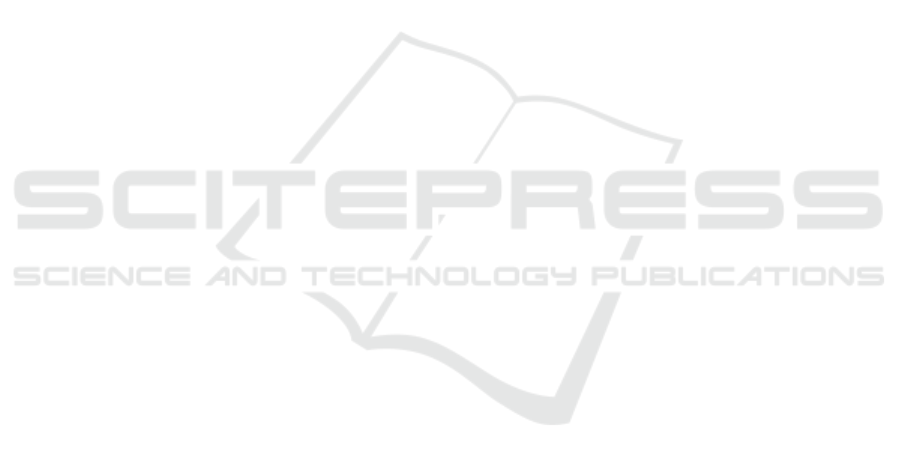
Publications, Lda.
Boenig-Liptsin, M., Tanweer, A., and Edmundson, A.
(2022). Data science ethos lifecycle: Interplay of
ethical thinking and data science practice. Journal
of Statistics and Data Science Education, 30(3):228–
240.
Borgman, C. L. (2019). The lives and afterlives of data.
Harvard Data Science Review, 1(1).
Borowicc, S. and Alves-Souza, S. (2024). Heterogeneous
Data Integration: A Literature Scope Review:. In Pro-
ceedings of the 26th International Conference on En-
terprise Information Systems, pages 189–200, Angers,
France. SCITEPRESS - Science and Technology Pub-
lications.
Brasil, M. d. S. d. B. (2024a). Painel de monitoramento das
arboviroses. Acesso em: 8 nov. 2024.
Brasil, M. d. S. M. (2009). Diretrizes nacionais para a
prevenc¸
˜
ao e controle de epidemias de dengue. S
´
erie
A. Normas e Manuais T
´
ecnicos. Minist
´
erio da Sa
´
ude,
Bras
´
ılia.
Brasil, M. d. S. M. (2024b). Comiss
˜
ao intergestores tripar-
tite. Acesso em: 8 nov. 2024.
Brasil, M. d. S. M. (2024c). Doenc¸as e
agravos de notificac¸
˜
ao de 2007 em diante
(sinan). https://datasus.saude.gov.br/acesso-a-
informacao/doencas-e-agravos-de-notificacao-de-
2007-em-diante-sinan/. Accessed: 2024-11-17.
Carvalho, C. N., Dourado, I., and Bierrenbach, A. L. (2011).
Subnotificac¸
˜
ao da comorbidade tuberculose e aids:
uma aplicac¸
˜
ao do m
´
etodo de linkage. Revista de
Sa
´
ude P
´
ublica, 45:548–555. Publisher: Faculdade de
Sa
´
ude P
´
ublica da Universidade de S
˜
ao Paulo.
Codeco, C., Coelho, F., Cruz, O., Oliveira, S., Castro, T.,
and Bastos, L. (2018). Infodengue: A nowcasting sys-
tem for the surveillance of arboviruses in brazil. Revue
d’
´
Epid
´
emiologie et de Sant
´
e Publique, 66:S386. Eu-
ropean Congress of Epidemiology “Crises, epidemio-
logical transitions and the role of epidemiologists”.
Coeli, C. M. (2006). Relacionamento de Bases de Dados
em Sa
´
ude. CADERNOS SA
´
UDE COLETIVA, 14(2).
Cunningham, W. and Leuf, B. (2002). The Wiki Way: Quick
Collaboration on the Web. Addison-Wesley, Boston,
MA.
Dekhtyar, A. (2023). DATA 301: Introduction to Data Sci-
ence. Lecture notes.
Deshpande, P., Rasin, A., Tchoua, R., Furst, J., Raicu,
D., Schinkel, M., Trivedi, H., and Antani, S. (2023).
Biomedical heterogeneous data categorization and
schema mapping toward data integration. Frontiers
in Big Data, 6. Publisher: Frontiers.
Gasc
´
o-Hern
´
andez, M., Martin, E. G., Reggi, L., Pyo, S., and
Luna-Reyes, L. F. (2018). Promoting the use of open
government data: Cases of training and engagement.
Government Information Quarterly, 35:233–242.
Keller, S. A., Shipp, S. S., Schroeder, A. D., and Korkmaz,
G. (2020). Doing data science: A framework and case
study. Harvard Data Science Review.
Kimball, R. and Caserta, J. (2004). The Data Warehouse
ETL Toolkit: Practical Techniques for Extracting,
Cleaning, Conforming, and Delivering Data. Wiley,
Indianapolis, IN.
Lazarre, W., Guidedi, K., Amaria, S., and Kolyang (2022).
Modular Ontology Design: A State-of-Art of Dis-
eases Ontology Modeling and Possible Issue. Revue
d’Intelligence Artificielle, 36(3):497–501.
Mahmoud, A., Shams, M. Y., Elzeki, O. M., and Awad,
N. A. (2021). Using semantic web technologies to
improve the extract transform load model. Comput-
ers, Materials & Continua, 68(2):2711–2726.
Pinto, L. F., Carvalho, A. A. d., and Pisco, L. A. C. (2024).
Inovac¸
˜
oes na gest
˜
ao da atenc¸
˜
ao prim
´
aria
`
a sa
´
ude,
contribuic¸
˜
oes dos inqu
´
eritos domiciliares e do censo
demogr
´
afico ibge (2022). Ci
ˆ
encia & Sa
´
ude Coletiva,
29(11):e07762024.
Stodden, V. (2020). The data science life cycle: A disci-
plined approach to advancing data science as a sci-
ence. Communications of the ACM, 63(7):58–61.
Tour
´
e, V., Krauss, P., Gnodtke, K., Buchhorn, J., Unni, D.,
Horki, P., Raisaro, J., Kalt, K., Teixeira, D., Crameri,
K., and
¨
Osterle, S. (2023). FAIRification of health-
related data using semantic web technologies in the
Swiss Personalized Health Network. Scientific Data,
10(1).
Wache, H., V
¨
ogele, T., Visser, U., Stuckenschmidt, H.,
Schuster, G., Neumann, H., and H
¨
ubner, S. (2001).
Ontology-based integration of information - a survey
of existing approaches. In Proceedings of the IJCAI-
01 Workshop on Ontologies and Information Sharing,
pages 108–118. CEUR Workshop Proceedings.
Wilkinson, M. D. (2016). Comment: The fair guiding prin-
ciples for scientific data management and stewardship.
Nature Publishing Group.
ICEIS 2025 - 27th International Conference on Enterprise Information Systems
242