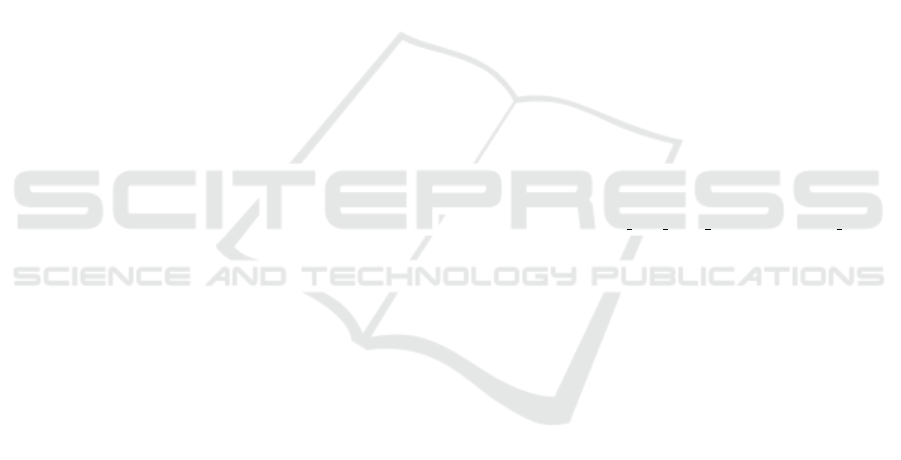
Aw, A. and Rascle, M. (2000). Resurrection of” second
order” models of traffic flow. SIAM journal on applied
mathematics, 60(3):916–938.
Brederode, L., Pel, A., Wismans, L., de Romph, E., and
Hoogendoorn, S. (2019). Static traffic assignment
with queuing: model properties and applications.
Transportmetrica A: Transport Science, 15(2):179–
214.
Contreras, S., Agarwal, S., and Kachroo, P. (2017). Quality
of traffic observability on highways with lagrangian
sensors. IEEE Transactions on Automation Science
and Engineering, 15(2):761–771.
Fafoutellis, P. and Vlahogianni, E. I. (2023). Unlocking
the full potential of deep learning in traffic forecast-
ing through road network representations: A critical
review. Data Science for Transportation, 5(3):23.
Frederix, R., Viti, F., Corthout, R., and Tamp
`
ere, C. M.
(2011). New gradient approximation method for dy-
namic origin–destination matrix estimation on con-
gested networks. Transportation Research Record,
2263(1):19–25.
Goodfellow, I., Pouget-Abadie, J., Mirza, M., Xu, B.,
Warde-Farley, D., Ozair, S., Courville, A., and Ben-
gio, Y. (2014). Generative adversarial nets. Advances
in neural information processing systems, 27.
He, Y., An, C., Jia, Y., Liu, J., Lu, Z., and Xia, J. (2024).
Efficient and robust freeway traffic speed estimation
under oblique grid using vehicle trajectory data. IEEE
Transactions on Intelligent Transportation Systems.
Hochreiter, S. (1997). Long short-term memory. Neural
Computation MIT-Press.
Jamil, A. R. M., Ganguly, K. K., and Nower, N. (2022). An
experimental analysis of reward functions for adaptive
traffic signal control system. In Distributed Sensing
and Intelligent Systems: Proceedings of ICDSIS 2020,
pages 513–523. Springer.
Jin, D., Shi, J., Wang, R., Li, Y., Huang, Y., and Yang, Y.-B.
(2023a). Trafformer: unify time and space in traffic
prediction. In Proceedings of the AAAI Conference on
Artificial Intelligence, volume 37, pages 8114–8122.
Jin, G., Liu, L., Li, F., and Huang, J. (2023b). Spatio-
temporal graph neural point process for traffic con-
gestion event prediction. In Proceedings of the AAAI
Conference on Artificial Intelligence, volume 37,
pages 14268–14276.
Kamarianakis, Y. and Prastacos, P. (2003). Forecasting traf-
fic flow conditions in an urban network: Compari-
son of multivariate and univariate approaches. Trans-
portation Research Record, 1857(1):74–84.
Kim, Y., Bang, H., et al. (2018). Introduction to kalman
filter and its applications. Introduction and Implemen-
tations of the Kalman Filter, 1:1–16.
Kucharski, R., Kostic, B., and Gentile, G. (2017). Real-
time traffic forecasting with recent dta methods. In
2017 5th IEEE International Conference on Models
and Technologies for Intelligent Transportation Sys-
tems (MT-ITS), pages 474–479. IEEE.
Lee, S. and Fambro, D. B. (1999). Application of subset
autoregressive integrated moving average model for
short-term freeway traffic volume forecasting. Trans-
portation research record, 1678(1):179–188.
Li, Y., Yu, R., Shahabi, C., and Liu, Y. (2017). Diffusion
convolutional recurrent neural network: Data-driven
traffic forecasting. arXiv preprint arXiv:1707.01926.
Ma, X., Dai, Z., He, Z., Ma, J., Wang, Y., and Wang, Y.
(2017). Learning traffic as images: A deep convo-
lutional neural network for large-scale transportation
network speed prediction. sensors, 17(4):818.
Payne, H. J. (1971). Model of freeway traffic and control.
Mathematical Model of Public System, pages 51–61.
Ren, H., Deng, J., Zhang, B., Ye, Z., and Fu, X. (2022).
A breadth-first search algorithm accelerator based on
csci graph data format. pages 636–640.
Sun, C., Guanetti, J., Borrelli, F., and Moura, S. J. (2020).
Optimal eco-driving control of connected and au-
tonomous vehicles through signalized intersections.
IEEE Internet of Things Journal, 7(5):3759–3773.
Tamp
`
ere, C. M., Corthout, R., Cattrysse, D., and Immers,
L. H. (2011). A generic class of first order node
models for dynamic macroscopic simulation of traffic
flows. Transportation Research Part B: Methodologi-
cal, 45(1):289–309.
Tay, L., Lim, J. M.-Y., Liang, S.-N., Keong, C. K., and
Tay, Y. H. (2023). Urban traffic volume estimation
using intelligent transportation system crowdsourced
data. Engineering Applications of Artificial Intelli-
gence, 126:107064.
Telraam (2023). Telraam. https://telraam.net/. Accessed:
2023.
Vanderhoydonc et al. (2018). Strategische verkeersmod-
ellen vlaanderen versie 4.1.1 – overzichtsrap-
portage. https://assets.vlaanderen.be/image/upload/
v1625826383/1
spm 4.1.1 overzichtsrapport tusvju.
pdf.
Vlaams Verkeerscentrum (2023). Vlaams verkeerscentrum.
http://www.verkeerscentrum.be. Accessed: 2023.
Wang, R., Fan, S., and Work, D. B. (2016). Efficient mul-
tiple model particle filtering for joint traffic state es-
timation and incident detection. Transportation Re-
search Part C: Emerging Technologies, 71:521–537.
Williams, B. M. (2001). Multivariate vehicular traffic flow
prediction: evaluation of arimax modeling. Trans-
portation Research Record, 1776(1):194–200.
Wu, Z., Pan, S., Long, G., Jiang, J., Chang, X., and Zhang,
C. (2020). Connecting the dots: Multivariate time se-
ries forecasting with graph neural networks. In Pro-
ceedings of the 26th ACM SIGKDD international con-
ference on knowledge discovery & data mining, pages
753–763.
Yan, Y., Cui, S., Liu, J., Zhao, Y., Zhou, B., and Kuo, Y.-
H. (2025). Multimodal fusion for large-scale traffic
prediction with heterogeneous retentive networks. In-
formation Fusion, 114:102695.
Zhan, X., Zheng, Y., Yi, X., and Ukkusuri, S. V. (2016).
Citywide traffic volume estimation using trajectory
data. IEEE Transactions on Knowledge and Data En-
gineering, 29(2):272–285.
Zhang, J., Mao, S., Yang, L., Ma, W., Li, S., and Gao,
Z. (2024). Physics-informed deep learning for traf-
fic state estimation based on the traffic flow model
and computational graph method. Information Fusion,
101:101971.
VEHITS 2025 - 11th International Conference on Vehicle Technology and Intelligent Transport Systems
430