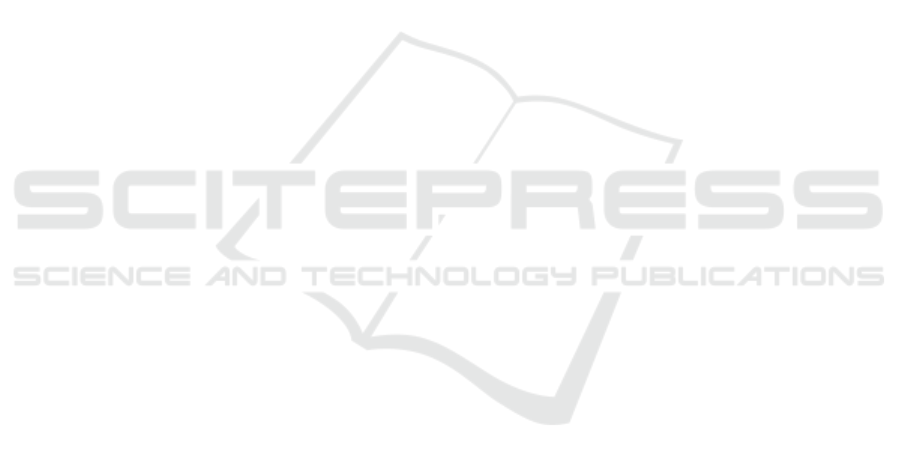
Leveraging these scientifically validated thresholds,
we developed a robust audio classifier using a Feed-
Forward Neural Network (FFNN) which efficiently
categorizes music across all languages and genres into
”Stressed,” ”Not-Stressed,” and ”Borderline” class la-
bels by analyzing key features, which are linked to
positive emotional valence and arousal. This uni-
versal audio feature-based approach surpasses genre-
specific limitations, offering accurate and culturally
inclusive stress classification across diverse languages
and music styles. For future work, we aim to explore
and incorporate advanced techniques that enhance the
interpretability of our model.
ACKNOWLEDGEMENTS
We extend our deepest gratitude to Dr Keya Das, De-
partment of Psychiatry at PES University Institute
of Medical Sciences and Research for her invaluable
contributions to this study. Her expertise, time, and
insightful feedback were crucial in validating our ap-
proach and ensuring the success of this research.
REFERENCES
Adiasto, K., B. D. G. J. v. H.-M. L. M.-R. K. . G. S. A. E.
(2022). Music listening and stress recovery in healthy
individuals: A systematic review with meta-analysis
of experimental studies. PLoS One, 17(6):e0270031.
Adiasto, K., van Hooff, M. L. M., Beckers, D. G. J., and
Geurts, S. A. E. (2023a). The sound of stress recovery:
an exploratory study of self-selected music listening
after stress. BMC Psychology, 11(1):40.
Adiasto, K., van Hooff, M. L. M., Beckers, D. G. J., and
Geurts, S. A. E. (2023b). The sound of stress recovery:
an exploratory study of self-selected music listening
after stress. BMC Psychology, 11(1):40.
Ahuja, R. & Banga, A. (2019). Mental stress detection
in university students using machine learning algo-
rithms. Procedia Computer Science, 152:349–353.
Anderson, C. A., Carnagey, N. L., and Eubanks, J. (2003).
Exposure to violent media: The effects of songs
with violent lyrics on aggressive thoughts and feel-
ings. Journal of Personality and Social Psychology,
84(5):960–971.
Duman, D., Neto, P., Mavrolampados, A., Toiviainen, P.,
and Luck, G. (2022). Music we move to: Spotify
audio features and reasons for listening. PLoS One,
17(9):e0275228.
Erbay Dalli,
¨
O., B. C. . Y. Y. (2023). The effectiveness
of music interventions on stress response in intensive
care patients: A systematic review and meta-analysis.
Journal of Clinical Nursing, 32(11-12):2827–2845.
Gu, Y.-Y., Z. L.-L. T. X.-Y. Y. F.-X. L. L.-M. G. Y.-S. L. H.
L. T.-Z. B. G.-Q. . F. Z.-Q. (2023). A spatial transcrip-
tome reference map of macaque brain states. Transla-
tional Psychiatry, 13:220.
Gujar, S. S. & Reha, A. Y. (2023). Exploring relationship
between music and mood through machine learning
technique. In Proceedings of the 5th International
Conference on Information Management & Machine
Intelligence, pages 1–6.
He, H., Bai, Y., and Garcia, E. A. (2008). Adasyn: Adaptive
synthetic sampling approach for imbalanced learning.
In Proceedings of the 2008 International Joint Con-
ference on Artificial Intelligence, pages 1322–1327.
Juslin, P. N. and Sloboda, J. A. (2010). Handbook of Music
and Emotion: Theory, Research, Applications. Oxford
University Press, Oxford, UK.
Lin, S. T., Y. P. L. C. Y.-S. Y. Y.-Y. Y. C.-H. M. F.-. C. C. C.
(2011). Mental health implications of music: Insight
from neuroscientific and clinical studies. Harvard Re-
view of Psychiatry, 19(1):34–46.
Liu, X., Wu, J., and Zhou, Z. (2007). Generative oversam-
pling for mining imbalanced datasets. Proceedings of
the 7th ACM SIGKDD International Conference on
Knowledge Discovery and Data Mining, pages 9–18.
Lundberg, S. M. & Lee, S. I. (2017). A unified approach to
interpreting model predictions. In Advances in Neural
Information Processing Systems, volume 30.
McFerran, K. S., G. S. H. C. . M. K. (2014). Examining the
relationship between self-reported mood management
and music preferences of australian teenagers. Nordic
Journal of Music Therapy, 24(3):187–203.
Saarikallio, S. & Erkkil
¨
a, J. (2007). The role of music in
adolescents’ mood regulation. Psychology of Music,
35(1):88–109.
Stewart, J., Garrido, S., Hense, C., and McFerran, K.
(2019). Music use for mood regulation: Self-
awareness and conscious listening choices in young
people with tendencies to depression. Frontiers in
Psychology, 10:1199.
Sundararajan, M., T. A. . Y. Q. (2017). Axiomatic attribu-
tion for deep networks. In International Conference
on Machine Learning, pages 3319–3328. PMLR.
Vuust, P., Heggli, O. A., Friston, K. J., et al. (2022). Music
in the brain. Nature Reviews Neuroscience, 23:287–
305.
Zhang, Y., Chen, J., Liao, L., and Yao, K. (2018). Ran-
dom noise injection-based adversarial training for ro-
bust speech recognition. In Proceedings of the 2018
IEEE International Conference on Acoustics, Speech
and Signal Processing (ICASSP), pages 3156–3160.
An Empirical Study Using Machine Learning to Analyze the Relationship Between Musical Audio Features and Psychological Stress
1039