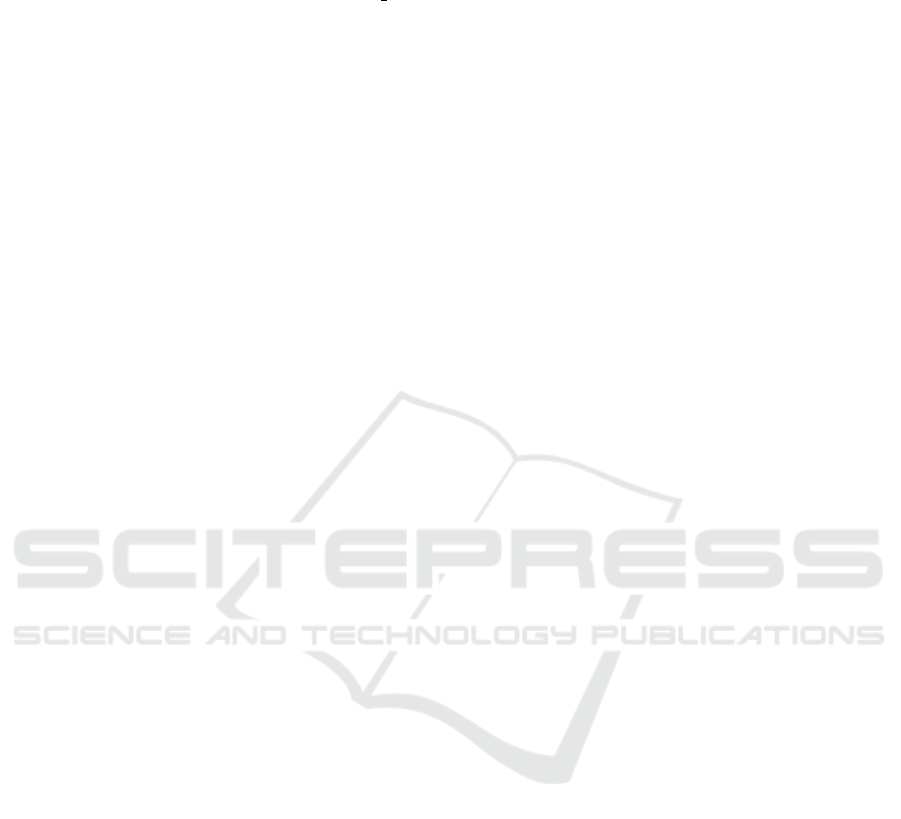
work was funded by the federal state of Baden-
W
¨
urttemberg as part of the Doctoral Certificate Pro-
gramme ”Wissensmedien” (grant number BW6 10).
REFERENCES
Badrinath, A., Wang, F., and Pardos, Z. A. (2021). pybkt:
An accessible library of bayesian knowledge tracing
models. In Hsiao, S. I., Sahebi, S. S., Bouchet, F., and
Vie, J., editors, Proceedings of the 14th International
Conference on Educational Data Mining, EDM 2021,
virtual, June 29 - July 2, 2021. International Educa-
tional Data Mining Society.
Bengio, Y., Courville, A., and Vincent, P. (2013a). Rep-
resentation learning: A review and new perspectives.
IEEE Transactions on Pattern Analysis and Machine
Intelligence, 35(8):1798–1828.
Bengio, Y., L
´
eonard, N., and Courville, A. C. (2013b).
Estimating or propagating gradients through stochas-
tic neurons for conditional computation. ArXiv,
abs/1308.3432.
Bulut, O., Shin, J., Yildirim-Erbasli, S. N., Gorgun, G., and
Pardos, Z. A. (2023). An introduction to bayesian
knowledge tracing with pybkt. Psych, 5(3):770–786.
Chen, J., Liu, Z., Huang, S., Liu, Q., and Luo, W. (2023).
Improving interpretability of deep sequential knowl-
edge tracing models with question-centric cognitive
representations. Proceedings of the AAAI Conference
on Artificial Intelligence, 37(12):14196–14204.
Choi, Y., Lee, Y., Shin, D., Cho, J., Park, S., Lee, S., Baek,
J., Bae, C., Kim, B., and Heo, J. (2020). Ednet: A
large-scale hierarchical dataset in education. In Inter-
national Conference on Artificial Intelligence in Edu-
cation, pages 69–73, Morocco. Springer.
Corbett, A. T. and Anderson, J. R. (1994). Knowledge trac-
ing: Modeling the acquisition of procedural knowl-
edge. User modeling and user-adapted interaction,
4:253–278.
Gervet, T., Koedinger, K., Schneider, J., Mitchell, T., et al.
(2020). When is deep learning the best approach to
knowledge tracing? Journal of Educational Data
Mining, 12(3):31–54.
Ghosh, A., Heffernan, N., and Lan, A. S. (2020). Context-
aware attentive knowledge tracing. In Proceedings
of the 26th ACM SIGKDD International Conference
on Knowledge Discovery & Data Mining, KDD ’20,
page 2330–2339, New York, NY, USA. Association
for Computing Machinery.
Khajah, M. M., Lindsey, R. V., and Mozer, M. C.
(2016). How deep is knowledge tracing? ArXiv,
abs/1604.02416.
Li, L. and Wang, Z. (2023). Calibrated q-matrix-
enhanced deep knowledge tracing with relational at-
tention mechanism. Applied Sciences, 13(4):2541.
Liu, Y., Yang, Y., Chen, X., Shen, J., Zhang, H., and
Yu, Y. (2020). Improving knowledge tracing via
pre-training question embeddings. arXiv preprint
arXiv:2012.05031.
Liu, Z., Liu, Q., Chen, J., Huang, S., Tang, J., and Luo,
W. (2022). pykt: A python library to benchmark
deep learning based knowledge tracing models. In
Advances in Neural Information Processing Systems,
volume 35, pages 18542–18555. Curran Associates,
Inc.
Nakagawa, H., Iwasawa, Y., and Matsuo, Y. (2018). End-
to-end deep knowledge tracing by learning binary
question-embedding. In 2018 IEEE International
Conference on Data Mining Workshops (ICDMW),
pages 334–342. IEEE.
Pel
´
anek, R. (2017). Bayesian knowledge tracing, logistic
models, and beyond: an overview of learner modeling
techniques. User Model. User Adapt. Interact., 27(3-
5):313–350.
Piech, C., Bassen, J., Huang, J., Ganguli, S., Sahami, M.,
Guibas, L. J., and Sohl-Dickstein, J. (2015). Deep
knowledge tracing. Advances in neural information
processing systems, 28.
Stamper, J., Niculescu-Mizil, A., Ritter, S., Gordon, G.,
and Koedinger, K. (2010). [data set name]. [chal-
lenge/development] data set from kdd cup 2010 edu-
cational data mining challenge. Retrieved from http://
pslcdatashop.web.cmu.edu/KDDCup/downloads.jsp.
Wang, W., Ma, H., Zhao, Y., and Li, Z. (2024). Pre-training
question embeddings for improving knowledge trac-
ing with self-supervised bi-graph co-contrastive learn-
ing. ACM Transactions on Knowledge Discovery from
Data, 18(4):1–20.
Wang, W., Ma, H., Zhao, Y., Li, Z., and He, X. (2021).
Relevance-aware q-matrix calibration for knowledge
tracing. In Artificial Neural Networks and Machine
Learning–ICANN 2021: 30th International Confer-
ence on Artificial Neural Networks, Bratislava, Slo-
vakia, September 14–17, 2021, Proceedings, Part III
30, pages 101–112. Springer.
Wang, W., Ma, H., Zhao, Y., Li, Z., and He, X. (2022).
Tracking knowledge proficiency of students with cal-
ibrated q-matrix. Expert Systems with Applications,
192:116454.
Yeung, C. (2019). Deep-irt: Make deep learning based
knowledge tracing explainable using item response
theory. In Proceedings of the 12th International
Conference on Educational Data Mining, Montr
´
eal,
Canada. International Educational Data Mining Soci-
ety (IEDMS).
Zhang, J., Shi, X., King, I., and Yeung, D. (2017). Dynamic
key-value memory networks for knowledge tracing.
In Proceedings of the 26th International Conference
on World Wide Web, pages 765–774, Perth, Australia.
ACM.
CSEDU 2025 - 17th International Conference on Computer Supported Education
84