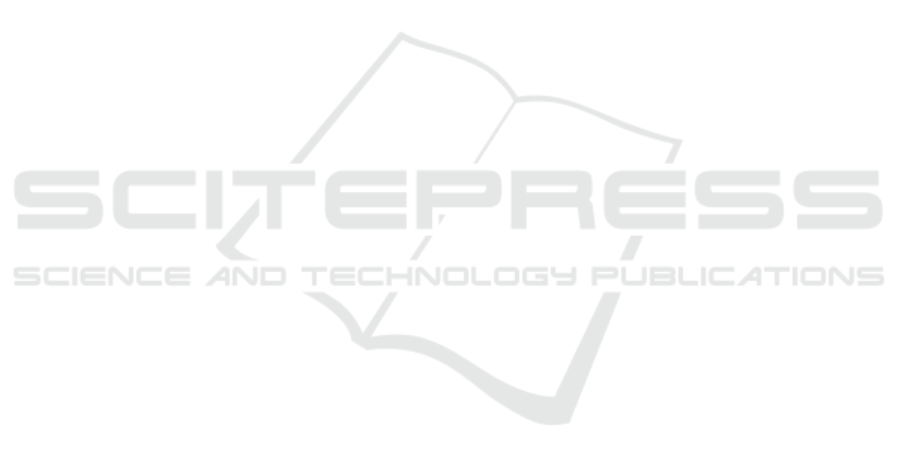
ACKNOWLEDGEMENTS
This study was financed by the Coordenac¸
˜
ao de
Aperfeic¸oamento de Pessoal de N
´
ıvel Superior -
Brasil (CAPES), Brazil - Finance Code 001, and by
the Carlos Chagas Filho Research Support Founda-
tion of the Rio de Janeiro State (FAPERJ), Brazil
(Grants E-26/204.238/2024 and E-26/204.544/2024).
REFERENCES
Alqwadri, A., Azzeh, M., and Almasalha, F. (2021). Appli-
cation of machine learning for online reputation sys-
tems. IJAC, 18(3):492–502.
Chereja, I., Hahn, S. M. L., Matei, O., and Avram, A.
(2021). Operationalizing analytics with newsql. In
Soft. Eng. and Alg., pages 249–263. Springer.
Chevalier, M., El Malki, M., Kopliku, A., Teste, O., and
Tournier, R. (2015). How can we implement a mul-
tidimensional data warehouse using nosql? In EIS,
pages 108–130. Springer.
Civelek, M. E., C¸ emberci, M., and Eralp, N. E. (2016). The
role of social media in crisis communication and crisis
management. IJRBSC, 5(3).
Cole, R. et al. (2011). The mixed workload ch-benchmark.
In IWTDS, pages 1–6.
Corbett, J. C. et al. (2013). Spanner: Google’s globally dis-
tributed database. ACM Trans. Comput. Syst., 31(3).
Cuzzocrea, A., Bellatreche, L., and Song, I.-Y. (2013). Data
warehousing and olap over big data: current chal-
lenges and future research directions. In DOLAP, page
67–70. ACM.
Dageville, B. et al. (2016). The snowflake elastic data ware-
house. In ICMD, page 215–226, NY, USA. ACM.
Dehne, F., Eavis, T., and Rau-Chaplin, A. (2003). Parallel
multi-dimensional rolap index. In ISCCG, pages 86–
93.
Doorley, J. and Garcia, H. F. (2015). Reputation manage-
ment: The key to successful public relations and cor-
porate communication. Routledge.
Fagin, R., Kumar, R., and Sivakumar, D. (2003). Efficient
similarity search and classification via rank aggrega-
tion. In SIGMOD, pages 301–312.
Fan, W. and Gordon, M. D. (2014). The power of social
media analytics. Commun. ACM, 57(6):74–81.
Garcia-Molina, H., Ullman, J., and Widom, J. (2008).
Database Systems. PH, USA, 2 edition.
Golfarelli, M. and Rizzi, S. (2009). Data Warehouse
Design: Modern Principles and Methodologies.
McGraw-Hill Education.
Grolinger, K., Higashino, W. A., Tiwari, A., and Capretz,
M. A. (2013). Data management in cloud environ-
ments: Nosql and newsql data stores. J. of Cloud
Computing: Advances, Sys. and App., 2:1–24.
Huang, D., Liu, Q., Cui, Q., Fang, Z., Ma, X., Xu, F.,
Shen, L., Tang, L., Zhou, Y., Huang, M., Wei, W.,
Liu, C., Zhang, J., Li, J., Wu, X., Song, L., Sun, R.,
Yu, S., Zhao, L., Cameron, N., Pei, L., and Tang, X.
(2020). Tidb: a raft-based htap database. PVLDB,
13(12):3072–3084.
Ingole, A., Khude, P., Kittad, S., Parmar, V., and Ghotkar,
A. (2024). Competitive sentiment analysis for brand
reputation monitoring. In ICETITE, pages 1–7.
Inmon, W. (2005). Building the Data Warehouse. Wiley.
Jeong, J. Y. and Park, N. (2023). Examining the
influence of artificial intelligence on public rela-
tions: Insights from the organization-situation-public-
communication (ospc) model. JCRI, 9(7):485–495.
Kimball, R. and Ross, M. (2013). The Data Warehouse
Toolkit: The Definitive Guide. Wiley.
Li, G. and Zhang, C. (2022). Htap databases: What is new
and what is next. In SIGMOD, page 2483–2488, New
York, NY, USA. ACM.
Liu, P., Yuan, W., Fu, J., Jiang, Z., Hayashi, H., and Neubig,
G. (2023). Pre-train, prompt, and predict: A system-
atic survey of prompting methods in natural language
processing. ACM Comput. Surv., 55(9).
Macnamara, J. (2005). Media content analysis: Its uses,
benefits and best practice methodology. Asia-Pacific
Public Relations J., 6:1.
Marr, B. and Schiuma, G. (2003). Business performance
measurement – past, present and future. Management
Decision, 41(8):680–687.
Morfonios, K., Konakas, S., Ioannidis, Y., and Kotsis, N.
(2007). Rolap implementations of the data cube. ACM
Comput. Surv., 39(4):12–es.
Ramzan, S., Bajwa, I. S., Kazmi, R., and Amna (2019).
Challenges in nosql-based distributed data storage: A
systematic literature review. Electronics, 8(5).
Santa Soriano, A. and Torres Vald
´
es, R. M. (2021). En-
gaging universe 4.0: The case for forming a public
relations-strategic intelligence hybrid. Public Rela-
tions Rev., 47(2):102035.
Taft, R. et al. (2020). Cockroachdb: The resilient
geo-distributed sql database. In SIGMOD, page
1493–1509, NY, USA. ACM.
Ur Rahman, M. W., Shao, S., Satam, P., Hariri, S., Padilla,
C., Taylor, Z., and Nevarez, C. (2022). A BERT-based
Deep Learning Approach for Reputation Analysis in
Social Media . In AICCSA, pages 1–8. IEEE.
Valduriez, P., Jimenez-Peris, R., and
¨
Ozsu, M. T.
(2021). Distributed Database Systems: The Case for
NewSQL, pages 1–15. Springer.
Verbitski, A. et al. (2017). Amazon Aurora: Design con-
siderations for high throughput cloud-native relational
databases. In SIGMOD.
Wang, Y., Cheng, Y., and Sun, J. (2021). When public rela-
tions meets social media: A systematic review of so-
cial media related public relations research from 2006
to 2020. Public Relations Rev., 47(4):102081.
Zhang, Y., Zhang, Y., Wang, S., and Lu, J. (2019). Fusion
olap: Fusing the pros of molap and rolap together for
in-memory olap. TKDE, 31(9):1722–1735.
Zhao, R., Gui, L., Yan, H., and He, Y. (2023). Track-
ing brand-associated polarity-bearing topics in user
reviews. TACL, 11:404–418.
ICEIS 2025 - 27th International Conference on Enterprise Information Systems
62