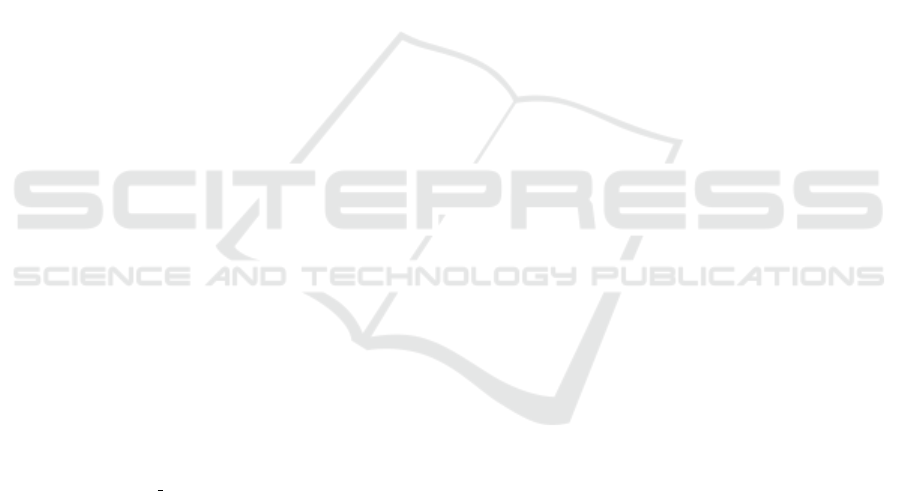
ACKNOWLEDGEMENTS
This work was supported by JSPS KAKENHI Grant
Numbers JP22K12157, JP23K28377, JP24H00714.
This work was conducted using the comput-
ers at the Artificial Intelligence eXploration Re-
search Center (AIX) at the University of Electro-
Communications.
We acknowledge the assistance for the GPT-
4o (OpenAI, 2024c), OpenAI o1-preview (OpenAI,
2024e), OpenAI o1 (OpenAI, 2024d) and Anthropic
Claude 3.5 Sonnet (Anthropic, 2024) were used for
proofreading, which was further reviewed and revised
by the authors.
The pun database used in this work was developed
under JSPS KAKENHI Grant-in-Aid for Scientific
Research (C) Grant Number 17K00294. We would
like to express our gratitude to Professor Kenji Araki
of the Language Media Laboratory, Division of Me-
dia and Network Technologies, Faculty of Informa-
tion Science and Technology, Hokkaido University,
for providing the pun database.
REFERENCES
Anthropic (2024). Introducing claude 3.5 sonnet. https:
//www.anthropic.com/news/claude-3-5-sonnet. (Ac-
cessed on 11/07/2024).
Araki, K. (2018). Performance evaluation of pun generation
system using pun database in japanese. Proceedings
SIG-LSE-B703-8, The Japanese Society for Artificial
Intelligence, 2nd Workshops (in Japanese).
Araki, K., Sayama, K., Uchida, Y., and Yatsu, M.
(2018). Expansion and analysis of a fashionable
database. JSAI Type 2 Study Group Language Engi-
neering Study Group Material (SIG-LSE-B803-1) (in
Japanese), pages 1–15.
Araki, K., Uchida, Y., Sayama, K., and Tazu, M. (2020).
Pun database. http://arakilab.media.eng.hokudai.ac.
jp/
∼
araki/dajare eng.htm. (Accessed on 11/05/2024).
Chen, Y., Yang, C., Hu, T., Chen, X., Lan, M ., Cai, L.,
Zhuang, X., Lin, X., Lu, X., and Zhou, A. (2024). Are
U a joke master? pun generation via multi-stage cur-
riculum learning towards a humor LLM. In Findings
of the ACL 2024.
D’Oosterlinck, K., Xu, W., Develder, C., Demeester, T.,
Singh, A., Potts, C., Kiela, D., and Mehri, S. (2024).
Anchored preference optimization and contrastive re-
visions: Addressing underspecification in alignment.
https://arxiv.org/abs/2408.06266.
Goodfellow, I., Pouget-Abadie, J., Mirza, M., Xu, B.,
Warde-Farley, D., Ozair, S., Courville, A., and Ben-
gio, Y. (2014). Generative adversarial networks. Ad-
vances in Neural Information Processing Systems, 3.
Hatakeyama, K. and Tokunaga, T. (2021). Automatic gener-
ation of japanese juxtaposition puns using transformer
models. 27th Annual Meeting of the Language Pro-
cessing Society of Japan (in Japanese).
Hu, E. J., Shen, Y., Wallis, P., Allen-Zhu, Z., Li, Y., Wang,
S., Wang, L., and Chen, W. (2022). LoRA: Low-rank
adaptation of large language models. In ICLR.
Jentzsch, S. and Kersting, K. (2023). ChatGPT is fun, but it
is not funny! humor is still challenging large language
models. In Proceedings WASSA, pages 325–340.
Luo, F., Li, S., Yang, P., Li, L., Chang, B., Sui, Z., and Sun,
X. (2019). Pun-gan: Generative adversarial network
for pun generation. In Proceedings of the 2019 Con-
ference on EMNLP-IJCNLP, pages 3388–3393.
Minami, T., Sei, Y., Tahara, Y., and Osuga, A. (2023). An
investigation of pun generation models using para-
phrasing with deep reinforcement learning. 15th Fo-
rum on Data Engineering and Information Manage-
ment (DBSJ 21th Annual Meeting) (in Japanese).
OpenAI (2022). ChatGPT: Optimizing language models for
dialogue. https://openai.com/blog/chatgpt/. (Accessed
on 02/05/2024).
OpenAI (2024a). Embeddings - OpenAI API. https:
//platform.openai.com/docs/guides/embeddings. (Ac-
cessed on 02/04/2024).
OpenAI (2024b). GPT-4o mini: advancing cost-
efficient intelligence. https://openai.com/index/
gpt-4o-mini-advancing-cost-efficient-intelligence/.
(Accessed on 08/25/2024).
OpenAI (2024c). Hello GPT-4o. https://openai.com/index/
hello-gpt-4o/. (Accessed on 11/07/2024).
OpenAI (2024d). Introducing OpenAI o1. https://openai.
com/o1/. (Accessed on 01/04/2025).
OpenAI (2024e). Introducing OpenAI o1-preview. https:
//openai.com/index/introducing-openai-o1-preview/.
(Accessed on 11/07/2024).
Rafailov, R., Sharma, A., Mitchell, E., Ermon, S., Manning,
C. D., and Finn, C. (2024). Direct preference opti-
mization: Your language model is secretly a reward
model. https://arxiv.org/abs/2305.18290.
Ritsumeikan University Dajare Club (2020). rits-
dajare/daas: Dajare as a service japanese pun de-
tection / evaluation engine). https://github.com/
rits-dajare/daas. (Accessed on 10/31/2024).
Yatsu, M. and Araki, K. (2018). Comparison of pun de-
tection methods using Japanese pun corpus. In Pro-
ceedings of the Eleventh International Conference on
Language Resources and Evaluation (LREC 2018).
Zhao, W. X. et al. (2023). A survey of large language mod-
els. arXiv preprint arXiv:2303.18223.
Zheng, L., Chiang, W.-L., Sheng, Y., Zhuang, S., Wu, Z.,
Zhuang, Y., Lin, Z., Li, Z., Li, D., Xing, E. P., Zhang,
H., Gonzalez, J. E., and Stoica, I. (2024). Judging
llm-as-a-judge with mt-bench and chatbot arena. In
Proceedings of the 37th International Conference on
NeurIPS.
Ziegler, D. M., Stiennon, N., Wu, J., Brown, T. B., Rad-
ford, A., Amodei, D., Christiano, P., and Irving, G.
(2020). Fine-tuning language models from human
preferences. https://arxiv.org/abs/1909.08593.
ICAART 2025 - 17th International Conference on Agents and Artificial Intelligence
1100