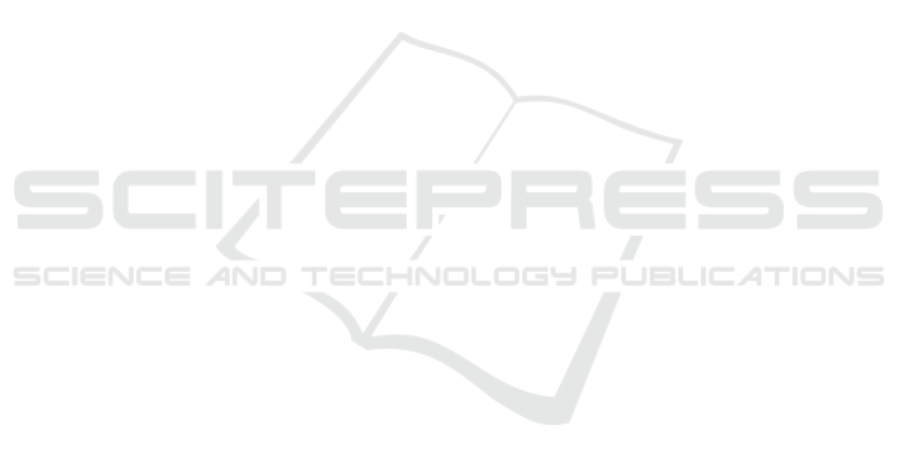
Security Concerns: While blockchain and ZKP en-
hance security, they are not immune to all attacks. Po-
tential vulnerabilities in cryptographic algorithms can
be exploited, and ensuring robust security protocols is
essential (Sun et al., 2021).
Cost of Implementation: The cost associated with
implementing blockchain and ZKP technologies can
be prohibitive. This includesfinancial costs but also
time and resources required for development, deploy-
ment, and maintenance (Derei et al., 2023).
5 CONCLUSIONS
We explored the foundational concepts and develop-
ments in zero-knowledge proofs (ZKPs), a crypto-
graphic technique that ensures the validity of a state-
ment without revealing any additional information.
We provided 1. Introduction to Zero-Knowledge
Proofs as an overview of ZKPs, including their ori-
gin and fundamental principles. 2. Types of Zero-
Knowledge Proofs providing a differentiation exam-
ination of the different types of ZKPs, including in-
teractive and non-interactive proofs. 3. Classifica-
tion of Common Protocols based on Defined Assess-
ment criteria - following a systematic selection pro-
cess. 4. Advanced Protocols like zkSNARKs, zk-
STARKs, Bulletproofs, comparing their mechanisms,
advantages, and limitations.
Our review shows that decentralisation or resource
limitations create challenges such as scalability, com-
putational overhead, privacy and data integrity, wider
security concerns and cost to be addressed.
REFERENCES
Alkhamisi, A. O. and Alboraei, F. (2019). Privacy-aware
decentralized and scalable access control management
for iot environment. Jrnl of King Abdulaziz Univ
Comp and Inf Tech Sci, 8(1):71–84.
Ames, S., Hazay, C., Ishai, Y., and Venkitasubramaniam,
M. (2017). Ligero: Lightweight sublinear arguments
without a trusted setup. In Conf Comp & Comm Sec.
Atzori, L., Iera, A., and Morabito, G. (2010). The internet
of things: A survey. Comp Netw, 54(15):2787–2805.
Ben-Sasson, E., Bentov, I., Horesh, Y., and Riabzev, M.
(2018). Scalable, transparent, and post-quantum se-
cure computational integrity. Crypt ePrint Arch.
Ben-Sasson, E., Chiesa, A., Tromer, E., and Virza, M.
(2014). Succinct {Non-Interactive} zero knowledge
for a von neumann architecture. In USENIX.
Berenjestanaki, M. H., Barzegar, H. R., El Ioini, N., and
Pahl, C. (2023). Blockchain-based e-voting systems:
a technology review. Electronics, 13(1):17.
Boyar, J., Friedl, K., and Lund, C. (1991). Practical zero-
knowledge proofs: Giving hints and using deficien-
cies. Journal of cryptology, 4:185–206.
B
¨
unz, B., Bootle, J., Boneh, D., Poelstra, A., Wuille, P., and
Maxwell, G. (2018). Bulletproofs: Short proofs for
confidential transactions and more. In Sym Sec&Priv.
Derei, T., Aulenbach, B., Carolino, V., Geren, C., Kauf-
man, M., Klein, J., Islam Shanto, R., and Korth,
H. F. (2023). Scaling zero-knowledge to verifiable
databases. In Workshop on Verifiable DBS.
El Ioini, N. and Pahl, C. (2018). Trustworthy orchestra-
tion of container based edge computing using permis-
sioned blockchain. In 2018 Fifth International Con-
ference on Internet of Things: Systems, Management
and Security, pages 147–154.
Fiat, A. and Shamir, A. (1986). How to prove your-
self: Practical solutions to identification and signature
problems. In Theory and Appl of cryptographic Tech.
Fiege, U., Fiat, A., and Shamir, A. (1987). Zero knowledge
proofs of identity. In Symp on Theory of computing.
Gabizon, A., Williamson, Z. J., and Ciobotaru, O. (2019).
Plonk: Permutations over lagrange-bases for oe-
cumenical noninteractive arguments of knowledge.
Cryptology ePrint Archive.
Giacomelli, I., Madsen, J., and Orlandi, C. (2016).
{ZKBoo}: Faster {Zero-Knowledge} for boolean cir-
cuits. In USENIX.
Goldreich, O. and Oren, Y. (1994). Definitions and prop-
erties of zero-knowledge proof systems. Journal of
Cryptology, 7(1):1–32.
Goldwasser, S., Micali, S., and Rackoff, C. (2019). The
knowledge complexity of interactive proof-systems.
In Providing sound foundations for cryptography.
Groth, J. and Sahai, A. (2008). Efficient non-interactive
proof systems for bilinear groups. In EUROCRYPT.
Guillou, L. C. and Quisquater, J.-J. (1988). A practical zero-
knowledge protocol fitted to security microprocessor
minimizing both transmission and memory. In EU-
ROCRYPT, pages 123–128. Springer.
Jedlicka, J. and Grant, E. S. (2022). Data privacy through
zero-knowledge proofs. In ICERECT.
Lin, X., Zhang, Y., Huang, C., Xing, B., Chen, L., Hu, D.,
and Chen, Y. (2023). An access control system based
on blockchain with zero-knowledge rollups in high-
traffic iot environments. Sensors, 23(7):3443.
Mukherjee, M., Matam, R., Shu, L., Maglaras, L., Ferrag,
M. A., Choudhury, N., and Kumar, V. (2017). Secu-
rity and privacy in fog computing: Challenges. IEEE
Access, 5:19293–19304.
Pahl, C. and El Ioini, N. (2019). Blockchain based service
continuity in mobile edge computing. In IOTSMS,
pages 136–141.
Roman, R., Zhou, J., and Lopez, J. (2013). On the features
and challenges of security and privacy in distributed
internet of things. Comp Netw, 57(10):2266–2279.
Schnorr, C.-P. (1990). Efficient identification and signatures
for smart cards. In CRYPTO.
Song, L., Ju, X., Zhu, Z., and Li, M. (2021). An access
control model for the internet of things based on zero-
IoTBDS 2025 - 10th International Conference on Internet of Things, Big Data and Security
252