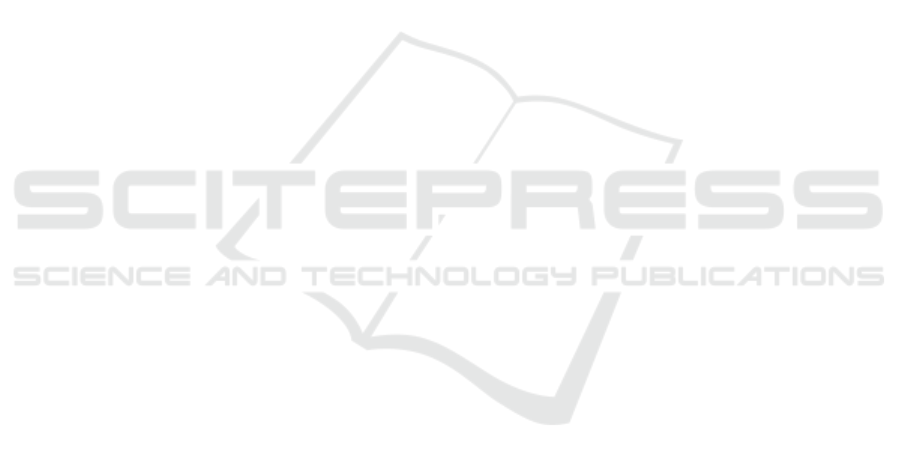
tative variables. Additional metacognitive strategies,
such as the Monitoring strategy—where students seek
clarification on unclear concepts—will also be ex-
amined. Moreover, investigating the relationship be-
tween student profiles, GenAI metacognitive strate-
gies, and learning outcomes is essential.
ACKNOWLEDGMENT
This work was supported by grants PID2023-
146692OB-C31 (GENIELearn project) and PID2020-
112584RB-C31 (H2O Learn project) funded by MI-
CIU / AEI / 10.13039 / 501100011033, MCIN / AEI
/ 10.13039 / 501100011033 and ERDF, EU. Further
support has been received from the UNESCO Chair
“Scalable Digital Education for All” at UC3M.
REFERENCES
Alario-Hoyos, C., Kemcha, R., Kloos, C. D., Callejo, P.,
Est
´
evez-Ayres, I., Sant
´
ın-Crist
´
obal, D., Cruz-Argudo,
F., and L
´
opez-S
´
anchez, J. L. (2024). Tailoring your
code companion: Leveraging llms and rag to develop
a chatbot to support students in a programming course.
In 2024 IEEE International Conference on Teaching,
Assessment and Learning for Engineering (TALE),
pages 1–8.
Anthropic (2023). Claude: Next-generation ai assistant.
Accessed: January 16, 2025.
Bastani, H., Bastani, O., Sungu, A., Ge, H.,
¨
Ozge Kabakcı,
and Mariman, R. (2024). Generative ai can harm
learning. Technical report, The Wharton School Re-
search Paper.
Callejo, P., Alario-Hoyos, C., and Delgado-Kloos, C.
(2024). Evaluating the impact of chatgpt on program-
ming learning outcomes in a big data course. Interna-
tional Journal of Engineering Education, 40(4):863–
872.
Carvalho, P. F., Sana, F., and Yan, V. X. (2020). Self-
regulated spacing in a massive open online course is
related to better learning. npj Science of Learning,
5(1):2.
Catal
´
an, A. C., Gonz
´
alez-Castro, N., Delgado, K. C.,
Alario-Hoyos, C., and Mu
˜
noz-Merino, P. J. (2021).
Conversational agent for supporting learners on a
mooc on programming with java. Computer Science
and Information Systems, 18(4):1271–1286.
Chan, C. K. Y. and Hu, W. (2023). Students’ voices on
generative ai: Perceptions, benefits, and challenges
in higher education. International Journal of Educa-
tional Technology in Higher Education, 20(1):43.
Chen, J., Lin, H., Han, X., and Sun, L. (2024). Benchmark-
ing large language models in retrieval-augmented gen-
eration. Proceedings of the AAAI Conference on Arti-
ficial Intelligence, 38(16):17754–17762.
Dickey, E., Bejarano, A., and Garg, C. (2024). Ai-lab: A
framework for introducing generative artificial intel-
ligence tools in computer programming courses. SN
Comput. Sci., 5(6).
Firth, J., Rivers, I., and Boyle, J. (2021). A systematic re-
view of interleaving as a concept learning strategy. Re-
view of Education, 9(2):642–684.
Flavell, J. H. (1979). Metacognition and cognitive monitor-
ing: A new area of cognitive–developmental inquiry.
American psychologist, 34(10):906.
Ghimire, A. and Edwards, J. (2024). Coding with ai: How
are tools like chatgpt being used by students in foun-
dational programming courses. In Olney, A. M.,
Chounta, I.-A., Liu, Z., Santos, O. C., and Bittencourt,
I. I., editors, Artificial Intelligence in Education, pages
259–267, Cham. Springer Nature Switzerland.
Larsen, D. P. (2018). Planning education for long-term
retention: the cognitive science and implementation
of retrieval practice. In Seminars in neurology, vol-
ume 38, pages 449–456. Thieme Medical Publishers.
Lo, C. K., Hew, K. F., and yung Jong, M. S. (2024). The
influence of chatgpt on student engagement: A sys-
tematic review and future research agenda. Computers
and Education, 219:105100.
Margulieux, L. E., Prather, J., Reeves, B. N., Becker, B. A.,
Cetin Uzun, G., Loksa, D., Leinonen, J., and Denny,
P. (2024). Self-regulation, self-efficacy, and fear of
failure interactions with how novices use llms to solve
programming problems. In Proceedings of the 2024
on Innovation and Technology in Computer Science
Education V. 1, ITiCSE 2024, page 276–282, New
York, NY, USA. Association for Computing Machin-
ery.
Neo, M. (2022). The merlin project: Malaysian stu-
dents’acceptance of an ai chatbot in their learning pro-
cess. Turkish Online Journal of Distance Education,
23(3):31–48.
Puryear, B. and Sprint, G. (2022). Github copilot in the
classroom: learning to code with ai assistance. J.
Comput. Sci. Coll., 38(1):37–47.
Sun, D., Boudouaia, A., Zhu, C., and Li, Y. (2024). Would
chatgpt-facilitated programming mode impact college
students’ programming behaviors, performances, and
perceptions? an empirical study. International Jour-
nal of Educational Technology in Higher Education,
21(1):14.
Uguina-Gadella, L., Est
´
evez-Ayres, I., Fisteus, J. A.,
Alario-Hoyos, C., and Kloos, C. D. (2024). Anal-
ysis and prediction of students’ performance in a
computer-based course through real-time events.
IEEE Transactions on Learning Technologies,
17:1794–1804.
Vargas-Murillo, A. R., de la Asuncion Pari-Bedoya, I.
N. M., and de Jes
´
us Guevara-Soto, F. (2023). Chal-
lenges and opportunities of ai-assisted learning: A
systematic literature review on the impact of chat-
gpt usage in higher education. International Jour-
nal of Learning, Teaching and Educational Research,
22(7):122–135.
Xia, Q., Chiu, T. K. F., Chai, C. S., and Xie, K. (2023).
The mediating effects of needs satisfaction on the rela-
tionships between prior knowledge and self-regulated
learning through artificial intelligence chatbot. British
Journal of Educational Technology, 54(4):967–986.
CSEDU 2025 - 17th International Conference on Computer Supported Education
280