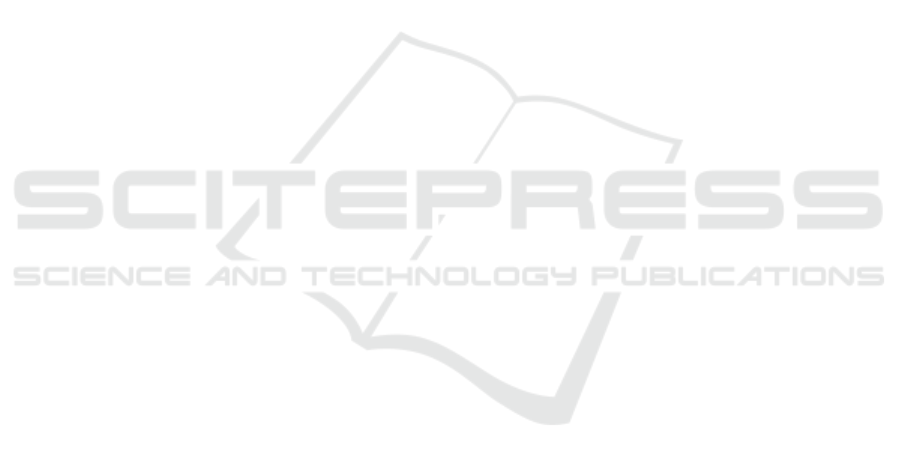
naive algorithm using appropriate libraries such as
OpenMP and SYCL. These approaches significantly
reduce computation time compared to sequential pro-
grams (Ogunyiola et al., 2024). Matrix multiplica-
tion is a subject well known to every computer sci-
ence student. It is a straightforward operation, with a
basic algorithm that is simple to understand and im-
plement. The operation can be parallelized in many
ways. It is therefore a good example for introducing
students to more advanced topics like parallel pro-
gramming. When teaching parallel programming, it
is crucial to emphasize key aspects such as program
execution time and computational accuracy. This arti-
cle contains example implementations of parallel ma-
trix multiplication and the results of measuring the
time and accuracy of calculations. Students in paral-
lel programming courses can make such implemen-
tations on their own to then test their performance
and accuracy of computation. The aim of this pa-
per is to present methods for teaching parallel pro-
gramming on CPU, due to its widespread availability.
The paper discusses the library optimized for Intel
processors, such as MKL, and the popular OpenMP
standard, as well as the modern framework SYCL.
This analysis allows students to understand the im-
pact of hardware architecture and code optimization
on performance. In this paper Intel processor was
chosen to run the tests, but it is not the only option.
It is possible to use run such tests on another archi-
tecture. Other programming languages can also be
used to work with OpenMP and SYCL. In this article,
C++ was chosen because it is well known to students
and widely used. However, the paper omits GPUs
to avoid complications related to heterogeneous com-
puting systems. This article presents timing results
for a naive sequential algorithm without using any li-
braries for parallel computing, as well as results using
OpenMP and SYCL. Additionally, the performance
of matrix multiplication using the MKL library is ana-
lyzed. The tests assess the accuracy of each approach
by comparing the results to the sequential naive al-
gorithm, with the mean square error (MSE) serving
as the accuracy metric. The naive sequential algo-
rithm is used as the benchmark. The results obtained
in this paper show that SYCL performs the compu-
tation faster than OpenMP, while maintaining similar
accuracy.However, both libraries lag significantly be-
hind MKL in terms of execution speed for this im-
plementation. Therefore, it is worth presenting this
solution to students to encourage them to extend their
knowledge in this area and further optimise their code
and learn about algorithms dedicated to distributed
matrix multiplication. Chapter 2 describes libraries
chosen to present in this paper. Chapter 3 provides
a description of an example exercise that can be car-
ried out during classes with students. Chapter 4 con-
tains the implementation of the algorithms using the
libraries discussed. These include code fragments of
the programmes, together with a description of how
they were compiled so that they can be reproduced.
Chapter 5 describes the testing environment, includ-
ing relevant environment variables and a description
of the numerical experiment itself. Chapter 6 presents
the experimental results: execution times, computa-
tional accuracy, and speed-ups relative to the baseline
algorithm. Chapter 7 provides a summary and out-
lines potential directions for future research.
2 LIBRARIES
In this paper OpenMP and SYCL libraries were cho-
sen for the study because they allow software de-
velopment for heterogeneous systems. This implies
code portability between CPU and GPU, which is a
basis for further research. However, this article fo-
cuses exclusively on a single hardware configuration:
a multi-core CPU with shared memory. The portabil-
ity of solutions between CPUs and GPUs is beyond
the scope of this work. Intel MKL library is also
included in the comparison. Intel Math Kernel Li-
brary (MKL) is an advanced mathematical library op-
timised for performance on Intel processors that sup-
ports a wide range of computational operations such
as linear algebra (including double-precision matrix
multiplication), Fourier transforms, statistics or vec-
tor functions. With optimised algorithms and the abil-
ity to automatically adapt to the hardware architec-
ture, MKL allows computational applications in areas
such as machine learning, scientific simulation or en-
gineering modelling to be significantly accelerated. It
supports a variety of programming languages, includ-
ing C, C++ and Fortran, and integrates with popular
frameworks, making it a versatile tool for developers.
Using MKL can reduce computation time, enabling
faster results and more efficient use of available hard-
ware resources (Intel Corporation, ). OpenMP (Open
Multi-Processing) is a widely-used parallel program-
ming tool that enables the effective use of multi-
threading in applications running on today’s multi-
core processors. It is an open standard that integrates
with popular programming languages such as C, C++
and Fortran, allowing code to be easily extended with
parallel functionality using special directives, library
functions and environment variables. Thanks to its
flexibility, OpenMP allows programmers to control
the division of tasks between threads, synchronisa-
tion, as well as resource management, making it sig-
CSEDU 2025 - 17th International Conference on Computer Supported Education
714