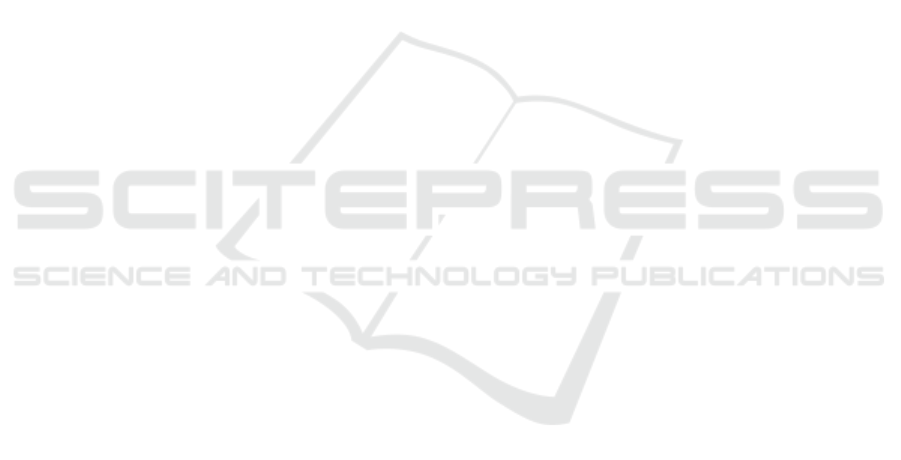
In the experiment, the proposed method was applied
to three large-scale event logs, and it was demon-
strated that effective parameter combinations could be
obtained without excess or deficiency.
In this study, we focused on only two of the pa-
rameters used in HeuristicsMiner for model identi-
fication. Adapting this approach to other discovery
techniques and more parameters would make the pro-
cess discovery more efficient. There are many other
parameter-based process discovery techniques such as
Fuzzy Miner (G
¨
unther and van der Aalst, 2007), each
with different characteristics and analytical capabil-
ities. In our future work, we would like to support
other techniques in this way so that users can use more
algorithms with little knowledge of process discovery
and are more likely to encounter models that more ac-
curately reflect their business flow.
It is also necessary to consider a method for se-
lecting ideal process models from the set of models
obtained from the input logs identified by the pro-
posed method. The experiment has shown that this
method can significantly reduce the number of can-
didate models. However, when the input logs are
large and the process model transition table contains
many cells, it is still difficult to find the model that the
user wants from the set of models generated by this
method. A possible method to assist users in select-
ing a process model is to use metrics such as precision
rate and recall rate to further narrow down the candi-
date models, and the specific procedure for this needs
to be studied. In addition, if a tool with an interac-
tive interface can be developed to present the model
the user is seeking in a question-and-answer format,
it will be easier to obtain a process model. In this
way, it is important to develop tools that can be easily
handled even by users without knowledge of process
discovery, in order to popularize process mining.
REFERENCES
Augusto, A., Dumas, M., La Rosa, M., Leemans, S. J. J.,
and vanden Broucke, S. K. L. M. (2021). Optimiza-
tion framework for dfg-based automated process dis-
covery approaches. Software and Systems Modeling,
20:1245–1270.
Burattin, A. (2015). Heuristics Miner for Time Inter-
val, pages 85–95. Springer International Publishing,
Cham.
Burattin, A. and Sperduti, A. (2010). Automatic determina-
tion of parameters’ values for heuristics miner++. In
IEEE Congress on Evolutionary Computation, pages
1–8.
G
¨
unther, C. W. and van der Aalst, W. M. P. (2007).
Fuzzy mining – adaptive process simplification based
on multi-perspective metrics. In Business Process
Management, pages 328–343, Berlin, Heidelberg.
Springer Berlin Heidelberg.
Keith, B. and Vega, V. (2017). Process mining applications
in software engineering. In Trends and Applications in
Software Engineering, pages 47–56, Cham. Springer
International Publishing.
Mans, R., Schonenberg, H., Leonardi, G., Panzarasa, S.,
Cavallini, A., Quaglini, S., and van der Aalst, W.
(2008). Process mining techniques: An application
to stroke care. Studies in health technology and infor-
matics, 136:573–578.
Medeiros, A., Dongen, B., van der Aalst, W., and Wei-
jters, A. (2004). Process mining for ubiquitous mo-
bile systems: An overview and a concrete algorithm.
In Ubiquitous Mobile Information and Collaboration
Systems, volume 3272, pages 151–165.
Montasser, R. K. and Helal, I. M. A. (2023). Process discov-
ery automation: Benefits and limitations. In 2023 In-
telligent Methods, Systems, and Applications (IMSA),
pages 496–501.
PDC2023 (2023). Process discovery contest
2023. https://icpmconference.org/2023/
process-discovery-contest/.
ProM (2023). Prom tools. https://promtools.org/
prom-6-13/.
Rozinat, A., Jong, I., Gunther, C., and van der Aalst, W.
(2009). Process mining applied to the test process of
wafer scanners in asml. Systems, Man, and Cybernet-
ics, Part C: Applications and Reviews, IEEE Transac-
tions on, 39:474–479.
van der Aalst, W. (2016). Process Mining: Data Science in
Action. Springer Berlin, Heidelberg.
van der Aalst, W., Weijters, T., and Maruster, L. (2004).
Workflow mining: discovering process models from
event logs. IEEE Transactions on Knowledge and
Data Engineering, 16(9):1128–1142.
Weijters, A. and Ribeiro, J. (2011). Flexible heuristics
miner (fhm). In Journal of Applied Physiology - J
APPL PHYSIOL, pages 310–317.
Weijters, A., van der Aalst, W., and Alves de Medeiros, A.
(2006). Process mining with the HeuristicsMiner al-
gorithm. BETA publicatie : working papers. Technis-
che Universiteit Eindhoven.
Wen, L., van der Aalst, W., Wang, J., and Sun, J. (2007).
Mining process models with non-free-choice con-
structs. Data Min. Knowl. Discov., 15:145–180.
Wen, L., Wang, J., van der Aalst, W., Huang, B., and Sun, J.
(2010). Mining process models with prime invisible
tasks. Data & Knowledge Engineering, 69:999–1021.
ENASE 2025 - 20th International Conference on Evaluation of Novel Approaches to Software Engineering
456