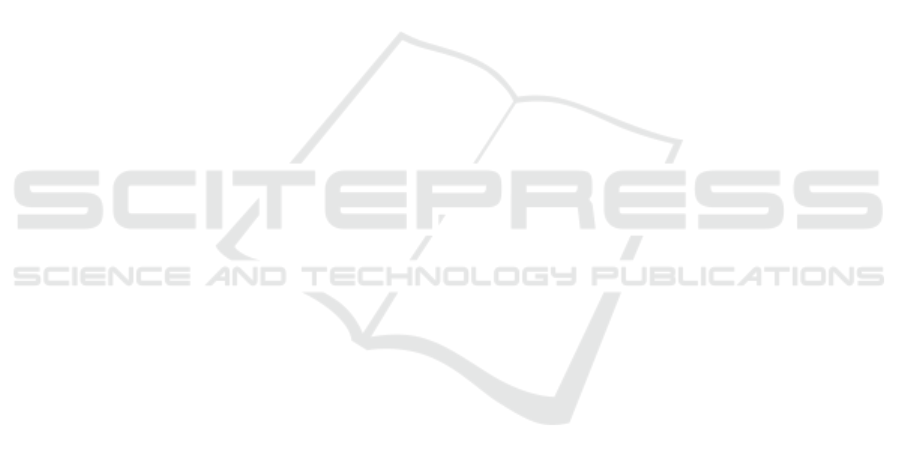
ACKNOWLEDGEMENTS
The authors thank the Pontif
´
ıcia Universidade
Cat
´
olica de Minas Gerais – PUC-Minas and
Coordenac¸
˜
ao de Aperfeic¸oamento de Pessoal de
N
´
ıvel Superior — CAPES (CAPES – Grant PROAP
88887.842889/2023-00 – PUC/MG, Grant PDPG
88887.708960/2022-00 – PUC/MG - Inform
´
atica,
and Finance Code 001). The present work was also
carried out with the support of Fundac¸
˜
ao de Amparo
`
a Pesquisa do Estado de Minas Gerais (FAPEMIG)
under grant number APQ-01929-22.
REFERENCES
Alonzo, R., Hussain, J., Stranges, S., and Anderson, K. K.
(2021). Interplay between social media use, sleep
quality, and mental health in youth: A systematic re-
view. Sleep Medicine Reviews, 56:101414.
Buysse, D. J., Reynolds III, C. F., Monk, T. H., Berman,
S. R., and Kupfer, D. J. (1989). The pittsburgh sleep
quality index: a new instrument for psychiatric prac-
tice and research. Psychiatry research, 28(2):193–
213.
Carr, C. T. and Hayes, R. A. (2015). Social media: Defin-
ing, developing, and divining. Atlantic Journal of
Communication, 23(1):46–65.
Coutinho, L., Song, M., and Z
´
arate, L. (2024). Longitudi-
nal data analysis based on triadic rules to describe of
the psychological reactions during covid 19 pandemic.
In 17th International Joint Conference on Biomedi-
cal Engineering Systems and Technologies, volume 2,
pages 323–329.
Exelmans, L. and Van den Bulck, J. (2016). Bedtime mo-
bile phone use and sleep in adults. Social Science and
Medicine, 148:93–101.
Ganter, B. and Wille, R. (2012). Formal concept analysis:
mathematical foundations. Springer Science & Busi-
ness Media.
Hirshkowitz, M., Whiton, K., Albert, S. M., Alessi, C.,
Bruni, O., DonCarlos, L., Hazen, N., Herman, J.,
Katz, E. S., Kheirandish-Gozal, L., Neubauer, D. N.,
O’Donnell, A. E., Ohayon, M., Peever, J., Rawd-
ing, R., Sachdeva, R. C., Setters, B., Vitiello, M. V.,
Ware, J. C., and Adams Hillard, P. J. (2015). Na-
tional sleep foundation’s sleep time duration recom-
mendations: methodology and results summary. Sleep
Health, 1(1):40–43.
Islam, M. R., Tushar, M. I., Jannath, S., Moona, A. A., Ak-
ter, S., and Islam, S. M. A. (2021). Data set concerning
the use of social networking sites and mental health
problems among the young generation in bangladesh.
Data in Brief, 39:107593.
Kumar, P. M., Udaya Kumar, V., Meenakshi, S., Bahekar,
T. N., Narapaka, P. K., Dhingra, S., and Murti, K.
(2024). Chapter 1 - epidemiology and risk factors of
mental disorders. In Bagchi, D., Chaurasia, R. N., and
Ohia, S. E., editors, A Review on Diverse Neurological
Disorders, pages 3–12. Academic Press.
Kuss, D. J. and Griffiths, M. D. (2017). Social network-
ing sites and addiction: Ten lessons learned. Interna-
tional Journal of Environmental Research and Public
Health, 14(3).
Lana, P., Nobre, C., Zarate, L., and Song, M. (2022). For-
mal concept analysis applied to a longitudinal study
of covid-19. Scitepress.
Lehmann, F. and Wille, R. (1995). A triadic approach
to formal concept analysis. In Conceptual Struc-
tures: Applications, Implementation and Theory:
Third International Conference on Conceptual Struc-
tures, ICCS’95 Santa Cruz, CA, USA, August 14–18,
1995 Proceedings 3, pages 32–43. Springer.
Marttila, E., Koivula, A., and R
¨
as
¨
anen, P. (2021). Does
excessive social media use decrease subjective well-
being? a longitudinal analysis of the relationship be-
tween problematic use, loneliness and life satisfaction.
Telematics and Informatics, 59:101556.
Missaoui, R. and Emamirad, K. (2017). Lattice miner-a
formal concept analysis tool. In 14th International
Conference on Formal Concept Analysis, volume 3,
pages 2–5.
Orzeł-Gryglewska, J. (2010). Consequences of sleep de-
privation. International Journal of Occupational
Medicine and Environmental Health, 23(1):95 – 114.
O’Day, E. B. and Heimberg, R. G. (2021). Social media use,
social anxiety, and loneliness: A systematic review.
Computers in Human Behavior Reports, 3:100070.
Poelmans, J., Ignatov, D. I., Kuznetsov, S. O., and Dedene,
G. (2013). Formal concept analysis in knowledge pro-
cessing: A survey on applications. Expert Systems
with Applications, 40(16):6538–6560.
Russell, D. W. (1996). Ucla loneliness scale (version 3):
Reliability, validity, and factor structure. Journal of
personality assessment, 66(1):20–40.
Song, H., Zmyslinski-Seelig, A., Kim, J., Drent, A., Victor,
A., Omori, K., and Allen, M. (2014). Does facebook
make you lonely?: A meta analysis. Computers in
Human Behavior, 36:446–452.
Song, M. A. J., Galvez, L. E. Z., and Santos, D. S. (2024).
Applying formal concept analysis to characterize in-
fant mortality: Aplicando an
´
alise de formal de con-
ceitos para a caracterizac¸
˜
ao da mortalidade infantil.
Concilium, 24(3):387–407.
Wille, R. (1995). The basic theorem of triadic concept anal-
ysis. Order, 12:149–158.
Wille, R. (2009). Restructuring lattice theory: An approach
based on hierarchies of concepts. In Proceedings of
the 7th International Conference on Formal Concept
Analysis, ICFCA ’09, page 314–339, Berlin, Heidel-
berg. Springer-Verlag.
ˇ
Skopljanac Ma
ˇ
cina, F. and Bla
ˇ
skovi
´
c, B. (2014). Formal
concept analysis – overview and applications. Proce-
dia Engineering, 69:1258–1267. 24th DAAAM Inter-
national Symposium on Intelligent Manufacturing and
Automation, 2013.
Exploring Links Between Social Media Habits, Loneliness, and Sleep: A Formal Concept Analysis Approach
233