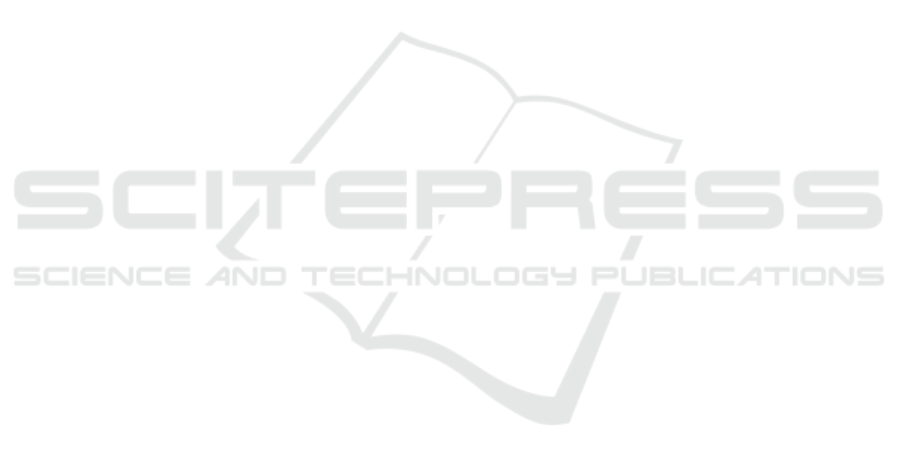
6.1 Threats to Validity
While the results were promising, there are certain
threats to the validity of our approach. First, the sam-
ple size was limited to 1714 student programs from
a single institution, which may not fully represent
the diversity of approaches found in broader educa-
tional contexts. Additionally, the focus on sorting al-
gorithms may have limited the generalizability of the
tool’s applicability to other algorithmic topics.
Moreover, the qualitative evaluation was con-
ducted with a relatively small group of instructors,
which may not fully reflect the perspectives of a wider
range of educators. Future studies should consider ex-
panding the qualitative evaluation to a broader sample
of instructors and different educational settings.
6.2 Future Work
As future work, we plan to expand the functionality
of the Python Design Wizard by creating a catalog of
pre-defined design tests that can be shared with the
community of educators. These tests could be tai-
lored to various common topics in introductory pro-
gramming courses, such as recursion, dynamic pro-
gramming, and data structures.
Furthermore, it would be interesting to investigate
the integration of this tool into other programming
paradigms and languages, as well as expanding its ca-
pabilities to more complex scenarios that go beyond
sorting algorithms. We also aim to explore the possi-
bility of developing more specific tests that could han-
dle different levels of complexity, providing a more
granular evaluation of algorithm design.
An interesting observation from our study is that
we evaluated the tests themselves rather than the tool
directly, which is why we included unit tests in our
validation process. This approach allowed us to mea-
sure how well the design tests perform in identifying
algorithmic patterns and violations, rather than focus-
ing solely on the technical capabilities of the tool.
REFERENCES
Ala-Mutka, K. M. (2005). A survey of automated assess-
ment approaches for programming assignments. Com-
puter science education, 15(2):83–102.
Araujo, E., Serey, D., and Figueiredo, J. (2016). Qualita-
tive aspects of students’ programs: Can we make them
measurable? In 2016 IEEE Frontiers in Education
Conference (FIE), pages 1–8. IEEE.
Beazley, D. (2012). Python Essential Reference. Addison-
Wesley.
Brunet, J., Guerrero, D., and Figueiredo, J. (2009). De-
sign tests: An approach to programmatically check
your code against design rules. In 2009 31st Inter-
national Conference on Software Engineering - Com-
panion Volume, pages 255–258.
Ericsson, K. A. and Simon, H. A. (1993a). Protocol Anal-
ysis: Verbal Reports as Data. MIT Press, Cambridge,
MA, revised edition edition.
Ericsson, K. A. and Simon, H. A. (1993b). Protocol Anal-
ysis: Verbal Reports as Data. MIT Press, Cambridge,
MA, revised edition edition.
Foundation, P. S. (2023). Python 3 Documentation. Avail-
able at https://docs.python.org/3/.
Janzen, S. and others (2013). Automated grading systems in
competitive programming contests. In Proceedings of
the 8th International Symposium on Educational Soft-
ware Development.
Juiz, A. and others (2014). Online judge and its applica-
tion to introductory programming courses. In ACM
SIGCSE Bulletin.
JUnit Team (2023). JUnit 5: The Next Generation of JUnit.
Accessed: 2024-11-11.
Keuning, H., Jeuring, J., and Heeren, B. (2018). A system-
atic literature review of automated feedback genera-
tion for programming exercises. ACM Transactions
on Computing Education (TOCE), 19(1):1–43.
Leite, L. (2015). A proposal for improving programming
education with frequent problem solving exercises. In
Brazilian Symposium on Computer Education.
Paiva, J. C., Leal, J. P., and Figueira,
´
A. (2022). Automated
assessment in computer science education: A state-
of-the-art review. ACM Transactions on Computing
Education (TOCE), 22(3):1–40.
Papastergiou, M. (2009). Digital game-based learning in
computer programming: Impact on educational effec-
tiveness and student motivation. Computers & Educa-
tion.
Singh, J., Gupta, P., and Sharma, M. (2013). Online judge
system for learning and practicing programming. In
International Journal of Computer Applications.
Truong, N., Roe, P., and Bancroft, P. (2004). Static analysis
of students’ java programs. In Proceedings of the Sixth
Australasian Conference on Computing Education-
Volume 30, pages 317–325. Citeseer.
van Rossum, G. (2009). The Python Language Reference
Manual. Network Theory Ltd.
Wasik, S., Antczak, M., Badura, J., Laskowski, A., and
Sternal, T. (2018). A survey on online judge sys-
tems and their applications. ACM Computing Surveys
(CSUR), 51(1):1–34.
Watson, C. and Li, F. W. (2014). Failure rates in intro-
ductory programming revisited. In Proceedings of the
2014 conference on Innovation & technology in com-
puter science education, pages 39–44.
Beyond Functionality: Automating Algorithm Design Evaluation in Introductory Programming Courses
525