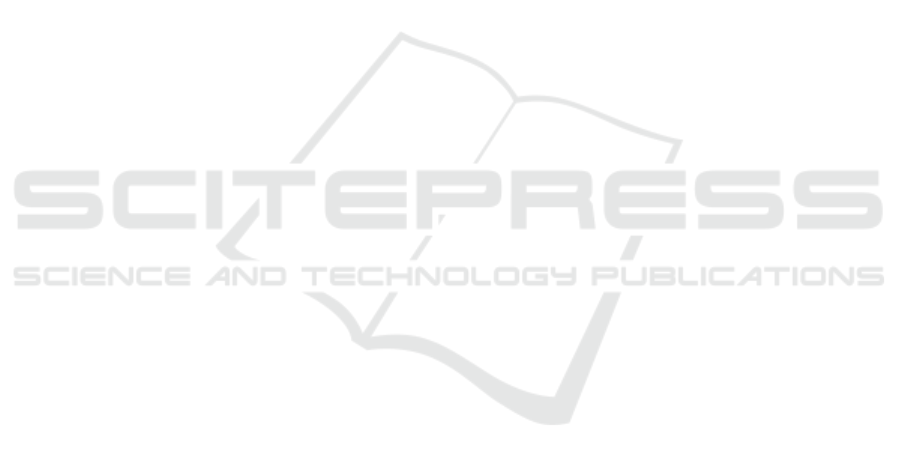
[”a”, ”b”, ”c”]), the references can be resolved by ex-
ploring the component graph, treated as a prefix tree.
The resolution process could start from the locality
node of the reference (for example the function in
which it is contained) and walk up the prefix tree until
the current prefix identifier of the reference (the first
identifier of the chain) is found as a child node of the
current scope node. Then, a downward search can be
carried out until all the identifiers of the reference are
exhausted (each time a prefix identifier matches, it is
removed from the array).
REFERENCES
Avgeriou, P., Kruchten, P., Ozkaya, I., and Seaman, C. B.
(2016). Managing technical debt in software engi-
neering (dagstuhl seminar 16162). Dagstuhl Reports,
6(4):110–138.
Avgeriou, P., Taibi, D., Ampatzoglou, A., Arcelli Fontana,
F., Besker, T., Chatzigeorgiou, A., Lenarduzzi, V.,
Martini, A., Moschou, A., Pigazzini, I., Saarimaki,
N., Sas, D. D., De Toledo, S. S., and Tsintzira, A. A.
(2021). An Overview and Comparison of Technical
Debt Measurement Tools. IEEE Software, 38(3).
Azadi, U., Fontana, F. A., and Taibi, D. (2019). Archi-
tectural smells detected by tools: a catalogue pro-
posal. In Proc, of the II Int. Conf, on Techni-
cal Debt, TechDebt@ICSE 2019, Montreal, Canada,
2019, pages 88–97. IEEE / ACM.
Brandes, U., Eiglsperger, M., Lerner, J., and Pich, C.
(2013). Graph markup language (graphml). In Tamas-
sia, R., editor, Handbook on Graph Drawing and Vi-
sualization, pages 517–541. Chapman and Hall/CRC.
Collard, M. L., Decker, M. J., and Maletic, J. I. (2013). sr-
cml: An infrastructure for the exploration, analysis,
and manipulation of source code: A tool demonstra-
tion. In 2013 IEEE International conference on soft-
ware maintenance, pages 516–519. IEEE.
Creager, D. A. and van Antwerpen, H. (2023). Stack graphs:
Name resolution at scale. In L
¨
ammel, R., Mosses,
P. D., and Steimann, F., editors, Eelco Visser Com-
memorative Symposium, EVCS 2023, April 5, 2023,
Delft, The Netherlands, OASIcs. Schloss Dagstuhl -
Leibniz-Zentrum f
¨
ur Informatik.
DeRemer, F. (1976). Lexical analysis. In Bauer, F. L.
and Eickel, J., editors, Compiler Construction, An Ad-
vanced Course, 2nd ed, volume 21 of Lecture Notes in
Computer Science, pages 109–120. Springer.
Ducasse, S., Anquetil, N., Bhatti, M. U., Cavalcante Hora,
A., Laval, J., and Girba, T. (2011). MSE and FAMIX
3.0: an Interexchange Format and Source Code Model
Family. Research report.
Fowler, M. and Beck, K. (2002). Refactoring: Improving
the Design of Existing Code. Addison-Wesley.
Grech, N., Georgiou, K., Pallister, J., Kerrison, S., Morse,
J., and Eder, K. (2015). Static analysis of energy con-
sumption for llvm ir programs. SCOPES ’15, page
12–21, NY, USA. ACM.
Latif, A., Azam, F., Anwar, M. W., and Zafar, A. (2023).
Comparison of leading language parsers – antlr,
javacc, sablecc, tree-sitter, yacc, bison. In 2023 13th
International Conference on Software Technology and
Engineering (ICSTE), pages 7–13.
Marin, V. J. and Rivero, C. R. (2018). Towards a frame-
work for generating program dependence graphs from
source code. In Proceedings of the 4th ACM SIG-
SOFT International Workshop on Software Analytics,
SWAN 2018, page 30–36, New York, NY, USA. As-
sociation for Computing Machinery.
Pruijt, L., K
¨
oppe, C., van der Werf, J. M. E. M., and
Brinkkemper, S. (2017). The accuracy of dependency
analysis in static architecture compliance checking.
Softw. Pract. Exp., 47(2):273–309.
Roveda, R., Fontana, F. A., Pigazzini, I., and Zanoni, M.
(2018). Towards an architectural debt index. In Pro-
ceedings - 44th Euromicro Conference on Software
Engineering and Advanced Applications, SEAA 2018,
pages 408–416. IEEE.
Sas, D. and Avgeriou, P. (2023). An architectural techni-
cal debt index based on machine learning and archi-
tectural smells. IEEE Transactions on Software Engi-
neering, pages 1–27.
Sas, D., Avgeriou, P., and Fontana, F. A. (2019). Investigat-
ing instability architectural smells evolution: An ex-
ploratory case study. In 2019 IEEE Int.Conf. on Soft-
ware Maintenance and Evolution, ICSME, OH, USA,
2019, pages 557–567. IEEE.
van Antwerpen, H., Bach Poulsen, C., Rouvoet, A., and
Visser, E. (2018). Scopes as types. Proc. ACM Pro-
gram. Lang., 2(OOPSLA).
Weiss, K. and Banse, C. (2022). A language-independent
analysis platform for source code. CoRR.
Zwaan, A. and van Antwerpen, H. (2023). Scope graphs:
The story so far. In Eelco Visser Commemorative Sym-
posium, 2023, Delft, The Netherlands, volume 109 of
OASIcs. Schloss Dagstuhl.
Lessons Learned from Implementing a Language-Agnostic Dependency Graph Parser
491