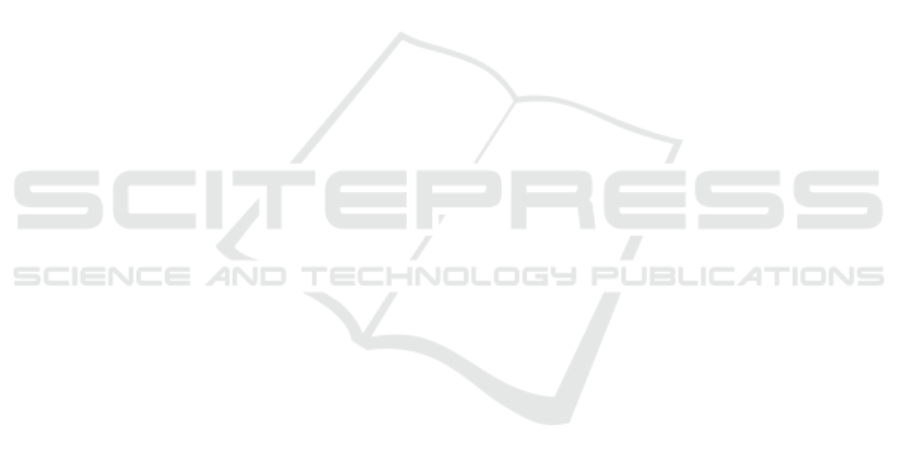
component models. IEEE Transactions on Software
Engineering, 37(5):593–615.
da Rocha, H., Pereira, J., Abrishambaf, R., and Espir-
ito Santo, A. (2022). An interoperable digital twin
with the ieee 1451 standards. Sensors, 22(19).
Delgado, N., Gates, A., and Roach, S. (2004). A taxon-
omy and catalog of runtime software-fault monitoring
tools. IEEE Transactions on Software Engineering,
30(12):859–872.
Despres, C. and Chauvel, D. (1999). Knowledge man-
agement(s). Journal of Knowledge Management,
3(2):110–123.
Dios, M. and Framinan, J. M. (2016). A review and classi-
fication of computer-based manufacturing scheduling
tools. Computers & Industrial Engineering, 99:229–
249.
Errandonea, I., Beltr
´
an, S., and Arrizabalaga, S. (2020).
Digital twin for maintenance: A literature review.
Computers in Industry, 123:103316.
Fahim, M., Sharma, V., Cao, T. V., Canberk, B., and Duong,
T. Q. (2022). Machine learning-based digital twin for
predictive modeling in wind turbines. IEEE Access,
10:14184–14194.
Firth, R., Mosley, V., Pethia, R. D., Gold, L. R., and Wood,
W. (2018). A Guide to the Classification and Assess-
ment of Software Engineering Tools.
Fuller, A., Fan, Z., Day, C., and Barlow, C. (2020). Digi-
tal twin: Enabling technologies, challenges and open
research. IEEE Access, 8:108952–108971.
Gabale, V., Raman, B., Dutta, P., and Kalyanraman, S.
(2013). A classification framework for scheduling al-
gorithms in wireless mesh networks. IEEE Communi-
cations Surveys & Tutorials, 15(1):199–222.
Ghaboura, S., Ferdousi, R., Laamarti, F., Yang, C., and Sad-
dik, A. E. (2023). Digital twin for railway: A compre-
hensive survey. IEEE Access, 11:120237–120257.
Gil, S., Mikkelsen, P. H., Gomes, C., and Larsen, P. G.
(2024). Survey on open-source digital twin frame-
works–a case study approach. Software: Practice and
Experience, 54(6):929–960.
Graham, D. R. (1991). Software testing tools: A new clas-
sification scheme. Software Testing, Verification and
Reliability, 1(3):17–34.
Hodavand, F., Ramaji, I. J., and Sadeghi, N. (2023). Dig-
ital twin for fault detection and diagnosis of building
operations: A systematic review. Buildings, 13(6).
Hu, W., Zhang, T., Deng, X., Liu, Z., and Tan, J. (2021).
Digital twin: a state-of-the-art review of its enabling
technologies, applications and challenges. Journal
of Intelligent Manufacturing and Special Equipment,
2(1):1–34.
Jones, D., Snider, C., Nassehi, A., Yon, J., and Hicks, B.
(2020). Characterising the digital twin: A systematic
literature review. CIRP Journal of Manufacturing Sci-
ence and Technology, 29:36–52.
Kahani, N., Bagherzadeh, M., Cordy, J. R., Dingel, J., and
Varr
´
o, D. (2019). Survey and classification of model
transformation tools. Software & Systems Modeling,
18(4):2361–2397.
Kazman, R., Echeverr
´
ıa, S., and Ivers, J. (2022). Extensi-
bility. Technical Report CMU/SEI-2022-TR-002. Ac-
cessed: 2024-Oct-30.
Kramer, J. (2007). Is abstraction the key to computing?
Commun. ACM, 50(4):36–42.
Lehnert, S. (2011). A taxonomy for software change im-
pact analysis. In Proceedings of the 12th International
Workshop on Principles of Software Evolution and the
7th Annual ERCIM Workshop on Software Evolution,
IWPSE-EVOL ’11, page 41–50, New York, NY, USA.
Association for Computing Machinery.
Lin, Y., Chen, L., Ali, A., Nugent, C., Ian, C., Li, R., Gao,
D., Wang, H., Wang, Y., and Ning, H. (2022). Human
digital twin: A survey.
Liu, M., Fang, S., Dong, H., and Xu, C. (2021). Review of
digital twin about concepts, technologies, and indus-
trial applications. Journal of Manufacturing Systems,
58:346–361. Digital Twin towards Smart Manufactur-
ing and Industry 4.0.
Liu, Y. K., Ong, S. K., and Nee, A. Y. C. (2022). State-of-
the-art survey on digital twin implementations. Ad-
vances in Manufacturing, 10(1):1–23.
Lo, C., Chen, C., and Zhong, R. Y. (2021). A review of digi-
tal twin in product design and development. Advanced
Engineering Informatics, 48:101297.
Lu, Q., Parlikad, A. K., Woodall, P., Ranasinghe, G. D.,
Xie, X., Liang, Z., Konstantinou, E., Heaton, J., and
Schooling, J. (2020). Developing a digital twin at
building and city levels: Case study of west cam-
bridge campus. Journal of Management in Engineer-
ing, 36(3):05020004.
Lv, Z. and Xie, S. (2022). Artificial intelligence in the dig-
ital twins: State of the art, challenges, and future re-
search topics [version 2; peer review: 2 approved].
Digital Twin, 1(12).
Medvidovic, N. and Taylor, R. N. (2000). A classification
and comparison framework for software architecture
description languages. IEEE Trans. Software Eng.,
26(1):70–93.
Mehta, N. R., Medvidovic, N., and Phadke, S. (2000). To-
wards a taxonomy of software connectors. In Pro-
ceedings of the 22nd International Conference on
Software Engineering, ICSE ’00, page 178–187, New
York, NY, USA. Association for Computing Machin-
ery.
Mendi, A. F. (2022). A digital twin case study on automo-
tive production line. Sensors, 22(18).
Mens, T., Buckley, J., Zenger, M., and Rashid, A. (2003).
Towards a taxonomy of software evolution.
Metzger, A. and Pohl, K. (2014). Software product line en-
gineering and variability management: achievements
and challenges. In Herbsleb, J. D. and Dwyer, M. B.,
editors, Proceedings of the on Future of Software En-
gineering, FOSE 2014, Hyderabad, India, May 31 -
June 7, 2014, pages 70–84. ACM.
Michael, J., Pfeiffer, J., Rumpe, B., and Wortmann,
A. (2022). Integration challenges for digital twin
systems-of-systems. In Proceedings of the 10th
IEEE/ACM International Workshop on Software Engi-
neering for Systems-of-Systems and Software Ecosys-
Towards a Classification Framework for the Digital Twin Tools: A Taxonomy
271