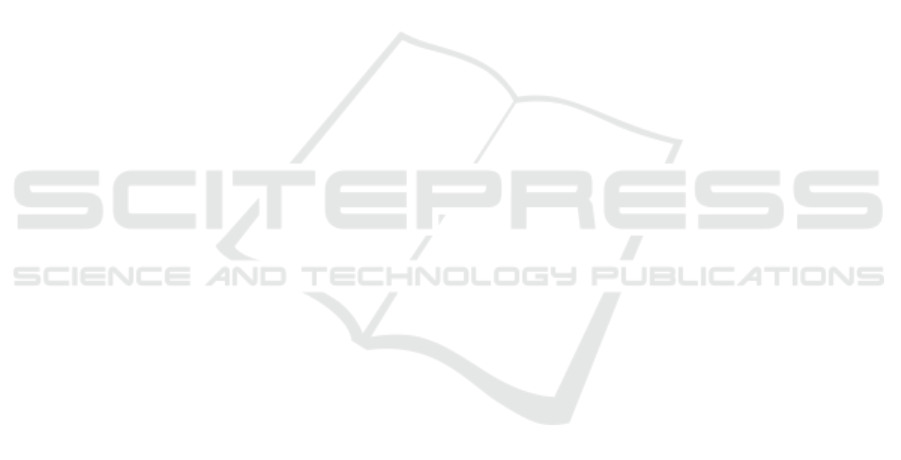
Fan, Z., Liu, J., and Wang, Y. (2021). Motion adaptive pose
estimation from compressed videos. pages 11699–
11708.
Fujita, T. and Kawanishi, Y. (2024). Recurrent graph convo-
lutional network for sequential pose prediction from
3D human skeleton sequence. In Proc. 27th Interna-
tional Conf. on Pattern Recognit., pages 342–358.
Iwata, S., Kawanishi, Y., Deguchi, D., Ide, I., Murase, H.,
and Aizawa, T. (2021). LFIR2Pose: Pose estimation
from an extremely low-resolution fir image sequence.
In Proc. 25th International Conf. on Pattern Recog-
nit., pages 2597–2603.
Jocher, G., Chaurasia, A., and Qiu, J. (2023). Ultralytics
YOLO. (accessed on January 26, 2025).
Kreiss, S., Bertoni, L., and Alahi, A. (2019). PifPaf: Com-
posite fields for human pose estimation. In Proc.
2019 IEEE/CVF Conf. on Comput. Vision and Pattern
Recognit., pages 11969–11978.
Lin, T.-Y., Maire, M., Belongie, S., Bourdev, L., Girshick,
R., Hays, J., Perona, P., Ramanan, D., Zitnick, C. L.,
and Doll
´
ar, P. (2015). Microsoft COCO: Common ob-
jects in context. arXiv:1405.0312.
Lin, T.-Y., Maire, M., Belongie, S., Hays, J., Perona, P., Ra-
manan, D., Doll
´
ar, P., and Zitnick, C. L. (2014). Mi-
crosoft COCO: Common objects in context. In Fleet,
D., Pajdla, T., Schiele, B., and Tuytelaars, T., editors,
Computer Vision – ECCV2014, pages 740–755.
Liu, Z., Chen, H., Feng, R., Wu, S., Ji, S., Yang, B., and
Wang, X. (2021). Deep dual consecutive network
for human pose estimation. In Proc. 2021 IEEE/CVF
Conf. on Comput. Vision and Pattern Recognit., pages
525–534.
Liu, Z., Feng, R., Chen, H., Wu, S., Gao, Y., Gao, Y., and
Wang, X. (2022). Temporal feature alignment and mu-
tual information maximization for video-based human
pose estimation. In Proc. 2022 IEEE/CVF Conf. on
Comput. Vision and Pattern Recognit., pages 11006–
11016.
Loper, M., Mahmood, N., Romero, J., Pons-Moll, G.,
and Black, M. J. (2015). SMPL: A skinned multi-
person linear model. ACM Transactions on Graphics,
34(6):1–16.
Luo, Y., Ren, J., Wang, Z., Sun, W., Pan, J., Liu, J., Pang,
J., and Lin, L. (2018). LSTM pose machines. In Proc.
2018 IEEE/CVF Conf. on Comput. Vision and Pattern
Recognit., pages 5207–5215.
Mizuno, M., Kawanishi, Y., Fujita, T., Deguchi, D., and
Murase, H. (2023). Subjective baggage-weight esti-
mation from gait: Can you estimate how heavy the
person feels? In Proc. 18th International Joint Con-
ference on Computer Vision, Imaging and Computer
Graphics Theory and Applications, volume 5, pages
567–574.
Papandreou, G., Zhu, T., Kanazawa, N., Toshev, A., Tomp-
son, J., Bregler, C., and Murphy, K. (2017a). Towards
accurate multi-person pose estimation in the wild. In
Proc. 2017 IEEE Conf. on Comput. Vision and Pattern
Recognit., pages 3711–3719.
Papandreou, G., Zhu, T., Kanazawa, N., Toshev, A., Tomp-
son, J., Bregler, C., and Murphy, K. (2017b). Towards
accurate multi-person pose estimation in the wild. In
Proc. 2017 IEEE Conf. on Comput. Vision and Pattern
Recognit., pages 3711–3719.
Pfister, T., Charles, J., and Zisserman, A. (2015). Flow-
ing ConvNets for human pose estimation in videos.
In Proc. 15th International Conf. on Comput. Vision,
pages 1913–1921.
Shahroudy, A., Liu, J., Ng, T.-T., and Wang, G. (2016).
NTU RGB+D: A large scale dataset for 3D human
activity analysis. In Proc. 2016 IEEE/CVF Conf. on
Comput. Vision and Pattern Recognit., pages 1010–
1019.
Song, J., Wang, L., Van Gool, L., and Hilliges, O. (2017).
Thin-Slicing Network: A deep structured model for
pose estimation in videos. In Proc. 2017 IEEE/CVF
Conf. on Comput. Vision and Pattern Recognit., pages
5563–5572. IEEE.
Song, L., Yu, G., Yuan, J., and Liu, Z. (2021). Human pose
estimation and its application to action recognition: A
survey. Journal of Visual Communication and Image
Representation, 76:103055.
Srivastav, V., Gangi, A., and Padoy, N. (2019). Human pose
estimation on privacy-preserving low-resolution depth
images. In Proc. 22nd Medical Image Computing and
Computer Assisted Intervention, pages 583–591.
Srivastav, V., Issenhuth, T., Kadkhodamohammadi, A.,
de Mathelin, M., Gangi, A., and Padoy, N. (2018).
MVOR: A multi-view rgb-d operating room dataset
for 2D and 3D human pose estimation. In Proc.
2018 MICCAI Workshop on Large-scale Annotation
of Biomedical data and Expert Label Synthesis.
Temuroglu, O., Kawanishi, Y., Deguchi, D., Hirayama, T.,
Ide, I., Murase, H., Iwasaki, M., and Tsukada, A.
(2020). Occlusion-aware skeleton trajectory repre-
sentation for abnormal behavior detection. In Proc.
26th International Workshop on Frontiers of Com-
puter Vision, volume 1212, pages 108–121, Singa-
pore. Springer Singapore.
Toshev, A. and Szegedy, C. (2014). DeepPose: Human
pose estimation via deep neural networks. In Proc.
2014 IEEE/CVF Conf. on Comput. Vision and Pattern
Recognit., pages 1653–1660.
Wang, C., Zhang, F., Zhu, X., and Ge, S. S. (2022). Low-
resolution human pose estimation. Pattern Recogni-
tion, 126:108579.
Xiao, B., Wu, H., and Wei, Y. (2018). Simple baselines
for human pose estimation and tracking. In Computer
Vision – ECCV2018, volume 11210, pages 472–487.
Xu, X., Chen, H., Moreno-Noguer, F., Jeni, L. A., and De la
Torre, F. (2020). 3D human shape and pose from a sin-
gle low-resolution image with self-supervised learn-
ing. In Computer Vision – ECCV2020, volume 12354,
pages 284–300.
Zhang, R., Zhu, Z., Li, P., Wu, R., Guo, C., Huang, G.,
and Xia, H. (2019). Exploiting offset-guided net-
work for pose estimation and tracking. In Proc.
2019 IEEE/CVF Conf. on Comput. Vision and Pattern
Recognit. Workshops, pages 1–9.
Human Pose Estimation from an Extremely Low-Resolution Image Sequence by Pose Transition Embedding Network
485