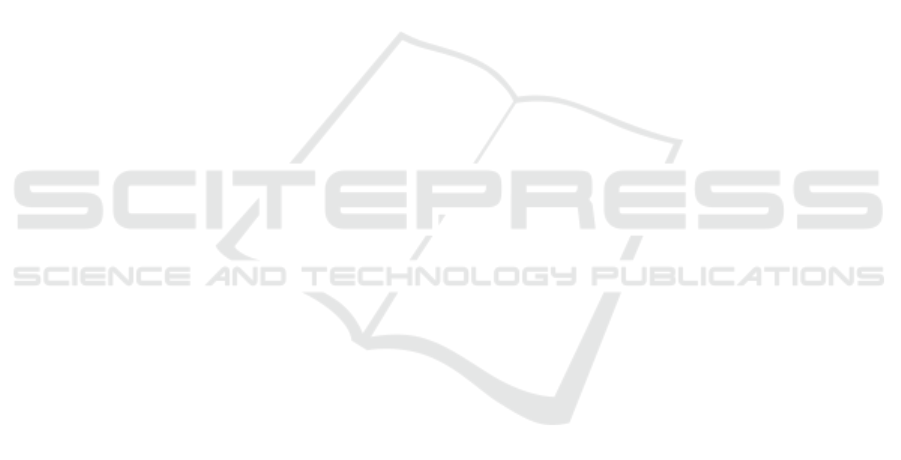
proofs far more quickly. This efficiency is criti-
cal for devices with limited computational power,
where prolonged proof generation can lead to de-
lays and increased energy consumption, negatively
impacting battery life and operational sustainability.
zk-STARK, by contrast, exhibits higher proof gen-
eration times due to its computational complexity,
making it less suited for resource-constrained scenar-
ios. In terms of verification time, Bulletproof excels
in both single-request and concurrent-request scenar-
ios, making it suitable for real-time applications re-
quiring low-latency performance. zk-STARK, while
providing stronger security guarantees, incurs longer
verification times, which limits applicability in time-
sensitive environments. Bulletproof’s ability to han-
dle concurrent verification requests further highlights
its robustness and practicality for high-load applica-
tions. zk-STARK’s longer verification times under
such conditions indicate potential bottlenecks in sce-
narios requiring rapid and simultaneous proof veri-
fications. While zk-STARK’s scalability and post-
quantum security provide valuable long-term benefits,
these come at the cost of higher computational and
storage requirements, reducing its practicality for IoT
applications. Overall, Bulletproof is a more feasible
choice for resource-constrained IoT systems, balanc-
ing efficiency and security effectively. Its lower proof
generation and verification times, coupled with com-
pact proof sizes, make it better for real-time and low-
power applications. zk-STARK is suited for scenarios
prioritizing robust security over performance.
5 CONCLUSIONS
This study evaluated the performance of Bulletproof
and zk-STARK zero-knowledge proof systems in dis-
tributed, decentralized, and resource-constrained en-
vironments, focusing on proof generation time, veri-
fication time, and proof size. The results show that
Bulletproof consistently outperforms zk-STARK in
terms of efficiency, producing faster proofs, smaller
proof sizes, and shorter verification times, making
it a practical choice for real-time, energy-efficient,
and bandwidth-limited IoT applications. While zk-
STARK provides robust security and scalability, its
higher resource demands limit its suitability for con-
strained settings. Future work could explore hy-
brid approaches that combine both protocols and
investigate optimizations (El Ioini and Pahl, 2018)
to enhance zk-STARK’s performance in resource-
constrained environments.
REFERENCES
Androulaki, E., Barger, A., Bortnikov, V., Cachin, C.,
Christidis, K., De Caro, A., Enyeart, D., Ferris, C.,
Laventman, G., Manevich, Y., et al. (2018). Hyper-
ledger fabric: a distributed operating system for per-
missioned blockchains. In EuroSys, pages 1–15.
Atzori, L., Iera, A., and Morabito, G. (2010). The internet
of things: A survey. Comp Netw, 54(15):2787–2805.
Ben-Sasson, E., Bentov, I., Horesh, Y., and Riabzev, M.
(2018). Scalable, transparent, and post-quantum se-
cure computational integrity. Crypt ePrint Arch.
Ben-Sasson, E., Chiesa, A., Tromer, E., and Virza, M.
(2017). Scalable zero knowledge via cycles of elliptic
curves. Algorithmica, 79:1102–1160.
Berenjestanaki, M. H., Barzegar, H. R., El Ioini, N., and
Pahl, C. (2023). Blockchain-based e-voting systems:
a technology review. Electronics, 13(1):17.
B
¨
unz, B., Bootle, J., Boneh, D., Poelstra, A., Wuille, P., and
Maxwell, G. (2018). Bulletproofs: Short proofs for
confidential transactions and more. In Sym Sec&Priv.
El Ioini, N. and Pahl, C. (2018). Trustworthy orchestra-
tion of container based edge computing using permis-
sioned blockchain. In 2018 Fifth International Con-
ference on Internet of Things: Systems, Management
and Security, pages 147–154.
Goldreich, O. and Oren, Y. (1994). Definitions and prop-
erties of zero-knowledge proof systems. Journal of
Cryptology, 7(1):1–32.
Goldwasser, S., Micali, S., and Rackoff, C. (2019). The
knowledge complexity of interactive proof-systems.
In Providing sound foundations for cryptography.
Mukherjee, M., Matam, R., Shu, L., Maglaras, L., Ferrag,
M. A., Choudhury, N., and Kumar, V. (2017). Secu-
rity and privacy in fog computing: Challenges. IEEE
Access, 5:19293–19304.
Narula, N., Vasquez, W., and Virza, M. (2018).
{zkLedger}:{Privacy-Preserving} auditing for dis-
tributed ledgers. In Symposium on networked systems
design and implementation.
Pahl, C. and El Ioini, N. (2019). Blockchain based service
continuity in mobile edge computing. In IOTSMS,
pages 136–141.
Roman, R., Zhou, J., and Lopez, J. (2013). On the features
and challenges of security and privacy in distributed
internet of things. Comp Netw, 57(10):2266–2279.
Werth, J., Berenjestanaki, M. H., Barzegar, H. R., El Ioini,
N., and Pahl, C. (2023a). A review of blockchain plat-
forms based on the scalability, security and decentral-
ization trilemma.
Werth, J., El Ioini, N., Berenjestanaki, M. H., Barze-
gar, H. R., and Pahl, C. (2023b). A platform se-
lection framework for blockchain-based software sys-
tems based on the blockchain trilemma.
Yang, Y., Wu, L., Yin, G., Li, L., and Zhao, H. (2017). A
survey on security and privacy issues in internet-of-
things. IEEE Internet of things Jrnl, 4(5):1250–1258.
IoTBDS 2025 - 10th International Conference on Internet of Things, Big Data and Security
244