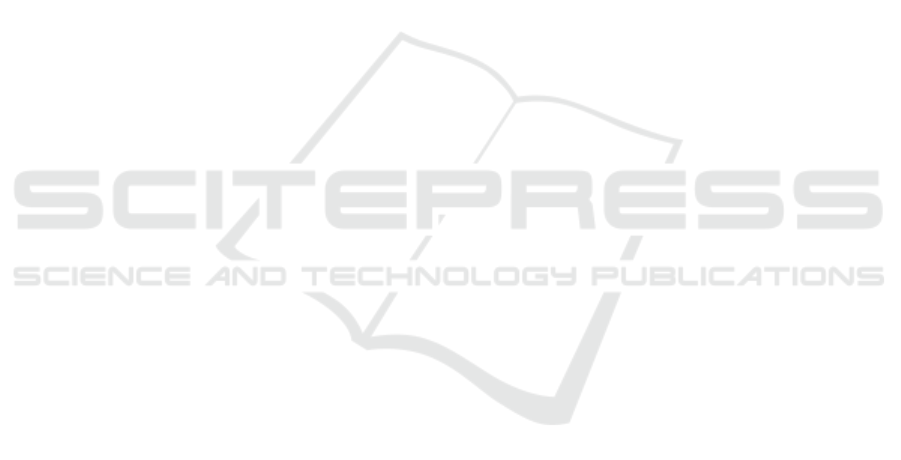
R
´
ıo, J. F., Wiebe, M., Peterson, P., G
´
erard-Marchant,
P., Sheppard, K., Reddy, T., Weckesser, W., Abbasi,
H., Gohlke, C., and Oliphant, T. E. (2020). Array pro-
gramming with numpy. Nat., 585:357–362.
Khajah, M. M., Lindsey, R. V., and Mozer, M. C.
(2016). How deep is knowledge tracing? ArXiv,
abs/1604.02416.
Kingma, D. and Ba, J. (2015). Adam: A method for
stochastic optimization. In 3rd International Confer-
ence on Learning Representations, San Diega, CA,
USA.
Lee, J. and Yeung, D.-Y. (2019). Knowledge query network
for knowledge tracing: How knowledge interacts with
skills. In Proceedings of the 9th International Con-
ference on Learning Analytics & Knowledge, LAK19,
page 491–500, New York, NY, USA. Association for
Computing Machinery.
Liu, Z., Liu, Q., Chen, J., Huang, S., Tang, J., and Luo,
W. (2022). pykt: A python library to benchmark
deep learning based knowledge tracing models. In
Advances in Neural Information Processing Systems,
volume 35, pages 18542–18555. Curran Associates,
Inc.
Mao, Y. (2018). Deep learning vs. bayesian knowledge trac-
ing: Student models for interventions. Journal of ed-
ucational data mining, 10(2).
Nagatani, K., Zhang, Q., Sato, M., Chen, Y., Chen, F.,
and Ohkuma, T. (2019). Augmenting knowledge trac-
ing by considering forgetting behavior. In Liu, L.,
White, R. W., Mantrach, A., Silvestri, F., McAuley,
J. J., Baeza-Yates, R., and Zia, L., editors, The World
Wide Web Conference, WWW 2019, San Francisco,
CA, USA, May 13-17, 2019, pages 3101–3107. ACM.
Oya, T. and Morishima, S. (2021). Lstm-sakt: Lstm-
encoded sakt-like transformer for knowledge tracing.
Piech, C., Bassen, J., Huang, J., Ganguli, S., Sahami, M.,
Guibas, L. J., and Sohl-Dickstein, J. (2015). Deep
knowledge tracing. Advances in neural information
processing systems, 28.
Rasch, G. (1993). Probabilistic models for some intelli-
gence and attainment tests. ERIC.
Settles, B., Brust, C., Gustafson, E., Hagiwara, M., and
Madnani, N. (2018). Second language acquisition
modeling. In Proceedings of the thirteenth workshop
on innovative use of NLP for building educational ap-
plications, pages 56–65, New Orleans, LA, USA. As-
sociation for Computational Linguistics.
Stamper, J., Niculescu-Mizil, A., Ritter, S., Gordon, G.,
and Koedinger, K. (2010). [data set name]. [chal-
lenge/development] data set from kdd cup 2010 edu-
cational data mining challenge. Retrieved from http://
pslcdatashop.web.cmu.edu/KDDCup/downloads.jsp.
Vaswani, A., Shazeer, N., Parmar, N., Uszkoreit, J., Jones,
L., Gomez, A. N., Kaiser, L. u., and Polosukhin, I.
(2017). Attention is all you need. In Advances in
Neural Information Processing Systems, volume 30.
Curran Associates, Inc.
Wu, L., Chen, X., Liu, F., Xie, J., Xia, C., Tan, Z., Tian, M.,
Li, J., Zhang, K., Lian, D., et al. (2025). Edustudio:
towards a unified library for student cognitive model-
ing. Frontiers of Computer Science, 19(8):198342.
Yeung, C. (2019). Deep-irt: Make deep learning based
knowledge tracing explainable using item response
theory. In Proceedings of the 12th International
Conference on Educational Data Mining, Montr
´
eal,
Canada. International Educational Data Mining Soci-
ety (IEDMS).
Yeung, C. and Yeung, D. (2018). Addressing two problems
in deep knowledge tracing via prediction-consistent
regularization. In Proceedings of the Fifth Annual
ACM Conference on Learning at Scale, pages 5:1–
5:10, London, UK. ACM.
Zhang, J., Shi, X., King, I., and Yeung, D. (2017). Dynamic
key-value memory networks for knowledge tracing.
In Proceedings of the 26th International Conference
on World Wide Web, pages 765–774, Perth, Australia.
ACM.
Addressing Label Leakage in Knowledge Tracing Models
95