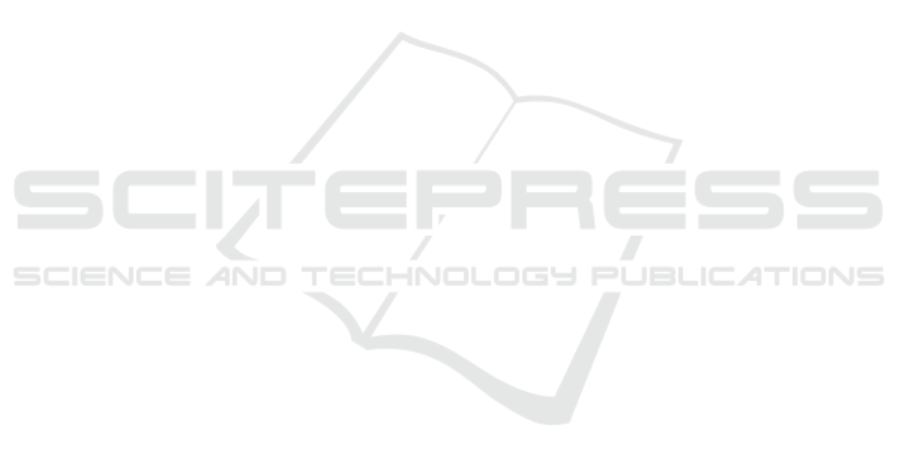
Dorodchi, M., Al-Hossami, E., Benedict, A., and Demeter,
E. (2019). Using synthetic data generators to promote
open science in higher education learning analytics. In
2019 IEEE International Conference on Big Data (Big
Data), pages 4672–4675. IEEE.
Drugova, E., Zhuravleva, I., Zakharova, U., and Latipov, A.
(2024). Learning Analytics driven Improvements in
Learning Design in higher Education: A systematic Lit-
erature Review. Journal of Computer Assisted Learning,
40(2):510–524.
Eitel-Porter, R. (2021). Beyond the promise: implementing
ethical AI. AI and Ethics, 1(1):73–80.
El Emam, K., Mosquera, L., and Hoptroff, R. (2020). Practi-
cal Synthetic Data Generation. O’Reilly Media, Inc.
Elmoazen, R., Saqr, M., Khalil, M., and Wasson, B. (2023).
Learning Analytics in virtual Laboratories: A system-
atic Literature Review of empirical Research. Smart
Learning Environments, 10(1).
European High-Level Expert Group on AI (2019). Ethics
guidelines for trustworthy AI — Shaping Europe’s digi-
tal future.
European Parliament (2023). Amendments adopted by the
European Parliament on 14 June 2023 on the proposal
for a regulation of the European Parliament and of the
Council on laying down harmonised rules on artificial
intelligence (Artificial Intelligence Act) and amending
certain Union legislative acts.
European Union (2024). Regulation (EU) 2024/1689 of the
European Parliament and of the Council of 13 June 2024
laying down harmonised rules on artificial intelligence.
Falco, G., Shneiderman, B., Badger, J., Carrier, R., Dahbura,
A., Danks, D., Eling, M., Goodloe, A., Gupta, J., Hart,
C., Jirotka, M., Johnson, H., LaPointe, C., Llorens, A. J.,
Mackworth, A. K., Maple, C., P
´
alsson, S. E., Pasquale,
F., Winfield, A., and Yeong, Z. K. (2021). Governing
AI safety through independent audits. Nature Machine
Intelligence, 3(7):566–571.
Fernsel, L., Kalff, Y., and Simbeck, K. (2024a). Assessing
the auditability of ai-integrating systems: A framework
and learning analytics case study. arXiv, 2411.08906.
Fernsel, L., Kalff, Y., and Simbeck, K. (2024b). Where is
the evidence? In Poquet, O., Ortega-Arranz, A., Viberg,
O., Chounta, I.-A., McLaren, B. M., and Jovanovic, J.,
editors, Proceedings of the 16th International Confer-
ence on Computer Supported Education (CSEDU 2024),
volume 2, pages 262–269.
Fjeld, J., Achten, N., Hilligoss, H., Nagy, A., et al. (2020).
Principled Artificial Intelligence: Mapping Consensus
in Ethical and Rights-Based Approaches to Principles
for AI. Berkman Klein Center Research Publication,
(1).
Galdon-Clavell, G., Zamorano, M. M., Castillo, C., Smith, O.,
et al. (2020). Auditing Algorithms: On Lessons Learned
and the Risks of Data Minimization. In Proceedings of
the AAAI/ACM Conference on AI, Ethics, and Society,
pages 265–271, New York. ACM.
Gardner, J., Brooks, C., and Baker, R. (2019). Evaluating the
Fairness of Predictive Student Models Through Slicing
Analysis. In Proceedings of the 9th International Con-
ference on Learning Analytics & Knowledge, LAK19,
pages 225–234, New York. ACM.
Garrison, D. R., Anderson, T., and Archer, W. (1999). Crit-
ical Inquiry in a Text-Based Environment: Computer
Conferencing in Higher Education. The Internet and
Higher Education, 2(2):87–105.
Gebru, T., Morgenstern, J., Vecchione, B., Vaughan, J. W.,
et al. (2021). Datasheets for datasets. Communications
of the ACM, 64(12):86–92.
Guidotti, R., Monreale, A., Ruggieri, S., Turini, F., Giannotti,
F., and Pedreschi, D. (2018). A Survey of Methods
for Explaining Black Box Models. ACM Computing
Surveys, 51(5):93:1–93:42.
Haim, A., Shaw, S., and Heffernan, N. (2023). How to open
science: A principle and reproducibility review of the
learning analytics and knowledge conference. In LAK23:
13th International Learning Analytics and Knowledge
Conference, pages 156–164.
Heikkinen, S., Saqr, M., Malmberg, J., and Tedre, M. (2023).
Supporting self-regulated Learning with Learning An-
alytics Interventions: A systematic Literature Review.
Education and Information Technologies, 28(3):3059–
3088.
Holland, S., Hosny, A., Newman, S., Joseph, J., and Chmielin-
ski, K. (2018). The Dataset Nutrition Label: A Frame-
work To Drive Higher Data Quality Standards.
Kale, A., Nguyen, T., Harris, Frederick C., Jr., Li, C., Zhang, J.,
and Ma, X. (2022). Provenance documentation to enable
explainable and trustworthy AI: A literature review.
Data Intelligence, pages 1–41.
Khalil, M., Slade, S., and Prinsloo, P. (2023). Learning Ana-
lytics in Support of Inclusiveness and disabled Students:
A systematic Review. Journal of Computing in Higher
Education, pages 202–219.
Kitto, K. and Knight, S. (2019). Practical ethics for build-
ing learning analytics. British Journal of Educational
Technology, 50(6):2855–2870.
Knechel, W. R., Krishnan, G. V., Pevzner, M., Shefchik, L. B.,
and Velury, U. K. (2013). Audit Quality: Insights from
the Academic Literature. AUDITING: A Journal of
Practice & Theory, 32(Supplement 1):385–421.
Kreuzberger, D., K
¨
uhl, N., and Hirschl, S. (2022). Machine
Learning Operations (MLOps): Overview, Definition,
and Architecture.
Landers, R. N. and Behrend, T. S. (2023). Auditing the AI
Auditors. A Framework for Evaluating Fairness and
Bias in high stakes AI predictive Models. The American
psychologist, 78(1):36–49.
Lang, C., Siemens, G., Friend Wise, A., Ga
ˇ
sevi
´
c, D., and Mer-
ceron, A., editors (2022). Handbook of Learning Ana-
lytics - Second edition. Society for Learning Analytics
Research (SoLAR), 2 edition.
Li, Y. and Goel, S. (2024). Artificial Intelligence Auditability
and Auditor Readiness for Auditing Artificial Intelli-
gence Systems. SSRN Journal.
Minkkinen, M., Niukkanen, A., and M
¨
antym
¨
aki, M. (2024).
What about investors? ESG analyses as tools for ethics-
based AI auditing. AI & Society, 39(1):329–343. PII:
1415.
Audits for Trust: An Auditability Framework for AI-Based Learning Analytics Systems
61