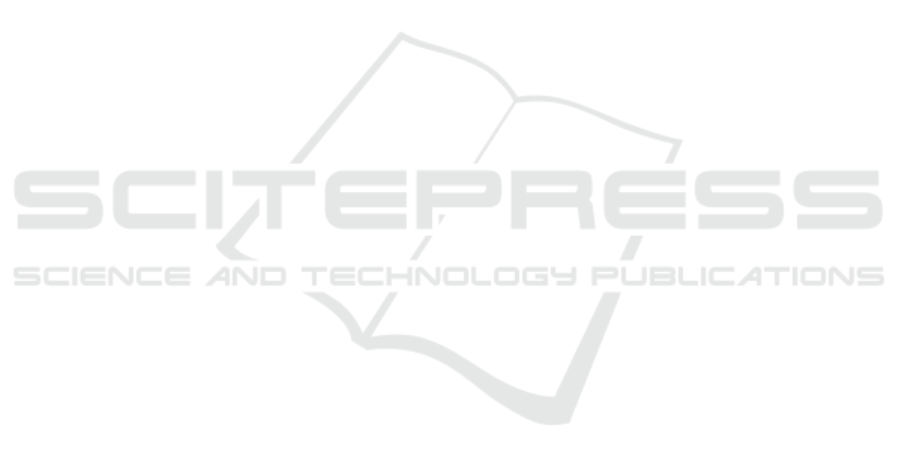
Gillmann, C., Maack, R. G. C., Raith, F., P
´
erez, J. F., and
Scheuermann, G. (2023). A taxonomy of uncertainty
events in visual analytics. IEEE Computer Graphics
and Applications, 43(5):62–71.
Gillmann, C., Saur, D., Wischgoll, T., and Scheuermann,
G. (2021). Uncertainty-aware visualization in medi-
cal imaging - a survey. Computer Graphics Forum,
40(3):665–689.
Gravel, P., Beaudoin, G., and de Guise, J. A. (2004). A
method for modeling noise in medical images. IEEE
Transactions on Medical Imaging, 23:1221–1232.
Howard, M., Luttman, A., and Fowler, M. (2014).
Sampling-based uncertainty quantification in decon-
volution of x-ray radiographs. Journal of Computa-
tional and Applied Mathematics, 270:43–51. Fourth
International Conference on Finite Element Methods
in Engineering and Sciences (FEMTEC 2013).
Klacansky, P. (2017). Open scivis datasets.
https://klacansky.com/open-scivis-datasets/.
Laidlaw, D. H., Trumbore, W. B., and Hughes, J. F. (1986).
Constructive solid geometry for polyhedral objects.
In Proceedings of the 13th Annual Conference on
Computer Graphics and Interactive Techniques, SIG-
GRAPH ’86, page 161–170, New York, NY, USA. As-
sociation for Computing Machinery.
Lorensen, W. E. and Cline, H. E. (1987). Marching cubes:
A high resolution 3d surface construction algorithm.
In Proceedings of the 14th Annual Conference on
Computer Graphics and Interactive Techniques, SIG-
GRAPH ’87, page 163–169, New York, NY, USA. As-
sociation for Computing Machinery.
Lu, C.-T., Chen, M.-Y., Shen, J.-H., Wang, L.-L., and Hsu,
C.-C. (2018). Removal of salt-and-pepper noise for
x-ray bio-images using pixel-variation gain factors.
Computers & Electrical Engineering, 71:862–876.
Madhura, J. J. and Babu, D. R. R. (2017). An effective hy-
brid filter for the removal of gaussian-impulsive noise
in computed tomography images. In 2017 Interna-
tional Conference on Advances in Computing, Com-
munications and Informatics (ICACCI), pages 1815–
1820.
Otsu, N. (1979). A threshold selection method from gray-
level histograms. IEEE Transactions on Systems,
Man, and Cybernetics, 9(1):62–66.
Potter, K., Rosen, P., and Johnson, C. R. (2012). From quan-
tification to visualization: A taxonomy of uncertainty
visualization approaches. In Dienstfrey, A. M. and
Boisvert, R. F., editors, Uncertainty Quantification in
Scientific Computing, pages 226–249, Berlin, Heidel-
berg. Springer Berlin Heidelberg.
Preim, B. and Botha, C. P. (2013). Visual Computing
for Medicine: Theory, Algorithms, and Applications.
Morgan Kaufmann Publishers Inc., San Francisco,
CA, USA, 2 edition.
Raidou, R. G. (2018). Uncertainty visualization: Recent
developments and future challenges in prostate can-
cer radiotherapy planning. In EuroRV3 2018: EuroVis
Workshop on Reproducibility, Verification, and Vali-
dation in Visualization, pages 13–17. The Eurograph-
ics Association.
Reiter, O., Breeuwer, M., Gr
¨
oller, E., and Raidou, R.
(2018). Comparative visual analysis of pelvic organ
segmentations. pages 037–041.
Ristovski, G., Preusser, T., Hahn, H. K., and Linsen, L.
(2014). Uncertainty in medical visualization: Towards
a taxonomy. Computers & Graphics, 39:60–73.
Roy, C. J. and Oberkampf, W. L. (2011). A comprehen-
sive framework for verification, validation, and uncer-
tainty quantification in scientific computing. Com-
puter Methods in Applied Mechanics and Engineer-
ing, 200(25):2131–2144.
Sacha, D., Senaratne, H., Kwon, B. C., Ellis, G., and Keim,
D. A. (2016). The role of uncertainty, awareness, and
trust in visual analytics. IEEE Transactions on Visu-
alization and Computer Graphics, 22(1):240–249.
Schlachter, M., Raidou, R. G., Muren, L. P., Preim, B.,
Putora, P. M., and B
¨
uhler, K. (2019). State-of-the-
art report: Visual computing in radiation therapy plan-
ning. In Computer Graphics Forum, volume 38, pages
753–779. Wiley Online Library.
Tian, X. and Samei, E. (2016). Accurate assessment and
prediction of noise in clinical ct images. Medical
Physics, 43(1):475–482.
van der Maaten, L. (2014). Accelerating t-sne using tree-
based algorithms. Journal of Machine Learning Re-
search, 15(93):3221–3245.
van der Maaten, L. and Hinton, G. (2008). Visualizing data
using t-sne. Journal of Machine Learning Research,
9(86):2579–2605.
Wu, Y., Yuan, G.-X., and Ma, K.-L. (2012). Visualiz-
ing flow of uncertainty through analytical processes.
IEEE Transactions on Visualization and Computer
Graphics, 18:2526–2535.
Yucel-Finn, A., Mckiddie, F., Prescott, S., and Griffiths, R.
(2023). A Brief History of Time: From the Big Bang
to Black Holes. Elsevier, 1 edition.
Zhang, J. (2021). Modern monte carlo methods for efficient
uncertainty quantification and propagation: A survey.
WIREs Computational Statistics, 13(5):e1539.
Zhou, S. K., Greenspan, H., Davatzikos, C., Duncan, J. S.,
Van Ginneken, B., Madabhushi, A., Prince, J. L.,
Rueckert, D., and Summers, R. M. (2021). A re-
view of deep learning in medical imaging: Imaging
traits, technology trends, case studies with progress
highlights, and future promises. Proceedings of the
IEEE, 109(5):820–838.
APPENDIX
The symbols x =
h
x
y
z
i
and x
2
= [
x
y
] denote the image
position in all artifact models described below.
Salt and Pepper Noise Artifact
For the salt and pepper noise artifact, we use the fol-
lowing four parameters:
IVAPP 2025 - 16th International Conference on Information Visualization Theory and Applications
762