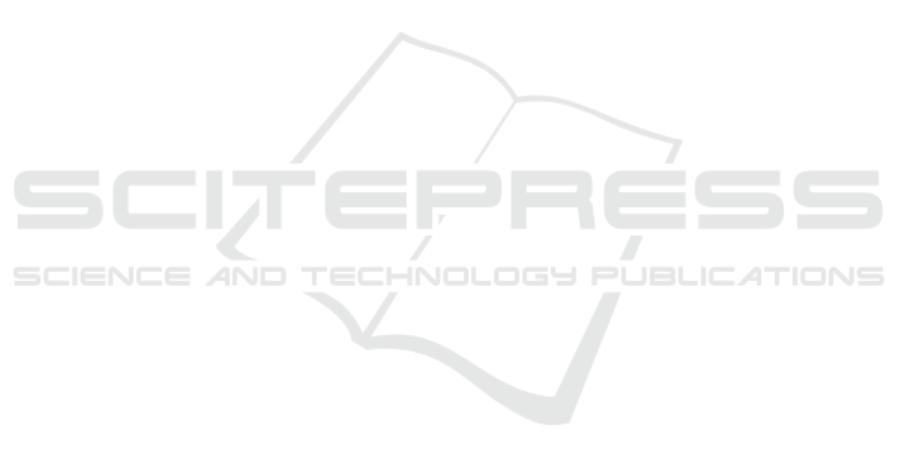
training.
ACKNOWLEDGMENTS
This result has been partially supported by
grant PID2021-127275OB-I00 funded by MICI-
U/AEI/10.13039/501100011033 and by “ERDF A
way of making Europe”, grant PDC2023-145863-I00
funded by MICIU/AEI/10.13039/501100011033 and
by “European Union NextGenerationEU/PRTR”,
and grant M.2 PDC_000756 funded by Consejería
de Universidad, Investigación e Innovación and by
ERDF Andalusia Program 2021-2027.
REFERENCES
Alowais, S. A., Alghamdi, S. S., Alsuhebany, N., Alqahtani,
T., Alshaya, A. I., Almohareb, S. N., Aldairem, A.,
Alrashed, M., Bin Saleh, K., Badreldin, H. A., et al.
(2023). Revolutionizing healthcare: the role of arti-
ficial intelligence in clinical practice. BMC medical
education, 23(1):689.
Bhuiyan, M. N., Rahman, M. M., Billah, M. M., and Saha,
D. (2021). Internet of things (iot): A review of its en-
abling technologies in healthcare applications, stan-
dards protocols, security, and market opportunities.
IEEE Internet of Things Journal, 8(13):10474–10498.
Chang, J. C., Amershi, S., and Kamar, E. (2017). Re-
volt: Collaborative crowdsourcing for labeling ma-
chine learning datasets. In Proceedings of the 2017
CHI Conference on Human Factors in Computing Sys-
tems, CHI ’17, page 2334–2346, New York, NY, USA.
Association for Computing Machinery.
Dang, L. M., Min, K., Wang, H., Piran, M. J., Lee, C. H.,
and Moon, H. (2020). Sensor-based and vision-based
human activity recognition: A comprehensive survey.
Pattern Recognition, 108:107561.
Davenport, T. and Kalakota, R. (2019). The potential for
artificial intelligence in healthcare. Future healthcare
journal, 6(2):94–98.
Dong, X., Gabrilovich, E., Heitz, G., Horn, W., Lao, N.,
Murphy, K., Strohmann, T., Sun, S., and Zhang, W.
(2014). Knowledge vault: a web-scale approach to
probabilistic knowledge fusion. In Proceedings of
the 20th ACM SIGKDD International Conference on
Knowledge Discovery and Data Mining, KDD ’14,
page 601–610, New York, NY, USA. Association for
Computing Machinery.
Duan, Y., Edwards, J., and Dwivedi, Y. K. (2019). Artificial
intelligence for decision making in the era of big data
- evolution, challenges and research agenda. Int. J. Inf.
Manag., 48:63–71.
Haug, C. J. and Drazen, J. M. (2023). Artificial intelligence
and machine learning in clinical medicine, 2023. New
England Journal of Medicine, 388(13):1201–1208.
Huang, Q. and Zhao, T. (2024). Data collection and label-
ing techniques for machine learning. arXiv preprint
arXiv:2407.12793.
Jobanputra, C., Bavishi, J., and Doshi, N. (2019). Human
activity recognition: A survey. Procedia Computer
Science, 155:698–703.
Johnson, K. B., Wei, W.-Q., Weeraratne, D., Frisse, M. E.,
Misulis, K., Rhee, K., Zhao, J., and Snowdon, J. L.
(2021). Precision medicine, ai, and the future of per-
sonalized health care. Clinical and translational sci-
ence, 14(1):86–93.
Ke, S.-R., Thuc, H. L. U., Lee, Y.-J., Hwang, J.-N., Yoo, J.-
H., and Choi, K.-H. (2013). A review on video-based
human activity recognition. Computers, 2(2):88–131.
Kumar, Y., Koul, A., Singla, R., and Ijaz, M. F. (2023). Ar-
tificial intelligence in disease diagnosis: a systematic
literature review, synthesizing framework and future
research agenda. Journal of ambient intelligence and
humanized computing, 14(7):8459–8486.
Laghari, A. A., Wu, K., Laghari, R. A., Ali, M., and Khan,
A. A. (2021). A review and state of art of internet of
things (iot). Archives of Computational Methods in
Engineering, pages 1–19.
Miller, D. and Brown, E. (2017). Artificial intelligence in
medical practice: The question to the answer? The
American journal of medicine, 131 2:129–133.
Mouha, R. A. R. A. et al. (2021). Internet of things (iot).
Journal of Data Analysis and Information Processing,
9(02):77.
Ramanujam, E., Perumal, T., and Padmavathi, S. (2021).
Human activity recognition with smartphone and
wearable sensors using deep learning techniques: A
review. IEEE Sensors Journal, 21(12):13029–13040.
Ratner, A. J., Bach, S. H., Ehrenberg, H. R., and Ré, C.
(2017). Snorkel: Fast training set generation for infor-
mation extraction. In Proceedings of the 2017 ACM
International Conference on Management of Data,
SIGMOD ’17, page 1683–1686, New York, NY, USA.
Association for Computing Machinery.
Shaikh, M. B. and Chai, D. (2021). Rgb-d data-based action
recognition: a review. Sensors, 21(12):4246.
Wang, J., Kraska, T., Franklin, M. J., and Feng, J. (2012).
Crowder: crowdsourcing entity resolution. Proc.
VLDB Endow., 5(11):1483–1494.
Wu, R., Sakala, P., Li, P., Chu, X., and He, Y.
(2021). Demonstration of panda: a weakly super-
vised entity matching system. Proc. VLDB Endow.,
14(12):2735–2738.
Yu, K.-H., Beam, A. L., and Kohane, I. S. (2018). Artifi-
cial intelligence in healthcare. Nature biomedical en-
gineering, 2(10):719–731.
Zhang, C. and Lu, Y. (2021). Study on artificial intelligence:
The state of the art and future prospects. Journal of
Industrial Information Integration, 23:100224.
StreamTag: A Platform for Flexible Tagged Data Management
239