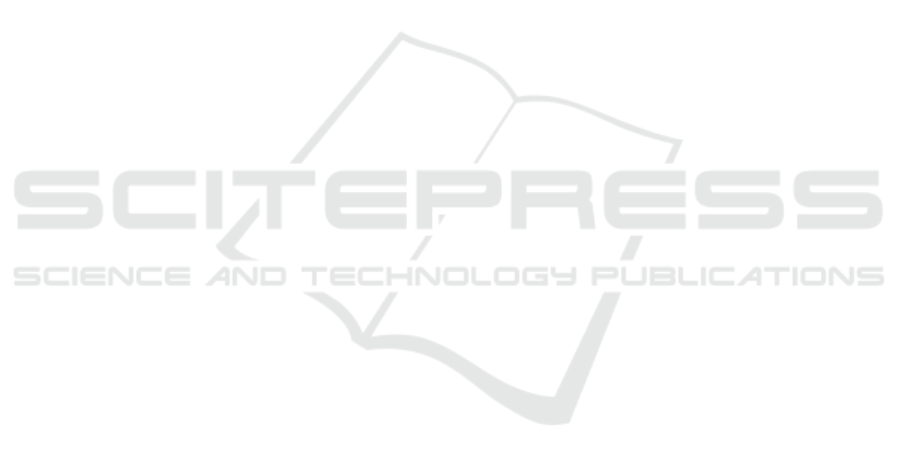
Bian, S., Liu, M., Zhou, B., and Lukowicz, P. (2022). The
state-of-the-art sensing techniques in human activity
recognition: A survey. Sensors, 22(12):4596.
Camp, N., Johnston, J., Lewis, M. G., Zecca, M., Di Nuovo,
A., Hunter, K., and Magistro, D. (2022). Percep-
tions of in-home monitoring technology for activi-
ties of daily living: Semistructured interview study
with community-dwelling older adults. JMIR aging,
5(2):e33714.
Claes, V., Devriendt, E., Tournoy, J., and Milisen, K.
(2015). Attitudes and perceptions of adults of 60 years
and older towards in-home monitoring of the activi-
ties of daily living with contactless sensors: an explo-
rative study. International journal of nursing studies,
52(1):134–148.
Codina, T. (2022). Konekta2, sensorizaci
´
on inteligente
de viviendas de personas mayores - fundaci
´
o
isocial. innovaci
´
o en l’acci
´
o social. Available
online: https://isocial.cat/es/konekta2-sensoritzacio-
intel-ligent-dhabitatges-de-persones-grans/ (accessed
on 14 May 2024).
Dang, L. M., Min, K., Wang, H., Piran, M. J., Lee, C. H.,
and Moon, H. (2020). Sensor-based and vision-based
human activity recognition: A comprehensive survey.
Pattern Recognition, 108:107561.
Del-Valle-Soto, C., Nolazco-Flores, J. A., Del Puerto-
Flores, J. A., Vel
´
azquez, R., Valdivia, L. J., Rosas-
Caro, J., and Visconti, P. (2022). Statistical study
of user perception of smart homes during vital signal
monitoring with an energy-saving algorithm. Interna-
tional Journal of Environmental Research and Public
Health, 19(16):9966.
Espinilla, M., Mart
´
ınez, L., Medina, J., and Nugent, C.
(2018a). The experience of developing the ujami
smart lab. Ieee Access, 6:34631–34642.
Espinilla, M., Medina, J., Hallberg, J., and Nugent, C.
(2018b). A new approach based on temporal sub-
windows for online sensor-based activity recognition.
Journal of Ambient Intelligence and Humanized Com-
puting, pages 1–13.
Fan, C. and Gao, F. (2021). Enhanced human activity recog-
nition using wearable sensors via a hybrid feature se-
lection method. Sensors (Basel, Switzerland), 21.
Franco, A., Magnani, A., and Maio, D. (2020). A multi-
modal approach for human activity recognition based
on skeleton and rgb data. Pattern Recognit. Lett.,
131:293–299.
Fu, B., Damer, N., Kirchbuchner, F., and Kuijper, A. (2020).
Sensing technology for human activity recognition: A
comprehensive survey. Ieee Access, 8:83791–83820.
Huang, W., Zhang, L., Gao, W., Min, F., and He, J.
(2021). Shallow convolutional neural networks for
human activity recognition using wearable sensors.
IEEE Transactions on Instrumentation and Measure-
ment, 70:1–11.
Hussain, A., Hussain, T., Ullah, W., and Baik, S. (2022).
Vision transformer and deep sequence learning for hu-
man activity recognition in surveillance videos. Com-
putational Intelligence and Neuroscience, 2022.
K
¨
onig, A., Junior, C. C., Derreumaux, A., Bensadoun, G.,
Petit, P.-D., Br
´
emond, F., David, R., Verhey, F., Aal-
ten, P., and Robert, P. (2015). Validation of an au-
tomatic video monitoring system for the detection of
instrumental activities of daily living in dementia pa-
tients. Journal of Alzheimer’s disease : JAD, 44
2:675–85.
Langheinrich, M. (2002). A privacy awareness system
for ubiquitous computing environments. In UbiComp
2002: Ubiquitous Computing: 4th International Con-
ference G
¨
oteborg, Sweden, September 29–October 1,
2002 Proceedings 4, pages 237–245. Springer.
Lee, J.-S., Su, Y.-W., and Shen, C.-C. (2007). A compara-
tive study of wireless protocols: Bluetooth, uwb, zig-
bee, and wi-fi. In IECON 2007 - 33rd Annual Confer-
ence of the IEEE Industrial Electronics Society, pages
46–51.
Liu, J., Liu, H., Chen, Y., Wang, Y., and Wang, C. (2020).
Wireless sensing for human activity: A survey. IEEE
Communications Surveys & Tutorials, 22:1629–1645.
Montagut-Mart
´
ınez, P., Garc
´
ıa-Arenas, J. J., Romero-
L
´
opez, M., Rodr
´
ıguez-Rodr
´
ıguez, N., P
´
erez-Cruzado,
D., and Gonz
´
alez-Lama, J. (2022). Feasibility of an
activity control system in patients with diabetes: A
study protocol of a randomised controlled trial. Dia-
betes, Metabolic Syndrome and Obesity: Targets and
Therapy, pages 2683–2691.
Montoro Lend
´
ınez, A., L
´
opez Ruiz, J. L., Nugent, C., and
Espinilla Est
´
evez, M. (2023). Activa: Innovation in
quality of care for nursing homes through activity
recognition. IEEE Access, 11:123335–123349.
Mottram, S., Peat, G., Thomas, E., Wilkie, R., and Croft,
P. (2008). Patterns of pain and mobility limitation in
older people: cross-sectional findings from a popula-
tion survey of 18,497 adults aged 50 years and over.
Quality of Life Research, 17:529–539.
Niccoli, T. and Partridge, L. (2012). Ageing as a risk factor
for disease. Current Biology, 22:R741–R752.
Ramirez, H., Velast
´
ın, S., Meza, I., Fabregas, E., Makris,
D., and Farias, G. (2021). Fall detection and activ-
ity recognition using human skeleton features. IEEE
Access, 9:33532–33542.
Steele, R., Lo, A., Secombe, C., and Wong, Y. K. (2009).
Elderly persons’ perception and acceptance of using
wireless sensor networks to assist healthcare. Inter-
national journal of medical informatics, 78(12):788–
801.
Su, J., Liao, Z., Sheng, Z., Liu, A. X., Singh, D., and
Lee, H.-N. (2023). Human activity recognition us-
ing self-powered sensors based on multilayer bidirec-
tional long short-term memory networks. IEEE Sen-
sors Journal, 23(18):20633–20641.
Umbricht, D., Cheng, W.-Y., Lipsmeier, F., Bamdadian,
A., and Lindemann, M. (2020). Deep learning-
based human activity recognition for continuous ac-
tivity and gesture monitoring for schizophrenia pa-
tients with negative symptoms. Frontiers in Psychi-
atry, 11:574375.
United Nations (2022). World Population Prospects. Avail-
able online: https://population.un.org/wpp/ (accessed
on 14 May 2024).
Yuan, L., Andrews, J., Mu, H., Vakil, A., Ewing, R., Blasch,
E., and Li, J. (2022). Interpretable passive multi-
modal sensor fusion for human identification and ac-
tivity recognition. Sensors (Basel, Switzerland), 22.
Perceptions and Acceptability of Sensor-Based Activity Recognition Systems Among Older Adults and Their Families
247