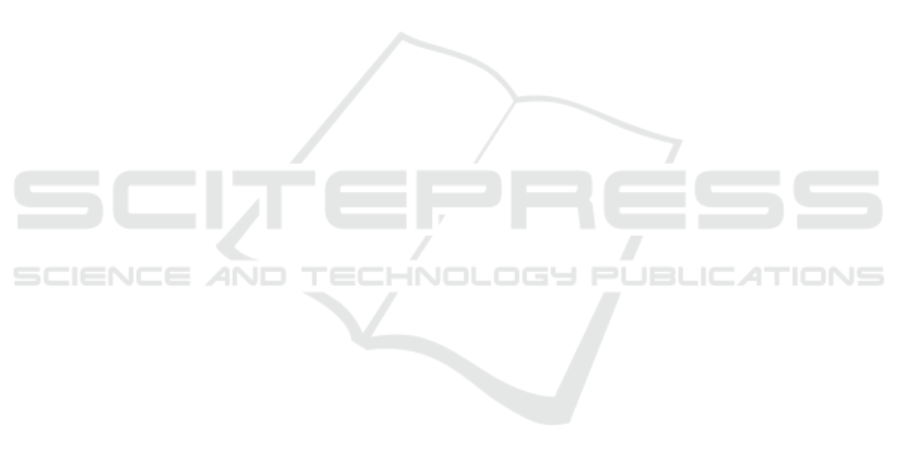
6 CONCLUSIONS
We compared important open IaC technologies based
on a structured catalogue of criteria to capture the
state-of-the-art. Then, we identified challenges for
IaC that would direct future research in the area. We
aligned the challenges with an IaC-specific DevOps
framework and mapped challenges to defect in order
to indicate defect handling strategies within the De-
vOps phases. We have build on a variety of sources
here to incorporate empirical research and thematic
issues on the topic, but have extended and combined
these into a coherent review of technologies and chal-
lenges. The review revealed a variety of different so-
lution, which due to their heterogeneity create a di-
verse technology market with many challenges result-
ing from this. Variety allows for innovative directions
to be taken, but also reflects that currently no consen-
sus on successful directions and that, in many tech-
nology aspects, not enough best practice knowledge
exist, adding to the list of open challenges.
What emerges is the need for more automation. In
particular the management of defects requires more
intelligent approaches for all stages. Here, solutions
from general software engineering with is defect pre-
vention, detection and remediation techniques using
patterns, smell and drift identification can be bor-
rowed to transfer ideas from general code to IaC as
a specific code format. This requires the role of AI
(Pahl, 2023; Tatineni and Chakilam, 2024) to be ex-
plored for the challenges in general and how in par-
ticular the defects across the DevOps stages can be
addressed.
REFERENCES
Alonso, J., Piliszek, R., and Cankar, M. (2023). Embrac-
ing iac through the devsecops philosophy: Concepts,
challenges, and a reference framework. IEEE Soft-
ware, 40(1):56–62.
Aviv, I., Gafni, R., Sherman, S., Aviv, B., Sterkin, A., and
Bega, E. (2023). Infrastructure from code: The next
generation of cloud lifecycle automation. IEEE Softw.,
40(1):42–49.
Borovits, N., Kumara, I., Nucci, D. D., Krishnan, P., Palma,
S. D., Palomba, F., Tamburri, D. A., and van den
Heuvel, W. (2022). Findici: Using machine learn-
ing to detect linguistic inconsistencies between code
and natural language descriptions in infrastructure-as-
code. Empir. Softw. Eng., 27(7):178.
Cerny, T., Abdelfattah, A. S., Maruf, A. A., Janes, A., and
Taibi, D. (2023). Catalog and detection techniques of
microservice anti-patterns and bad smells: A tertiary
study. Journal of Systems and Software, 206:111829.
Chardet, M., Coullon, H., and Robillard, S. (2021). Toward
safe and efficient reconfiguration with concerto. Sci-
ence of Computer Programming, 203:102582.
Karanjai, R., Kasichainula, K., Xu, L., Diallo, N., Chen, L.,
and Shi, W. (2023). Diac: Re-imagining decentralized
infrastructure as code using blockchain. IEEE Trans-
actions on Network and Service Management.
Kumara, I., Garriga, M., Romeu, A. U., Di Nucci, D.,
Palomba, F., Tamburri, D. A., and van den Heuvel, W.-
J. (2021). The do’s and don’ts of infrastructure code:
A systematic gray literature review. Information and
Software Technology, 137:106593.
Opdebeeck, R., Zerouali, A., and De Roover, C. (2023).
Behaviour-aware security smell detection for infras-
tructure as code. In BE-NL Software Evolution.
Ozkaya, I. (2023). Infrastructure as code and software
architecture conformance checking. IEEE Softw.,
40(1):4–8.
Pahl, C. (2023). Research challenges for machine learning-
constructed software. Service Oriented Computing
and Applications, 17(1):1–4.
Pahl, C., Fronza, I., El Ioini, N., and Barzegar, H. R. (2019).
A review of architectural principles and patterns for
distributed mobile information systems. In Intl Conf
on Web Information Systems and Technologies.
Pahl, C., Jamshidi, P., and Zimmermann, O. (2020). Mi-
croservices and containers. Software Engineering
Conference. Gesellschaft f
¨
ur Informatik eV.
Palma, S. D., Nucci, D. D., Palomba, F., and Tamburri,
D. A. (2020). Toward a catalog of software qual-
ity metrics for infrastructure code. J. Syst. Softw.,
170:110726.
Palma, S. D., Nucci, D. D., Palomba, F., and Tamburri,
D. A. (2022). Within-project defect prediction of
infrastructure-as-code using product and process met-
rics. IEEE Trans. Software Eng., 48(6):2086–2104.
Quattrocchi, G. and Tamburri, D. A. (2022). Predic-
tive maintenance of infrastructure code using ”fluid”
datasets: An exploratory study on ansible defect
proneness. J. Softw. Evol. Process., 34(11).
Quattrocchi, G. and Tamburri, D. A. (2023). Infrastructure
as code. IEEE Softw., 40(1):37–40.
Rahman, A., Farhana, E., Parnin, C., and Williams, L.
(2020). Gang of eight: A defect taxonomy for infras-
tructure as code scripts. In ICSE.
Rahman, A., Mahdavi-Hezaveh, R., and Williams, L.
(2019). A systematic mapping study of infrastructure
as code research. Information and Software Technol-
ogy, 108:65–77.
Sokolowski, D., Weisenburger, P., and Salvaneschi, G.
(2023). Decentralizing infrastructure as code. IEEE
Softw., 40(1):50–55.
Staron, M., Abrah
˜
ao, S., Penzenstadler, B., and Hochstein,
L. (2023). Recent research into infrastructure as code.
IEEE Softw., 40(1):86–88.
Tatineni, S. and Chakilam, N. V. (2024). Integrating ar-
tificial intelligence with devops for intelligent infras-
tructure management: Optimizing resource allocation
and performance in cloud-native applications. Jrnl of
Bioinformatics and AI, 4(1):109–142.
CLOSER 2025 - 15th International Conference on Cloud Computing and Services Science
158