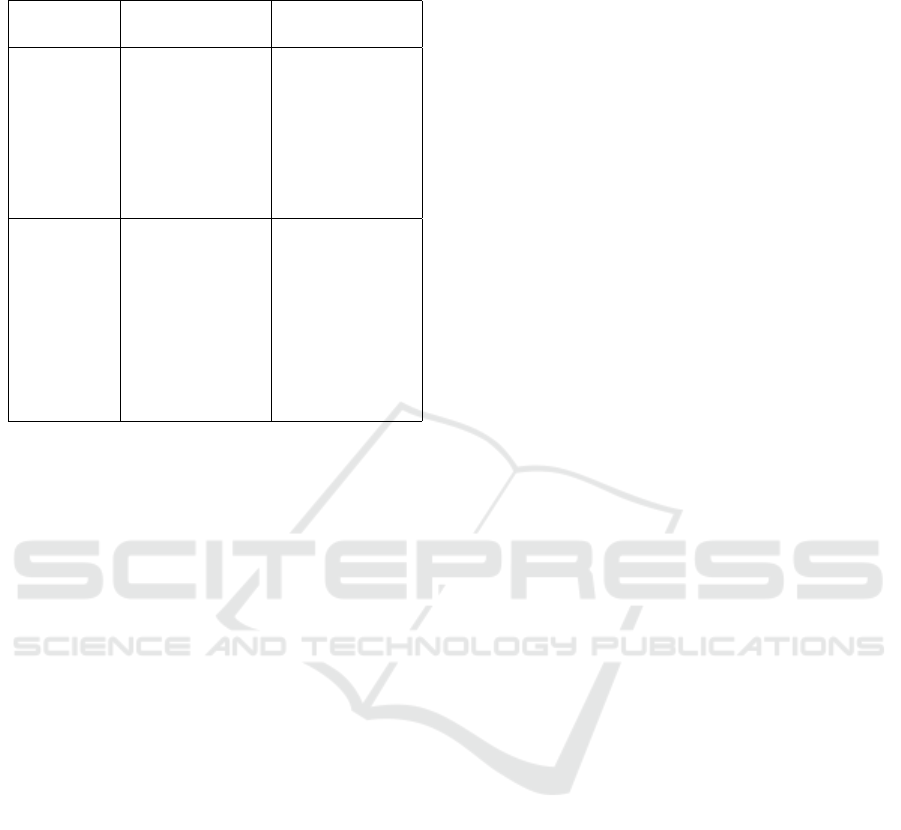
Table 1: Advantages and disadvantages of data summariza-
tion cases.
Data
Summariza-
tion
Advantages Disadvantages
(A) First Case
1.Produces summary for
each event
2.Helps understand
and reconstruct data
with less error
3.Allows for detailed
analysis of the data of
each event
1.Consumes more power
than Second Case
2.Requires more mem-
ory space for storing
multiple summaries
(B) Second Case
1.Creates a single sum-
mary that combines all
events
2.Consumes less power
than First Case (same as
if operating with fixed
minimal data, see Fig. 9)
3.Does not require
additional memory
space
1.Less clarity and under-
standing than First Case
2.May lose some
event-specific details in
the summary
memory, it may lose some specific details of individ-
ual events. Our simulations demonstrated that both
approaches optimize power consumption instead of
relying on incremental data volume. This work con-
tributes to the development of energy-efficient IoT
systems, emphasizing the need to consider all opera-
tional aspects for optimizing device performance and
longevity.
As a future research, we plan to conduct a real-
world experiment to validate the simulation results,
ensuring the practical applicability and accuracy of
our findings. In addition, we aim to investigate the
optimization of power consumption within the con-
text of communication (data transmission and recep-
tion) and peripheral components such as voltage reg-
ulators and real-time clocks (RTC). Notably, our mea-
surements indicate that using communication for data
transfer on our platform consumes less power than di-
rectly connecting and activating sensors for data col-
lection. This finding highlights potential benefits of
sharing sensor data rather than distributing or activat-
ing sensors over the network. Our goal is to identify
and implement specific strategies to further reduce
overall power consumption and costs in IoT appli-
cations, enhancing devices efficiency and extending
battery life.
REFERENCES
Alsharif, M. H., Anabi, H. K., Jahid, J. A., Kannadasan, R.,
Singla, M. K., Gupta, J., and Geem, Z. W. (2024). A
comprehensive survey of energy-efficient computing
to enable sustainable massive iot networks. Alexan-
dria Engineering Journal, 91:12–29.
Baccelli, E., Hahm, O., Petersen, H., and Schleiser, K.
(2015). Riot and the evolution of iot operating sys-
tems and applications. ERCIM News, 2015(101).
Bouguera, T., Diouris, J.-F., Chaillout, J.-J., Jaouadi, R.,
and Andrieux, G. (2018). Energy consumption model
for sensor nodes based on lora and lorawan. Sensors,
18(7):2104.
Brayner, A. and Menezes, R. (2007). Balancing energy con-
sumption and memory usage in sensor data process-
ing. In Proceedings of the 2007 ACM symposium on
Applied computing, pages 935–939.
De Donno, M., Tange, K., and Dragoni, N. (2019). Founda-
tions and evolution of modern computing paradigms:
Cloud, iot, edge, and fog. IEEE access, 7:150936–
150948.
Hammoud, H., Weis, F., Langrume, C., Leclerc, M., and
Bonnin, J.-M. (2024). Monitoring of in-field risk of
infection events by foliar pathogens using smart iot
nodes. In 2024 IEEE International Conferences on
Internet of Things (iThings) and IEEE Green Comput-
ing & Communications (GreenCom) and IEEE Cyber,
Physical & Social Computing (CPSCom) and IEEE
Smart Data (SmartData) and IEEE Congress on Cy-
bermatics, pages 324–330. IEEE.
Jacob, A. M., Rao, S. V., and Pillai, S. S. (2016). Re-
ducing energy consumption by cross layer design in
wireless sensor networks. In 2016 International Con-
ference on Control, Instrumentation, Communication
and Computational Technologies (ICCICCT), pages
93–97. IEEE.
Krishnamurthi, R., Kumar, A., Gopinathan, D., Nayyar, A.,
and Qureshi, B. (2020). An overview of iot sensor data
processing, fusion, and analysis techniques. Sensors,
20(21):6076.
Li, Y., Zhou, Z., Xue, X., Zhao, D., and Hung, P. C. (2023).
Accurate anomaly detection with energy efficiency in
iot–edge–cloud collaborative networks. IEEE Internet
of Things Journal, 10(19):16959–16974.
Lin, W., Shi, F., Wu, W., Li, K., Wu, G., and Mohammed,
A.-A. (2020). A taxonomy and survey of power mod-
els and power modeling for cloud servers. ACM Com-
puting Surveys (CSUR), 53(5):1–41.
Martinez, B., Monton, M., Vilajosana, I., and Prades, J. D.
(2015). The power of models: Modeling power con-
sumption for iot devices. IEEE Sensors Journal,
15(10):5777–5789.
Merino, P., Mujica, G., Se
˜
nor, J., and Portilla, J. (2020). A
modular iot hardware platform for distributed and se-
cured extreme edge computing. Electronics, 9(3):538.
Moschitta, A. and Neri, I. (2014). Power consumption as-
sessment in wireless sensor networks. In ICT-Energy:
Concepts Towards Zero-Power Information and Com-
munication Technology. IntechOpen.
Rose, K., Eldridge, S., and Chapin, L. (2015). The internet
of things: An overview. The Internet Society (ISOC),
80(15):1–53.
Sawaguchi, S., Christmann, J.-F., and Lesecq, S. (2021).
Highly adaptive linear actor-critic for lightweight
Toward a More Realistic Energy Consumption Model for IoT Nodes in Extreme-Edge Computing Environments
79