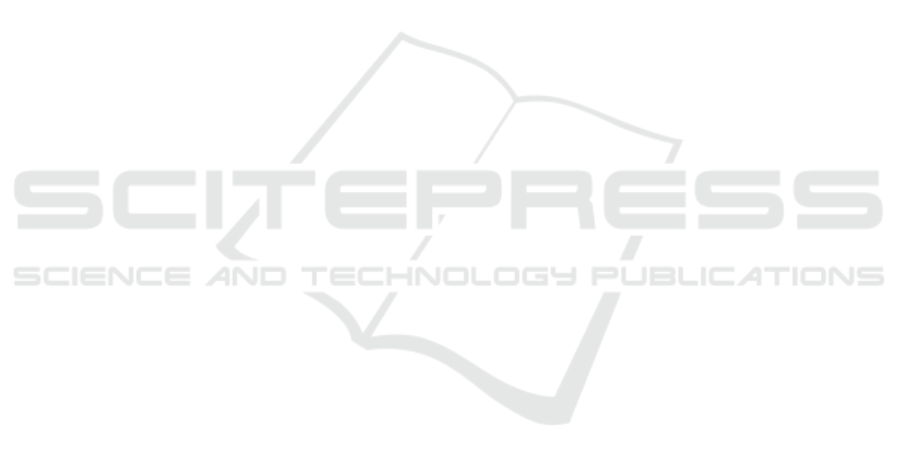
Exploratory Projects Contain...
1. Tutorials/Examples: Software projects that are
primarily created for educational or demonstra-
tion purposes to teach specific programming con-
cepts, techniques, or best practices. These
projects are often small in scope, focused on a par-
ticular feature or functionality, and intended to be
easily understood and replicated by learners.
2. Prototypes: Software projects that are built to
validate an idea, test the feasibility of a concept
or explore potential solutions to a problem. Pro-
totypes are typically not intended for production
use, but rather to gather feedback, identify tech-
nical challenges, and inform the development of a
more polished, production-ready application.
3. Proofs of Concept (PoC): Similar to prototypes,
PoCs are developed to demonstrate the viability of
a particular approach, technology, or solution to a
problem. These projects are often small in scale
and focused on validating a specific hypothesis or
claim rather than building a complete, production-
ready application.
4. School/Training Projects: Software projects cre-
ated as part of educational programs, such as
university courses, coding bootcamps, or internal
training initiatives. These projects focus primar-
ily on the learning process, allowing students to
apply their knowledge and develop practical pro-
gramming skills.
5. Research Projects: Software projects that are
part of research efforts, focused on showcas-
ing novel technologies, algorithms, or techniques
without emphasizing long-term aspects such as
software architecture or intending to make the
system productive.
6. Technology Exploration/Testing: Software
projects developed to experiment with new
technologies, languages, frameworks, or tools,
without a specific production-oriented goal in
mind. These projects are often small-scale,
exploratory in nature and may not have a clear
end-user or business value.
7. Experimental/R&D Projects: Software projects
that are part of research and development efforts,
focused on advancing the state-of-the-art in a par-
ticular domain or exploring innovative solutions
to complex problems. These projects may not
have immediate commercial or practical applica-
tions but are driven by a desire to push the bound-
aries of what is possible with technology.
8. Hobby/Personal Projects: Software projects de-
veloped by individuals for their own personal in-
terest, enjoyment, or learning, without a focus
on commercial or enterprise-level requirements.
These projects are often driven by the creator’s
passion, curiosity, or desire to explore a particu-
lar domain or technology.
4 DATASET
To apply different techniques for classification, we
needed a dataset. Since we did not find related work
or data, which we can use, we crawled, annotated and
analyzed README.md files from software project
repositories on GitHub. In the following we describe
the data collection and annotation process, as well as
giving some insights into this new dataset, which is
publicly available at:
https://github.com/CCWI/sw-repo-
classification-study
In addition to the data, the linked repository pro-
vides the classification source code, including the
prompts and instructed output structure for each ex-
periment.
4.1 Data Collection
Since no existing dataset classifies software repos-
itories in a comparable way, we curated and an-
notated our own. Using the GitHub API, we ex-
tracted README.md files from various repositories,
focusing solely on this common format and exclud-
ing less prevalent formats like README.rst, which
are beyond our study’s scope. The final dataset
comprises 200 randomly selected repositories from
GitHub, each containing at least one README.md
file.
In general, repositories are very heterogeneous in
terms of structure and content. They can contain a
wide variety of files. One of the most important files
in a repository is the README.md file, which typi-
cally provides an overview of the project, its purpose,
how to use it, and other relevant information. It is
often present in the root directory of the repository
and is displayed automatically on repository’s host-
ing platforms, like GitHub and GitLab pages. The
README.md file is written in markdown format. In
some cases, multiple README files may be present
in different directories of the repository to provide in-
formation about standalone or additional software, as
well as software components, which are typically in-
cluded in the main software as submodules.
Another aspect to consider is time. README
files can be changed and updated several times dur-
ing the development of a project. This can result in
Is It Professional or Exploratory? Classifying Repositories Through README Analysis
459