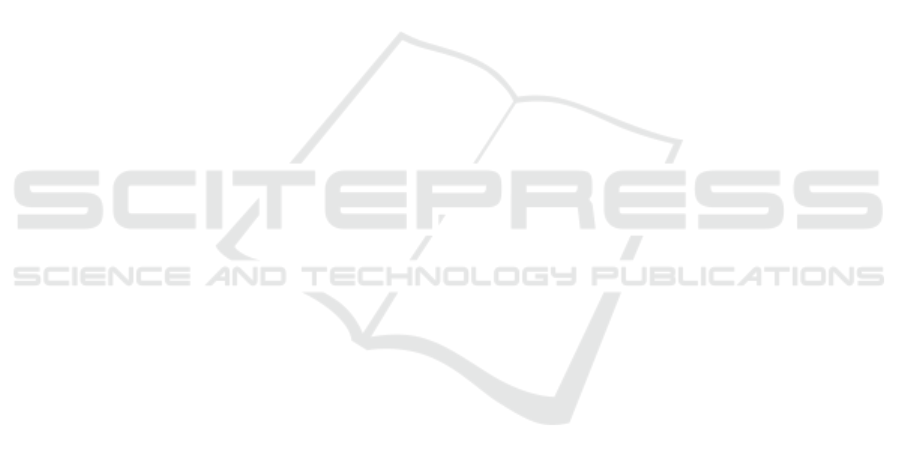
mental health scenarios.
Finally, our framework has a plug-and-play de-
sign that facilitates the use of both small open-source
models and larger, state-of-the-art models. While the
current implementation uses lightweight open source
models to keep the system efficient and accessible, the
framework is compatible with more advanced, pre-
trained models that can be integrated off-the-shelf as
needed. This flexibility allows for scalable model up-
grades, providing a pathway to incorporate more pow-
erful agents as needed without changing the system
architecture. Importantly, the expert agents processes
only anonymized data, ensuring that any model re-
placement or upgrade respects user privacy and data
security.
6 CONCLUSION
This paper presents the development and evaluation
of the proof of concept MentalRAG system, an agen-
tic framework for therapeutic support systems, with a
particular focus on suicide risk assessment and psy-
chological counselling. The system’s refined models
exhibited notable enhancements in the provision of
empathetic and contextually tailored responses, par-
ticularly in delicate scenarios such as emotional dis-
tress and crisis management.
The MentalRAG system employs a multi-agent
structure that leverages distinct specialized agents to
handle various aspects of mental health support, from
symptom identification to therapeutic proposal gen-
eration. The architecture of the system begins with
the Conversation Agent, which interacts with users
in real time, followed by the Anonymization Agent
to protect user privacy. Expert agents, including
the Symptoms Detection, Clinical Issues Detection,
and Suicide Detection Agents, provide diagnostic in-
sights by analyzing conversation data. The Report
Agent synthesizes these diagnostics into a cohesive
report, while the Proposal Agent generates personal-
ized therapeutic recommendations. This multi-agent
approach, combined with a human-in-the-loop vali-
dation process, ensures accurate, secure, and ethically
guided support in mental health applications.
Despite its potential, several limitations remain.
The evaluation was done by a single psychiatrist, in
future works it needs more specialists to reduce the
possibility of a bias. Also, the system’s “black box”
nature poses a risk in mental health applications, ne-
cessitating further safeguards to ensure safe and em-
pathetic interactions, mitigating biases and errors due
to its nature. Future work should focus on incorporat-
ing multimodal inputs (e.g., voice, video) to enhance
interaction depth and integrating additional datasets
for domain-specific fine-tuning.
By addressing these areas, the MentalRAG system
can evolve into a more reliable, efficient, and ethically
sound mental health support tool, better equipped to
meet user needs while minimizing risks.
ACKNOWLEDGEMENTS
The authors are deeply grateful to Dr. Daniel Neto,
whose contributions to the expert evaluation were
invaluable. His detailed feedback and specialized
knowledge as a PhD psychiatrist provided critical in-
sights that significantly enhanced the quality and rel-
evance of this project. This work was supported by
national funds through Fundac¸
˜
ao para a Ci
ˆ
encia e a
Tecnologia (FCT) with reference UIDB/50021/2020
and FCT Project WSMART ROUTE+ reference
2022.04180.PTDC.
REFERENCES
Brown, T. B., Mann, B., Ryder, N., Subbiah, M., Kaplan,
J., Dhariwal, P., Neelakantan, A., Shyam, P., Sas-
try, G., Askell, A., Agarwal, S., Herbert-Voss, A.,
Krueger, G., Henighan, T., Child, R., Ramesh, A.,
Ziegler, D. M., Wu, J., Winter, C., Hesse, C., Chen,
M., Sigler, E., Litwin, M., Gray, S., Chess, B., Clark,
J., Berner, C., McCandlish, S., Radford, A., Sutskever,
I., and Amodei, D. (2020). Language models are few-
shot learners. In Proceedings of the 34th Interna-
tional Conference on Neural Information Processing
Systems, NIPS ’20, Red Hook, NY, USA. Curran As-
sociates Inc.
Capital, S. (2024). What’s next for AI Agentic Workflows
ft. Andrew Ng of AI Fund.
Chowdhery, A., Narang, S., Devlin, J., Bosma, M., Mishra,
G., Roberts, A., Barham, P., Chung, H. W., Sutton,
C., Gehrmann, S., Schuh, P., Shi, K., Tsvyashchenko,
S., Maynez, J., Rao, A., Barnes, P., Tay, Y., Shazeer,
N., Prabhakaran, V., Reif, E., Du, N., Hutchinson,
B., Pope, R., Bradbury, J., Austin, J., Isard, M., Gur-
Ari, G., Yin, P., Duke, T., Levskaya, A., Ghemawat,
S., Dev, S., Michalewski, H., Garcia, X., Misra, V.,
Robinson, K., Fedus, L., Zhou, D., Ippolito, D., Luan,
D., Lim, H., Zoph, B., Spiridonov, A., Sepassi, R.,
Dohan, D., Agrawal, S., Omernick, M., Dai, A. M.,
Pillai, T. S., Pellat, M., Lewkowycz, A., Moreira, E.,
Child, R., Polozov, O., Lee, K., Zhou, Z., Wang, X.,
Saeta, B., Diaz, M., Firat, O., Catasta, M., Wei, J.,
Meier-Hellstern, K., Eck, D., Dean, J., Petrov, S., and
Fiedel, N. (2023). Palm: scaling language modeling
with pathways. J. Mach. Learn. Res., 24(1).
Chung, H. W., Hou, L., Longpre, S., Zoph, B., Tay, Y., Fe-
dus, W., Li, Y., Wang, X., Dehghani, M., Brahma,
ICT4AWE 2025 - 11th International Conference on Information and Communication Technologies for Ageing Well and e-Health
56