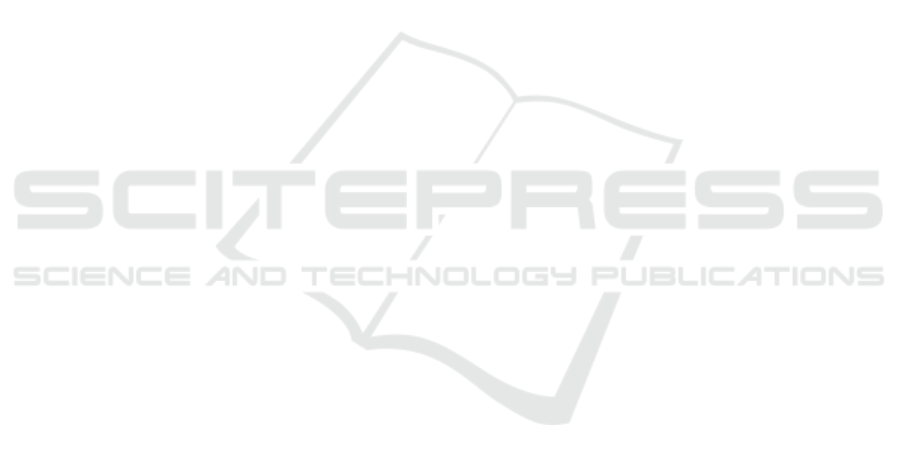
the financial support of the Deutsche Zentrum f
¨
ur
Digitalisierungs- und Technologieforschung der Bun-
deswehr (dtec.bw) under the funding of Europ
¨
aischen
Union – NextGenerationEU. We would like to express
our gratitude to Prof. Dr. Silja Hofmann for their sup-
port and insights throughout the experiment. AI tool
Chat GPT has been used to improve the legibility of
the text.
REFERENCES
Aramrattana, M., Fu, J., and Selpi (2022). Behavioral adap-
tation of drivers when driving among automated vehi-
cles. Journal of Intelligent and Connected Vehicles,
5(3):309–315.
Association for Standardization of Automation and Mea-
suring Systems (ASAM) (2024). ASAM Open-
DRIVE Standard. Available at: https://www.asam.net/
standards/detail/opendrive/.
Bartneck, C., L
¨
utge, C., Wagner, A., and Welsh, S. (2021).
Autonomous Vehicles, page 83–92. Dual Use Sci-
ence and Technology, Ethics and Weapons of Mass
Destruction.
Brown, P., Spronck, P., and Powell, W. (2022). The simu-
lator sickness questionnaire, and the erroneous zero
baseline assumption. Frontiers in Virtual Reality,
3:118.
Bucchiarone, A., Battisti, S., Marconi, A., Maldacea, R.,
and Ponce, D. C. (2020). Autonomous shuttle-as-a-
service (asaas): Challenges, opportunities, and social
implications. IEEE Transactions on Intelligent Trans-
portation Systems, 22(6):3790–3799.
Calvi, A., D’Amico, F., Ferrante, C., and Calcaterra, G.
(2022). A driving simulator study on the effects of
autonomous vehicles on drivers behaviour under car-
following conditions. Human Factors in Transporta-
tion, 60(60):10–54941.
Garus, A., Alonso, B., Alonso Raposo, M., Ciuffo, B., et al.
(2022). Impact of new mobility solutions on travel be-
haviour and its incorporation into travel demand mod-
els. Journal of Advanced Transportation, 2022.
Giese, H. and Klein, F. (2005). Autonomous shuttle system
case study. In Scenarios: Models, Transformations
and Tools: International Workshop, Dagstuhl Castle,
Germany, September 7-12, 2003, Revised Selected Pa-
pers, pages 90–94. Springer.
Gold, C., Naujoks, F., Radlmayr, J., Bellem, H., and
Jarosch, O. (2018). Testing scenarios for human fac-
tors research in level 3 automated vehicles. In Ad-
vances in Human Aspects of Transportation: Proceed-
ings of the AHFE 2017 International Conference on
Human Factors in Transportation, July 17- 21, 2017,
The Westin Bonaventure Hotel, Los Angeles, Califor-
nia, USA 8, pages 551–559. Springer.
Iclodean, C., Cordos, N., and Varga, B. O. (2020). Au-
tonomous shuttle bus for public transportation: A re-
view. Energies, 13(11):2917.
Kennedy, R. S., Lane, N. E., Berbaum, K. S., and Lilien-
thal, M. G. (1993). Simulator sickness questionnaire:
An enhanced method for quantifying simulator sick-
ness. The international journal of aviation psychol-
ogy, 3(3):203–220.
Lee, Y.-C. and Winston, F. (2016). Emotions & driving
in stressful traffic situations. Transportation Research
Part F: Psychology and Behavior, 42:215–226.
Maga
˜
na, V. C., Scherz, W. D., Seepold, R., Madrid, N. M.,
Pa
˜
neda, X. G., and Garcia, R. (2020). The effects of
the driver’s mental state and passenger compartment
conditions on driving performance and driving stress.
Sensors, 20(18):5274.
National Highway Traffic Safety Administration (2017).
Quick facts 2016. Technical Report DOT HS
812 456, U.S. Department of Transportation, Na-
tional Highway Traffic Safety Administration. Avail-
able at: https://crashstats.nhtsa.dot.gov/Api/Public/
Publication/812456.
Santiago-Chaparro, K., DeAmico, M., Bill, A., Chitturi, M.,
and Noyce, D. (2011). Virtual road safety audits using
driving simulators: A framework. In 3rd International
Conference on Road Safety and Simulation.
Sayed, I., Abdelgawad, H., and Said, D. (2022). Study-
ing driving behavior and risk perception: a road safety
perspective in egypt. Journal of Engineering and Ap-
plied Science, 69(1):22.
Tavakoli, A., Lai, N., Balali, V., and Heydarian, A. (2023).
How are drivers’ stress levels and emotions associated
with the driving context? a naturalistic study. Journal
of Transport & Health, 31:101649.
The MathWorks, Inc. (2024a). MATLAB Documenta-
tion. Available at: https://www.mathworks.com/help/
matlab/.
The MathWorks, Inc. (2024b). Simulink Documenta-
tion. Available at: https://www.mathworks.com/help/
simulink/.
Vector Informatik GmbH (2024a). CANoe4SW:
Software-in-the-Loop Testing Platform. Avail-
able at: https://www.vector.com/int/en/products/
products-a-z/software/canoe4sw/.
Vector Informatik GmbH (2024b). DYNA4: Simula-
tion and Virtual Testing Platform. Available at:
https://www.vector.com/int/en/products/products-a-z/
software/dyna4/.
Wang, Y., Zhang, W., Wu, S., and Guo, Y. (2007). Simula-
tors for driving safety study–a literature review. In Vir-
tual Reality: Second International Conference, ICVR
2007, Held as part of HCI International 2007, Bei-
jing, China, July 22-27, 2007. Proceedings 2, pages
584–593. Springer.
World Health Organization (2023a). Global status report on
road safety 2023. Available at: https://www.who.int/
publications/i/item/9789240086517.
World Health Organization (2023b). Global sta-
tus report on road safety 2023. Avail-
able at: https://www.who.int/teams/
social-determinants-of-health/safety-and-mobility/
global-status-report-on-road-safety-2023.
VEHITS 2025 - 11th International Conference on Vehicle Technology and Intelligent Transport Systems
64