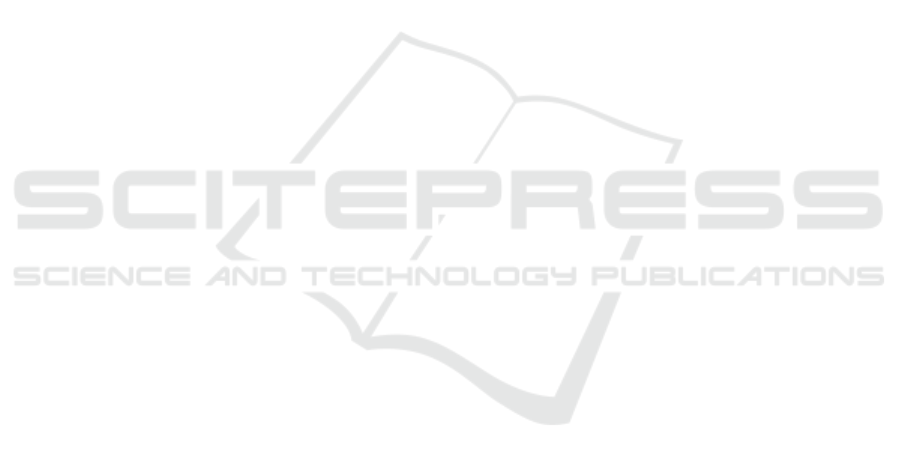
details, and improved geometry, especially in chal-
lenging areas like the sky and the ground.
REFERENCES
Barron, J. T., Mildenhall, B., Tancik, M., Hedman, P.,
Martin-Brualla, R., and Srinivasan, P. P. (2021).
Mip-NeRF: A multiscale representation for anti-
aliasing neural radiance fields. In Proceedings of the
IEEE/CVF International Conference on Computer Vi-
sion (ICCV), pages 5855–5864.
Barron, J. T., Mildenhall, B., Verbin, D., Srinivasan, P. P.,
and Hedman, P. (2022). Mip-NeRF 360: Unbounded
anti-aliased neural radiance fields. In Proceedings of
the IEEE/CVF Conference on Computer Vision and
Pattern Recognition (CVPR), pages 5470–5479.
Barron, J. T., Mildenhall, B., Verbin, D., Srinivasan, P. P.,
and Hedman, P. (2023). Zip-NeRF: Anti-aliased grid-
based neural radiance fields. In Proceedings of the
IEEE/CVF International Conference on Computer Vi-
sion (ICCV), pages 19697–19705.
He, K., Zhang, X., Ren, S., and Sun, J. (2016). Deep resid-
ual learning for image recognition. In Proceedings of
the IEEE Conference on Computer Vision and Pattern
Recognition (CVPR), pages 770–778.
Li, Y., Liu, Z., Monno, Y., and Okutomi, M. (2025).
TDM: Temporally-consistent diffusion model for all-
in-one real-world video restoration. In Proceedings
of International Conference on Multimedia Modeling
(MMM), pages 155–169.
Liu, S., Zeng, Z., Ren, T., Li, F., Zhang, H., Yang, J.,
Li, C., Yang, J., Su, H., Zhu, J., et al. (2023).
Grounding DINO: Marrying DINO with grounded
pre-training for open-set object detection. arXiv
preprint 2303.05499.
Mildenhall, B., Srinivasan, P. P., Tancik, M., Barron, J. T.,
Ramamoorthi, R., and Ng, R. (2020). NeRF: Repre-
senting scenes as neural radiance fields for view syn-
thesis. In Proceedings of European Conference on
Computer Vision (ECCV), pages 405–421.
M
¨
uller, T., Evans, A., Schied, C., and Keller, A. (2022).
Instant neural graphics primitives with a multiresolu-
tion hash encoding. ACM Transactions on Graphics
(TOG), 41(4):1–15.
Rematas, K., Liu, A., Srinivasan, P. P., Barron, J. T.,
Tagliasacchi, A., Funkhouser, T., and Ferrari, V.
(2022). Urban radiance fields. In Proceedings of the
IEEE/CVF Conference on Computer Vision and Pat-
tern Recognition (CVPR), pages 12932–12942.
Ren, T., Liu, S., Zeng, A., Lin, J., Li, K., Cao, H., Chen, J.,
Huang, X., Chen, Y., Yan, F., Zeng, Z., Zhang, H., Li,
F., Yang, J., Li, H., Jiang, Q., and Zhang, L. (2024).
Grounded SAM: Assembling open-world models for
diverse visual tasks. In arXiv preprint 2401.14159.
Rombach, R., Blattmann, A., Lorenz, D., Esser, P., and
Ommer, B. (2022). High-resolution image synthesis
with latent diffusion models. In Proceedings of the
IEEE/CVF Conference on Computer Vision and Pat-
tern Recognition (CVPR), pages 10684–10695.
Sch
¨
onberger, J. L. and Frahm, J.-M. (2016). Structure-
from-motion revisited. In Proceedings of IEEE Con-
ference on Computer Vision and Pattern Recognition
(CVPR), pages 4104–4113.
Sun, C., Sun, M., and Chen, H.-T. (2022). Direct voxel
grid optimization: Super-fast convergence for radi-
ance fields reconstruction. In Proceedings of the
IEEE/CVF Conference on Computer Vision and Pat-
tern Recognition (CVPR), pages 5459–5469.
Tancik, M., Casser, V., Yan, X., Pradhan, S., Mildenhall,
B., Srinivasan, P. P., Barron, J. T., and Kretzschmar,
H. (2022). Block-NeRF: Scalable large scene neural
view synthesis. In Proceedings of the IEEE/CVF Con-
ference on Computer Vision and Pattern Recognition
(CVPR), pages 8248–8258.
Turki, H., Zhang, J. Y., Ferroni, F., and Ramanan, D. (2023).
SUDS: Scalable urban dynamic scenes. In Proceed-
ings of the IEEE/CVF Conference on Computer Vi-
sion and Pattern Recognition (CVPR), pages 12375–
12385.
Wang, F., Louys, A., Piasco, N., Bennehar, M., Rold
˜
aao,
L., and Tsishkou, D. (2024). PlaNeRF: SVD unsu-
pervised 3D plane regularization for NeRF large-scale
urban scene reconstruction. In Proceedings of Inter-
national Conference on 3D Vision (3DV), pages 1291–
1300.
Zhang, K., Riegler, G., Snavely, N., and Koltun, V. (2020).
NeRF++: Analyzing and improving neural radiance
fields. arXiv preprint 2010.07492.
Zhang, L., Rao, A., and Agrawala, M. (2023). Adding con-
ditional control to text-to-image diffusion models. In
Proceedings of the IEEE/CVF International Confer-
ence on Computer Vision (ICCV), pages 3836–3847.
Segmentation-Guided Neural Radiance Fields for Novel Street View Synthesis
597