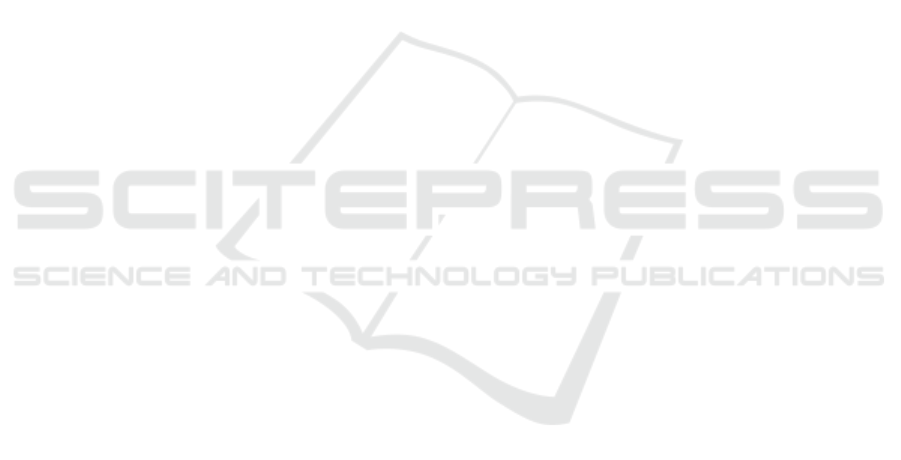
based fault localization. J. Softw. Evol. Process.,
36(6).
Dutta, A. (2022). Poster: Ebfl-an ensemble classifier
based fault localization. In 2022 IEEE Conference on
Software Testing, Verification and Validation (ICST),
pages 473–476.
Ghosh, D., Singh, J. P., and Singh, J. (2022). An adaptive
approach for fault localization using r-cnn. In 2022
International Conference on Advancements in Smart,
Secure and Intelligent Computing (ASSIC), pages 1–6.
Jo, J.-H., Lee, J., Jaffari, A., and Kim, E. (2021). Fault lo-
calization with data flow information and an artificial
neural network. International Journal of Software In-
novation (IJSI), 9(3):66–78.
Jones, J. A., Harrold, M. J., and Stasko, J. (2002). Visual-
ization of test information to assist fault localization.
page 467–477, New York, NY, USA. Association for
Computing Machinery.
Just, R., Jalali, D., and Ernst, M. D. (2014). Defects4j: A
database of existing faults to enable controlled test-
ing studies for java programs. In Proceedings of the
2014 International Symposium on Software Testing
and Analysis, ISSTA 2014, page 437–440, New York,
NY, USA. Association for Computing Machinery.
Li, Y., Wang, S., and Nguyen, T. (2021). Fault localiza-
tion with code coverage representation learning. In
2021 IEEE/ACM 43rd International Conference on
Software Engineering (ICSE), pages 661–673.
Lou, Y., Zhu, Q., Dong, J., Li, X., Sun, Z., Hao, D.,
Zhang, L., and Zhang, L. (2021). Boosting coverage-
based fault localization via graph-based representa-
tion learning. In Proceedings of the 29th ACM Joint
Meeting on European Software Engineering Confer-
ence and Symposium on the Foundations of Software
Engineering, ESEC/FSE 2021, page 664–676, New
York, NY, USA. ACM.
Masri, W. (2010). Fault localization based on informa-
tion flow coverage. Softw. Test. Verification Reliab.,
20(2):121–147.
Parnin, C. and Orso, A. (2011). Are automated debugging
techniques actually helping programmers? In Pro-
ceedings of the 20th International Symposium on Soft-
ware Testing and Analysis, ISSTA 2011, Toronto, ON,
Canada, July 17-21, 2011, pages 199–209. ACM.
Pearson, S., Campos, J., Just, R., Fraser, G., Abreu, R.,
Ernst, M. D., Pang, D., and Keller, B. (2017). Evalu-
ating and improving fault localization. In Proceedings
of the 39th International Conference on Software En-
gineering, ICSE ’17, page 609–620. IEEE Press.
Qian, J., Ju, X., and Chen, X. (2023). Gnet4fl: effective
fault localization via graph convolutional neural net-
work. Automated Software Engineering, 30(2):16.
Qian, J., Ju, X., Chen, X., Shen, H., and Shen, Y. (2021).
Agfl: A graph convolutional neural network-based
method for fault localization. In 2021 IEEE 21st Inter-
national Conference on Software Quality, Reliability
and Security (QRS), pages 672–680.
Rapps, S. and Weyuker, E. (1985). Selecting software test
data using data flow information. IEEE Transactions
on Software Engineering, SE-11(4):367–375.
Ren, S., Zuo, X., Chen, J., and Tan, W. (2022). Statement
spectrum with two dimensional eigenvalues for intelli-
gent software fault localization. Journal of Intelligent
& Fuzzy Systems, 42:2899–2914. 4.
Ribeiro, H. L., de Araujo, R. P. A., Chaim, M. L., de Souza,
H. A., and Kon, F. (2019). Evaluating data-flow cov-
erage in spectrum-based fault localization. In 2019
ACM/IEEE International Symposium on Empirical
Software Engineering and Measurement, ESEM 2019,
Porto de Galinhas, Recife, Brazil, September 19-20,
2019, pages 1–11. IEEE.
Santelices, R., Jones, J. A., Yanbing Yu, and Harrold, M. J.
(2009). Lightweight fault-localization using multiple
coverage types. In 2009 IEEE 31st International Con-
ference on Software Engineering, pages 56–66.
Silva, D. L. d. and Chaim, M. L. (2021). Impacto da relac¸
˜
ao
de subsunc¸
˜
ao na localizac¸
˜
ao de defeitos baseados em
espectros de fluxo de dados. Master’s thesis, Escola
de Artes, Ci
ˆ
encias e Humanidades, Universidade de
S
˜
ao Paulo, S
˜
ao Paulo.
Wong, E. and Qi, Y. (2011). Bp neural network-based effec-
tive fault localization. International Journal of Soft-
ware Engineering and Knowledge Engineering, 19.
Wong, W. E. (2023). Machine Learning-Based Techniques
for Software Fault Localization, pages 297–319.
Yan, Y., Jiang, S., Wang, R., Zhang, C., Wang, C., Zhang,
S., and Wen, M. (2022). A fault localization approach
based on birnn and multi-dimensional features. Inter-
national Journal of Software Engineering and Knowl-
edge Engineering, 32(08):1179–1201.
Zakari, A., Lee, S., Alam, K., and Ahmad, R. (2018). Soft-
ware fault localisation: A systematic mapping study.
IET Software, 13.
Zhang, Z., Lei, Y., Mao, X., Yan, M., Xu, L., and Zhang, X.
(2021). A study of effectiveness of deep learning in
locating real faults. Information and Software Tech-
nology, 131:106486.
Zheng, W., Hu, D., and Wang, J. (2016). Fault localization
analysis based on deep neural network. Mathematical
Problems in Engineering, 2016:1–11.
Zhuo, Z., Lei, Y., Tan, Q., Mao, X., Zeng, P., and Chang,
x. (2017). Deep learning-based fault localization with
contextual information. IEICE Transactions on Infor-
mation and Systems, E100.D:3027–3031.
A Study on Different Spectra in Fault Localization
499