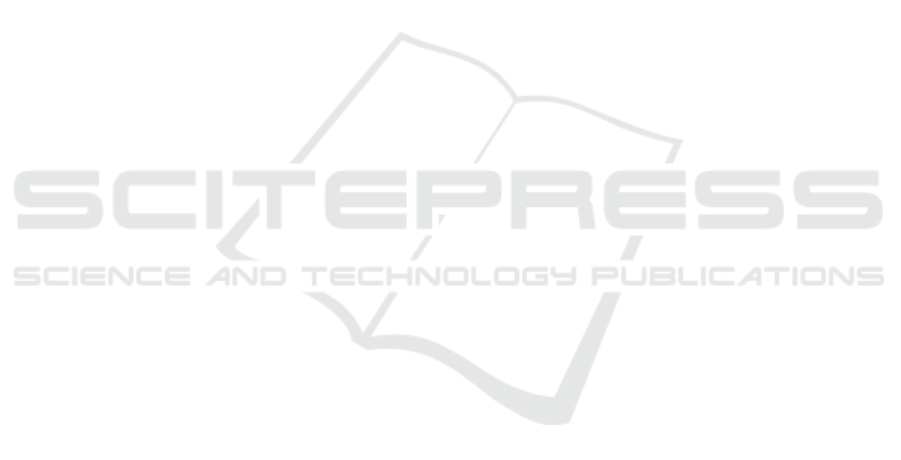
(Altenstetter and Busse, 2005).
In 2016, Medical Advisory Board initiated a joint
evaluation of existing quality assurance initiatives and
the development of a comprehensive, standardised
quality assurance process for all areas of assessment.
This process was developed with the involvement of
all regional Medical Advisory Boards and specialist
competence units, which set the standards for assess-
ment in each specialist area. The Medical Advisory
Boards have trialled the new national quality assur-
ance plan in three test runs throughout Germany, pi-
loted it in the largest assessment area and then gradu-
ally implemented it in practice in all assessment areas.
Finally, the national quality assurance plan was even
taken up by the legislature, incorporated into the Ger-
man Social Code and, following a broad national dis-
cussion among all stakeholders and interested parties,
adopted in a guideline confirmed by the Federal Min-
istry of Health with sub-legal binding force. Quality
assurance follows the generally recognised principles
of the quality assurance cycle for continuous eval-
uation and further development, see (Gemeinsamer
Bundesausschuss, 2023).
The assessment is carried out by the Medical Ad-
visory Boards, which are essentially organised by fed-
eral state. Accordingly, an initial quality check is car-
ried out in the regionally responsible Medical Advi-
sory Board. For quality assurance purposes, the as-
sessments are randomly drawn on a nationwide basis.
The special feature of the newly implemented system
is that a partial sample is sent to another Medical Ad-
visory Boards for a second independent assessment.
If there are noticeable differences between the self-
assessment and external assessment, a further qual-
ity check is carried out by a third Medical Advisory
Board.
The entire process of drawing up and providing
expert opinions, along with all the stages of the audit
described above, is conducted via a central server on
which all the expert opinions specified for the audit
are accessible in anonymised form, see (Medizinis-
cher Dienst Bund, 2023), (Ries et al., 2020) and (Ries
et al., 2023).
Should discrepancies remain following the third
stage of the examinations, a discussion and consen-
sus are reached on the specific case construction at
nationwide consensus conferences. After consensus
conferences were held for the first time in 2018 as
part of the feasibility study, they are now established
as a nationwide quality assurance instrument. They
promote mutual learning and take place twice a year
in each of the nine assessment areas, once in person
and once online via video conference. Representa-
tives of all regional Medical Advisory Boards take
part in each consensus conference, as well as at least
one representative of the assigned specialist compe-
tence unit. This makes it possible to promptly im-
plement the need for further development in the regu-
lations identified in the consensus conferences, as the
competence units set the standards for the assessment.
A total of 20 criteria have been established for the
evaluation of the assessment, which are standardised
across all assessment areas. Furthermore, additional
technical criteria are currently being harmonised with
the general criteria. In addition to the aforementioned
formal criteria, there are four criteria of central im-
portance for standardised assessment processes in the
regional Medical Advisory Boards, cf. (Gaertner and
van Essen, 2024), (Gaertner and Gnatzy, 2011).
Three of these central criteria are operationalised
as three test aspects for the accuracy of the expert
opinion, namely criteria 14, 15 and 16. Triggered by
limitations on the transfer of the case file due to data
protection regulations that vary in strictness from re-
gion to region, the national quality assurance plan has
created the new requirement for expert opinions with
the fourth central criterion 9 that they must be con-
vincing with a concise presentation of the character-
istics of the individual case that are relevant to the
assessment.
2 MATERIALS AND METHODS
The following analysis focuses on the medical fields
(cf. (Anja Dippmann, 2024)):
• 100: Incapacity of work
• 200: Hospital care
• 400: New and unconventional examination and
treatment methods / pharmacy
• 500: Prevention and rehabilitation
• 700: Medical supplies
In the year under review 2023, 16942 internal and
4953 external evaluations were carried out in these
medical fields. In addition, only the central criteria
• Criterion 9: The report contains the information
necessary to assess the facts of the case
• Criterion 14: The expert opinion takes into ac-
count current medical knowledge
• Criterion 15: The expert opinion takes into ac-
count the socio-medical requirements
• Criterion 16: The result of the expert opinion is
plausible and understandable in the context of the
facts presented
Relationships Between Central Quality Assurance Criteria for the Assessment of Statutory Health Insurance Patients for Further
Development Using Medical Informatics Methods
713