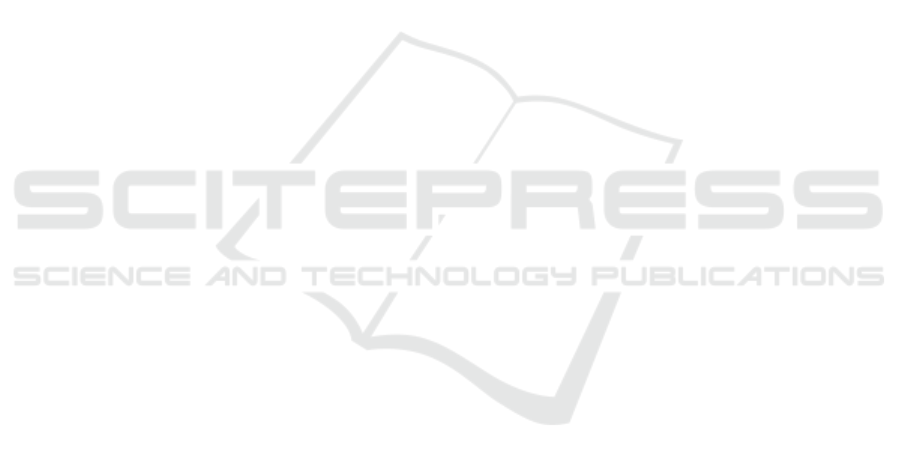
REFERENCES
Ahuja, G., Soda, G., and Zaheer, A. (2012). The genesis
and dynamics of organizational networks (march, pg
434, 2012). Organization Science, 23:1211–1211.
Angles, R., Arenas, M., Barcel
´
o, P., Hogan, A., Reutter, J.,
and Vrgo
ˇ
c, D. (2017). Foundations of modern query
languages for graph databases. ACM Computing Sur-
veys, 50(5):68:1–68:40.
Benjelloun, O., Garcia-Molina, H., Menestrina, D., Su, Q.,
Whang, S. E., and Widom, J. (2009). Swoosh: a
generic approach to entity resolution. The VLDB Jour-
nal, 18(1):255–276.
Bundesministerium f
¨
ur Bildung und Forschung (2024).
F
¨
orderkatalog - Database of Public Research Funding
in Germany. Federal database of publicly funded re-
search projects in Germany.
C
ˆ
ot
´
e, P.-O., Nikanjam, A., Ahmed, N., Humeniuk, D., and
Khomh, F. (2023). Data cleaning and machine learn-
ing: A systematic literature review. Publisher: [object
Object] Version Number: 1.
Elsevier (2024). Scopus. Abstract and citation database of
peer-reviewed literature.
European Patent Office (2024). PATSTAT - Worldwide
Patent Statistical Database. Global patent database
maintained by the European Patent Office.
Francis, N., Green, A., Guagliardo, P., Libkin, L., Lin-
daaker, T., Marsault, V., Plantikow, S., Rydberg, M.,
Selmer, P., and Taylor, A. (2018). Cypher: An evolv-
ing query language for property graphs. Proceedings
of the 2018 International Conference on Management
of Data.
Getoor, L. and Machanavajjhala, A. (2012). Entity resolu-
tion: Theory, practice & open challenges. Proceed-
ings of the VLDB Endowment, 5(12):2018–2019.
Hevner, A., R, A., March, S., T, S., Park, Park, J., Ram, and
Sudha (2004). Design science in information systems
research. Management Information Systems Quar-
terly, 28:75.
Hogan, A., Blomqvist, E., Cochez, M., D’amato, C., Melo,
G. D., Gutierrez, C., Kirrane, S., Gayo, J. E. L., Nav-
igli, R., Neumaier, S., Ngomo, A.-C. N., Polleres, A.,
Rashid, S. M., Rula, A., Schmelzeisen, L., Sequeda,
J., Staab, S., and Zimmermann, A. (2021). Knowledge
graphs. ACM Computing Surveys, 54(4):71:1–71:37.
Huang, Y., Li, R., Zou, F., Jiang, L., Porter, A. L., and
Zhang, L. (2022). Technology life cycle analysis:
From the dynamic perspective of patent citation net-
works. Technological Forecasting and Social Change,
181:121760.
Javed, M. A., Younis, M. S., Latif, S., Qadir, J., and Baig,
A. (2018). Community detection in networks: A mul-
tidisciplinary review. Journal of Network and Com-
puter Applications, 108:87–111.
Kumar, S. and Hanot, R. (2021). Community detection al-
gorithms in complex networks: A survey. In Thampi,
S. M., Krishnan, S., Hegde, R. M., Ciuonzo, D.,
Hanne, T., and Kannan R., J., editors, Advances in Sig-
nal Processing and Intelligent Recognition Systems,
pages 202–215. Springer.
Long, J. C., Cunningham, F. C., Carswell, P., and Braith-
waite, J. (2014). Patterns of collaboration in complex
networks: the example of a translational research net-
work. BMC Health Services Research, 14(1):225.
Noy, N., Gao, Y., Jain, A., Narayanan, A., Patterson, A., and
Taylor, J. (2019). Industry-scale knowledge graphs:
Lessons and challenges: Five diverse technology com-
panies show how it’s done. Queue, 17(2):Pages
20:48–Pages 20:75.
Ozcan, S. and Islam, N. (2017). Patent information re-
trieval: approaching a method and analysing nan-
otechnology patent collaborations. Scientometrics,
111(2):941–970.
Que, X., Checconi, F., Petrini, F., and Gunnels, J. A. (2015).
Scalable community detection with the louvain algo-
rithm. In 2015 IEEE International Parallel and Dis-
tributed Processing Symposium, pages 28–37. ISSN:
1530-2075.
Raghavan, U. N., Albert, R., and Kumara, S. (2007).
Near linear time algorithm to detect community struc-
tures in large-scale networks. Physical Review E,
76(3):036106.
Rahm, E. and Do, H. H. (2000). Data cleaning: Problems
and current approaches. IEEE Data Engineering Bul-
letin, 23(4):3–13.
Sattar, N. S. and Arifuzzaman, S. (2022). Scalable dis-
tributed louvain algorithm for community detection
in large graphs. The Journal of Supercomputing,
78(7):10275–10309.
Schwartz, M., Peglow, F., Fritsch, M., and G
¨
unther, J.
(2012). What drives innovation output from subsi-
dized r&d cooperation?—project-level evidence from
germany. Technovation, 32(6):358–369.
Shvaiko, P. and Euzenat, J. (2013). Ontology match-
ing: State of the art and future challenges. IEEE
Transactions on Knowledge and Data Engineering,
25(1):158–176. Conference Name: IEEE Transac-
tions on Knowledge and Data Engineering.
Valente, T. W., Coronges, K., Lakon, C., and Costenbader,
E. (2008). How correlated are network centrality mea-
sures? Connections (Toronto, Ont.), 28(1):16–26.
Wang, L. (2017). Heterogeneous data and big data ana-
lytics. Automatic Control and Information Sciences,
3(1):8–15. Number: 1 Publisher: Science and Educa-
tion Publishing.
Connection Is all You Need! Mining and Linking Disparate Data Sources for Collaboration Network Analysis
217