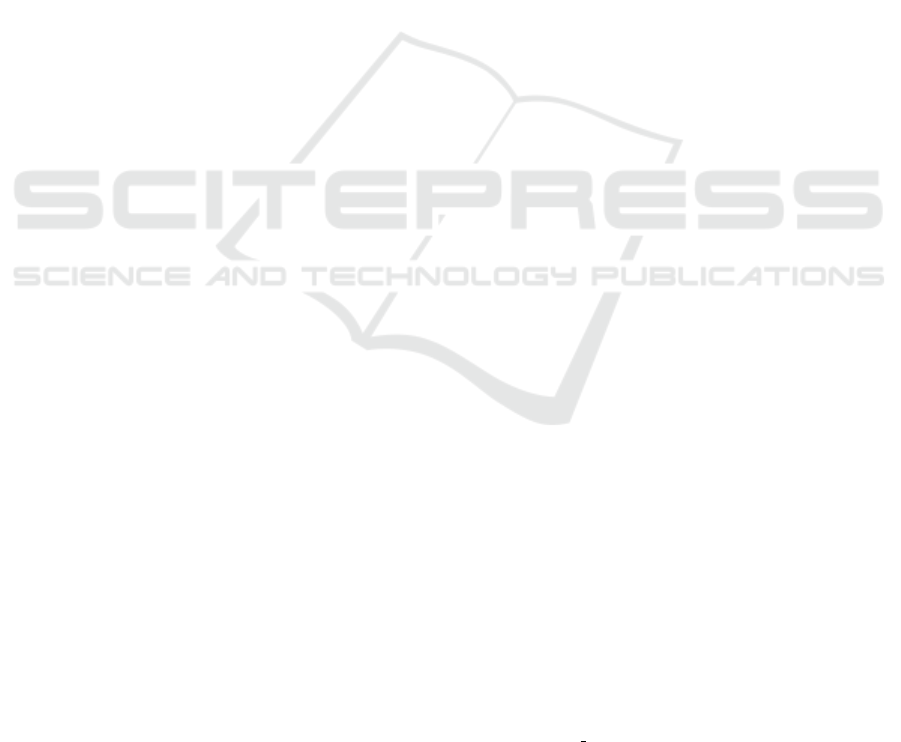
to further validate the feasibility of our method.
In addition, in games like the “Pok
´
emon Trad-
ing Card Game”, card texts often lack explicit inter-
actions, which necessitates relying primarily on co-
occurrence rates for synergy score calculations. To
address this limitation, we plan to simulate and col-
lect gameplay data to evaluate how cards interact in
practice, aiming to explore hidden synergies that are
not explicitly described in their texts.
6.3 Reflection on the Evaluation
Method
The lack of similar studies on DCCGs presents a
significant challenge to conducting objective, cross-
comparative evaluations of this tool. This has resulted
in current evaluations relying heavily on subjective re-
sponses, which are less convincing.
Furthermore, the small and homogeneous sample
size used in the evaluation limits the generalizabil-
ity of the findings. Since our study does not include
features to introduce the game, participants were re-
quired to have a basic understanding of DCCGs. Re-
lying solely on experienced DCCG players may intro-
duce bias by excluding the perspectives of beginners.
Expanding the participant pool to include a more di-
verse audience and integrating qualitative data is one
of our future plans to strengthen the robustness of the
evaluation.
7 CONCLUSIONS AND FUTURE
WORK
In this paper, we introduced a novel approach to visu-
alizing card synergies to overcome information over-
load in DCCGs through a graph-based visualization
framework. To test it, we visualized card synergies for
YGOMD and conducted a questionnaire survey with
a usability test involving recruited volunteers. Al-
though there are areas that require improvement, par-
ticipant feedback confirmed the effectiveness of our
visualization approach.
Our ultimate goal is to evolve this framework into
a comprehensive support tool, enabling our synergy
analysis method to be applied across most DCCGs.
Based on the results of this study, we will continue re-
fining the framework to benefit both players and game
designers, helping users gain deeper insights into card
synergies and the game itself.
ACKNOWLEDGMENT
This work was partly supported by JSPS KAKENHI
Grant Number JP24K14904.
REFERENCES
Chen, Z., Amato, C., Nguyen, T.-H. D., Cooper, S., Sun, Y.,
and El-Nasr, M. S. (2018). Q-DeckRec: A fast deck
recommendation system for collectible card games. In
Proc. IEEE CIG, pages 1–8.
Dockhorn, A. and Mostaghim, S. (2019). Introducing the
Hearthstone-AI competition. arXiv, 1906.04238.
Duel Links Meta LLC (2024). Top decks. https://www.
masterduelmeta.com/top-decks.
e Silva Vieira, R., Tavares, A. R., and Chaimowicz, L.
(2024). A taxonomy of collectible card games from
a game-playing AI perspective. arXiv, 2410.06299.
Forgin Bits (2024). Force directed diagram. https://www.
youtube.com/watch?v=hWt5WPO8c6A.
Fudolig, M., Alshaabi, T., Arnold, M., Danforth, C., and
Dodds, P. (2022). Sentiment and structure in word co-
occurrence networks on Twitter. Appl. Netw. Sci., 7:9.
Konami Digital Entertainment (2021). Yu-Gi-Oh! Master
Duel has over 10,000 cards to unlock. https://www.
konami.com/games/us/en/topics/1752/.
Puerta, P., Laguna, L., Vidal, L., Ares, G., Fiszman, S.,
and T
´
arrega, A. (2020). Co-occurrence networks of
Twitter content after manual or automatic processing.
a case-study on “gluten-free”. Food Qual. Prefer.,
86:103993.
Strother, J. B., Ulijn, J. M., and Fazal, Z. (2012). Visualiz-
ing instead of overloading: Exploring the promise and
problems of visual communication to reduce informa-
tion overload. In Information Overload: An Interna-
tional Challenge for Professional Engineers and Tech-
nical Communicators, pages 203–229. Wiley-IEEE
Press.
Swacha, J. (2021). Three dimensions of science: A web tool
for 3d visualization of scientific literature. In Proc.
ACM/IEEE JCDL, pages 274–277.
Turkay, S. and Adinolf, S. (2018). Understanding on-
line collectible card game players’ motivations: a sur-
vey study with two games. In Proc. OzCHI, page
501–505. ACM Press.
Vieira, R., Tavares, A. R., and Chaimowicz, L. (2020).
Drafting in collectible card games via reinforcement
learning. In Proc. SBGames, pages 54–61. IEEE CPS.
Wang, S., Li, W., and Gu, Z. (2023). GeoGraphViz:
Geographically constrained 3D force-directed graph
for knowledge graph visualization. Trans. GIS,
27(4):931–948.
Yu-Gi-Oh! Wiki (2024). Gaming terms. https:
//yugioh.\linebreak[0]fandom.com/wiki/Category:
Gaming\
Terms.
Zuin, G., Chaimowicz, L., and Veloso, A. (2022). Deep
learning techniques for explainable resource scales
in collectible card games. IEEE Trans. Games,
14(1):46–55.
HUCAPP 2025 - 9th International Conference on Human Computer Interaction Theory and Applications
618