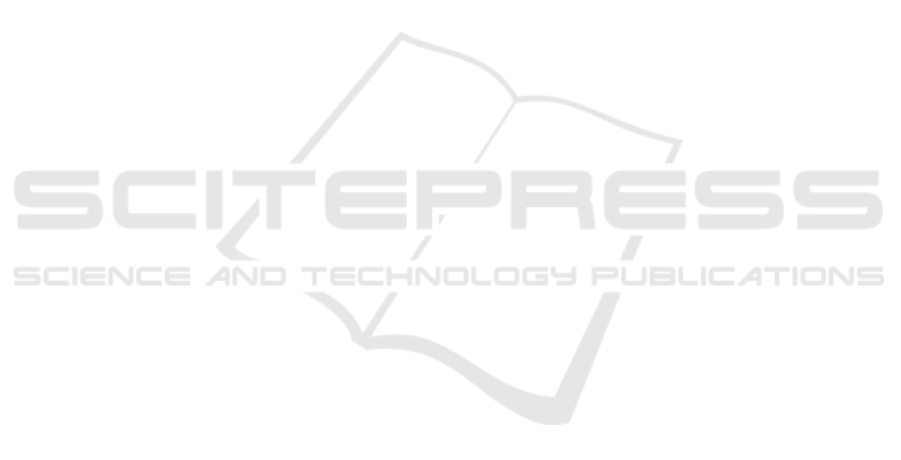
issues in deployed IoT stacks, particularly when de-
velopers contribute with hints, data dictionaries, and
parameter specifications. These inputs can be man-
ually added or automatically extracted from existing
functional tests within our framework. Future plans
include expanding the application set and source code
issues for better method comparisons and investing in
persistence testing with symbolic and concolic execu-
tion to provide faster feedback by identifying linked
parameters.
ACKNOWLEDGEMENTS
This research was supported by European Union’s
Horizon Europe research and innovation programme
under grant agreement no. 101070455, project DYN-
ABIC.
REFERENCES
Al-Hadhrami, Y. and Hussain, F. K. (2021). DDoS attacks
in IoT networks: a comprehensive systematic litera-
ture review. World Wide Web, 24(3):971–1001.
Atlidakis, V., Godefroid, P., and Polishchuk, M. (2019).
RESTler: Stateful REST API Fuzzing. In 2019
IEEE/ACM 41st ICSE, pages 748–758. ISSN: 1558-
1225.
Bures, M., Klima, M., Rechtberger, V., Bellekens, X., Tach-
tatzis, C., Atkinson, R., and Ahmed, B. S. (2020). In-
teroperability and Integration Testing Methods for IoT
Systems: A Systematic Mapping Study. In Software
Engineering and Formal Methods, pages 93–112.
Cristea, R., Feraru, M., and Paduraru, C. (2022). Building
blocks for IoT testing - a benchmark of IoT apps and a
functional testing framework. In 2022 IEEE/ACM 4th
International Workshop (SERP4IoT), pages 25–32.
Cristea, R. and P
˘
aduraru, C. (2023). An experiment to build
an open source application for the Internet of Things
as part of a software engineering course. In 2023
IEEE/ACM 5th International Workshop (SERP4IoT).
Dias, J. P., Couto, F., Paiva, A. C., and Ferreira, H. S.
(2018). A Brief Overview of Existing Tools for Test-
ing the Internet-of-Things. In 2018 IEEE ICST, pages
104–109.
Do, H., Mirarab, S., Tahvildari, L., and Rothermel, G.
(2008). An empirical study of the effect of time con-
straints on the cost-benefits of regression testing. In
Proceedings of the 16th ACM SIGSOFT International
Symposium on FSE.
Eceiza, M., Flores, J. L., and Iturbe, M. (2021). Fuzzing
the Internet of Things: A Review on the Techniques
and Challenges for Efficient Vulnerability Discovery
in Embedded Systems. IEEE Internet of Things Jour-
nal. Conference Name: IEEE Internet of Things Jour-
nal.
El-hajj, M., Fadlallah, A., Chamoun, M., and Serhrouchni,
A. (2019). A Survey of Internet of Things (IoT) Au-
thentication Schemes. Sensors, 19(5):1141. Number:
5 Publisher: Multidisciplinary Digital Publishing In-
stitute.
Fioraldi, A., Maier, D., Eißfeldt, H., and Heuse, M. (2020).
AFL++ : Combining Incremental Steps of Fuzzing
Research.
Gaborovi
´
c, A., Kari
´
c, K., Blagojevi
´
c, M., and Pla
ˇ
si
´
c, J.
(2022). Comparative analysis of ISO/IEC and IEEE
standards in the field of Internet of Things.
Gopinath, R. and Zeller, A. (2019). Building Fast Fuzzers.
arXiv:1911.07707 [cs].
Hemmati, H. (2015). How Effective Are Code Coverage
Criteria? In 2015 IEEE International Conference on
Software Quality, Reliability and Security, pages 151–
156.
Sadique, K., Rahmani, R., and Johannesson, P. (2020).
“IMSC-EIoTD: Identity Management and Secure
Communication for Edge IoT Devices”. en. In: Sen-
sors 20.22 (). (Visited on 03/07/2022).
K
¨
uhn, F., Hellbr
¨
uck, H., and Fischer, S. (2018). A Model-
based Approach for Self-healing IoT Systems:. In
Proceedings of the 7th International Conference on
Sensor Networks, pages 135–140.
Lin, J., Li, T., Chen, Y., Wei, G., Lin, J., Zhang, S., and
Xu, H. (2022). foREST: A Tree-based Approach for
Fuzzing RESTful APIs. arXiv:2203.02906 [cs].
Liu, C. (2005). Enriching software engineering courses
with service-learning projects and the open-source ap-
proach. In Proceedings of the 27th ICSE, pages 613–
614. ACM.
Martino, B. D., Esposito, A., and Cretella, G. (2016). To-
wards a IoT Framework for the Matchmaking of Sen-
sors’ Interfaces. In 2016 Intl IEEE Conferences on
Ubiquitous Intelligence & Computing (UIC/ATC/S-
calCom/CBDCom/IoP/SmartWorld), pages 888–894.
P
˘
aduraru, C., Cristea, R., and St
˘
aniloiu, E. (2021). Rive-
rIoT - a Framework Proposal for Fuzzing IoT Appli-
cations. In 2021 IEEE/ACM 3rd International Work-
shop (SERP4IoT), pages 52–58.
Seeger, J., Br
¨
oring, A., and Carle, G. (2020). Optimally
Self-Healing IoT Choreographies. ACM Transactions
on Internet Technology, 20(3):27:1–27:20.
Tzavaras, A., Mainas, N., and Petrakis, E. G. M. (2023).
OpenAPI framework for the Web of Things. Internet
of Things, 21:100675.
Wang, J., Chen, B., Wei, L., and Liu, Y. (2017). Skyfire:
Data-Driven Seed Generation for Fuzzing. In 2017
IEEE Symposium on Security and Privacy (SP), pages
579–594. ISSN: 2375-1207.
Wu, Y. (2021). Cloud-Edge Orchestration for the Internet of
Things: Architecture and AI-Powered Data Process-
ing. IEEE Internet of Things Journal, 8(16):12792–
12805. Conference Name: IEEE Internet of Things
Journal.
Zheng, Y., Davanian, A., Yin, H., Song, C., Zhu, H., and
Sun, L. (2019). FIRM-AFL: high-throughput greybox
fuzzing of iot firmware via augmented process emula-
tion. In Proceedings of the 28th USENIX Conference
on Security Symposium.
Zhu, X., Wen, S., Camtepe, S., and Xiang, Y. (2022).
Fuzzing: A Survey for Roadmap. ACM Computing
Surveys, pages 230:1–230:36.
ENASE 2025 - 20th International Conference on Evaluation of Novel Approaches to Software Engineering
448