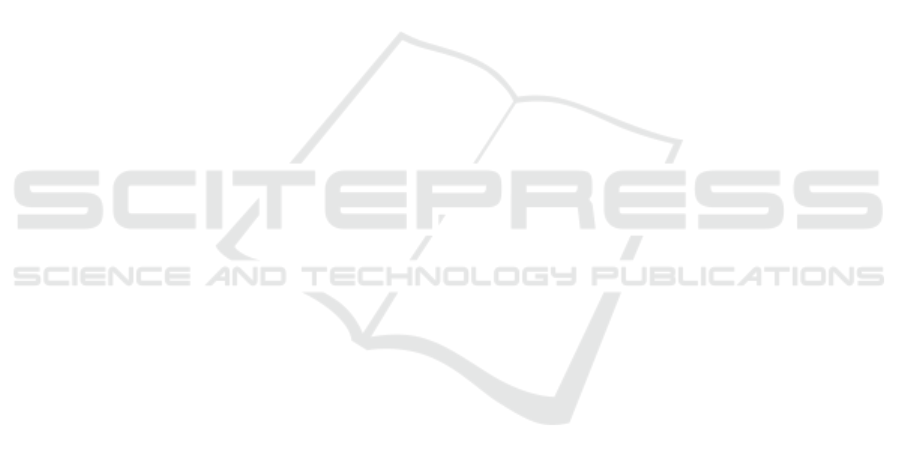
networks. Advances in neural information processing
systems, 25.
LeCun, Y., Bengio, Y., and Hinton, G. (2015). Deep learn-
ing. nature, 521(7553):436–444.
Li, F., Song, Z., Li, B., Wu, M. J., and Shen, C. (2015). De-
tecting shadow of moving object based on phong illu-
mination model. In First International Conference on
Information Sciences, Machinery, Materials and En-
ergy, pages 2004–2007. Atlantis Press.
Liu, J., Fang, T., and Li, D. (2011). Shadow detection in re-
motely sensed images based on self-adaptive feature
selection. IEEE Transactions on Geoscience and Re-
mote Sensing, 49(12):5092–5103.
Luo, S., Li, H., and Shen, H. (2020). Deeply supervised
convolutional neural network for shadow detection
based on a novel aerial shadow imagery dataset. IS-
PRS Journal of Photogrammetry and Remote Sensing,
167:443–457.
Luo, S., Shen, H., Li, H., and Chen, Y. (2019). Shadow re-
moval based on separated illumination correction for
urban aerial remote sensing images. Signal Process-
ing, 165:197–208.
Makarau, A., Richter, R., Muller, R., and Reinartz, P.
(2011). Adaptive shadow detection using a blackbody
radiator model. IEEE Transactions on Geoscience and
Remote Sensing, 49(6):2049–2059.
Margolin, R., Zelnik-Manor, L., and Tal, A. (2014). How
to evaluate foreground maps? In Proceedings of
the IEEE conference on computer vision and pattern
recognition, pages 248–255.
Otsu, N. et al. (1975). A threshold selection method from
gray-level histograms. Automatica, 11(285-296):23–
27.
Polidorio, A. M., Flores, F. C., Imai, N. N., Tommaselli,
A. M., and Franco, C. (2003). Automatic shadow seg-
mentation in aerial color images. In 16th brazilian
symposium on computer graphics and image process-
ing (SIBGRAPI 2003), pages 270–277. IEEE.
Ronneberger, O., Fischer, P., and Brox, T. (2015). U-
net: Convolutional networks for biomedical image
segmentation. In Medical image computing and
computer-assisted intervention–MICCAI 2015: 18th
international conference, Munich, Germany, October
5-9, 2015, proceedings, part III 18, pages 234–241.
Springer.
R
¨
ufenacht, D., Fredembach, C., and S
¨
usstrunk, S. (2013).
Automatic and accurate shadow detection using near-
infrared information. IEEE transactions on pattern
analysis and machine intelligence, 36(8):1672–1678.
Saha, J. and Chatterjee, A. (2017). Exploring the scope
of hsv color channels towards simple shadow contour
detection. In Pattern Recognition and Machine Intel-
ligence: 7th International Conference, PReMI 2017,
Kolkata, India, December 5-8, 2017, Proceedings 7,
pages 110–115. Springer.
Salvador, E., Cavallaro, A., and Ebrahimi, T. (2004).
Cast shadow segmentation using invariant color fea-
tures. Computer vision and image understanding,
95(2):238–259.
Sun, G., Huang, H., Weng, Q., Zhang, A., Jia, X., Ren, J.,
Sun, L., and Chen, X. (2019). Combinational shadow
index for building shadow extraction in urban areas
from sentinel-2a msi imagery. International Journal
of Applied Earth Observation and Geoinformation,
78:53–65.
Tsai, V. J. (2006). A comparative study on shadow compen-
sation of color aerial images in invariant color models.
IEEE transactions on geoscience and remote sensing,
44(6):1661–1671.
Vazquez, E., van de Weijer, J., and Baldrich, R. (2008).
Image segmentation in the presence of shadows and
highlights. In Computer Vision–ECCV 2008: 10th
European Conference on Computer Vision, Marseille,
France, October 12-18, 2008, Proceedings, Part IV
10, pages 1–14. Springer.
Vicente, T. F. Y., Hou, L., Yu, C.-P., Hoai, M., and Sama-
ras, D. (2016). Large-scale training of shadow de-
tectors with noisily-annotated shadow examples. In
Computer Vision–ECCV 2016: 14th European Con-
ference, Amsterdam, The Netherlands, October 11-
14, 2016, Proceedings, Part VI 14, pages 816–832.
Springer.
Wang, J., Li, X., and Yang, J. (2018). Stacked conditional
generative adversarial networks for jointly learning
shadow detection and shadow removal. In Proceed-
ings of the IEEE conference on computer vision and
pattern recognition, pages 1788–1797.
Wang, Q., Yan, L., Yuan, Q., and Ma, Z. (2017). An auto-
matic shadow detection method for vhr remote sens-
ing orthoimagery. Remote Sensing, 9(5):469.
Wang, Y., Liu, S., Li, L., Zhou, W., and Li, H. (2024).
Swinshadow: Shifted window for ambiguous adjacent
shadow detection. ACM Transactions on Multimedia
Computing, Communications and Applications.
Zerbe, L. M. and Liew, S. C. (2004). Reevaluating the
traditional maximum ndvi compositing methodology:
the normalized difference blue index. In IGARSS
2004. 2004 IEEE International Geoscience and Re-
mote Sensing Symposium, volume 4, pages 2401–
2404. IEEE.
Zhang, H., Sun, K., and Li, W. (2014). Object-oriented
shadow detection and removal from urban high-
resolution remote sensing images. IEEE transac-
tions on geoscience and remote sensing, 52(11):6972–
6982.
Zhao, M. and Bao, C. (1994). Image thresholding by his-
togram transformation. In Hybrid Image and Signal
Processing IV, volume 2238, pages 279–286. SPIE.
Zhou, B., Zhao, H., Puig, X., Fidler, S., Barriuso, A., and
Torralba, A. (2017). Scene parsing through ade20k
dataset. In Proceedings of the IEEE conference on
computer vision and pattern recognition, pages 633–
641.
Zhou, K., Wu, W., Shao, Y.-L., Fang, J.-L., Wang, X.-Q.,
and Wei, D. (2022). Shadow detection via multi-scale
feature fusion and unsupervised domain adaptation.
Journal of Visual Communication and Image Repre-
sentation, 88:103596.
ShadowScout: Robust Unsupervised Shadow Detection for RGB Imagery
667