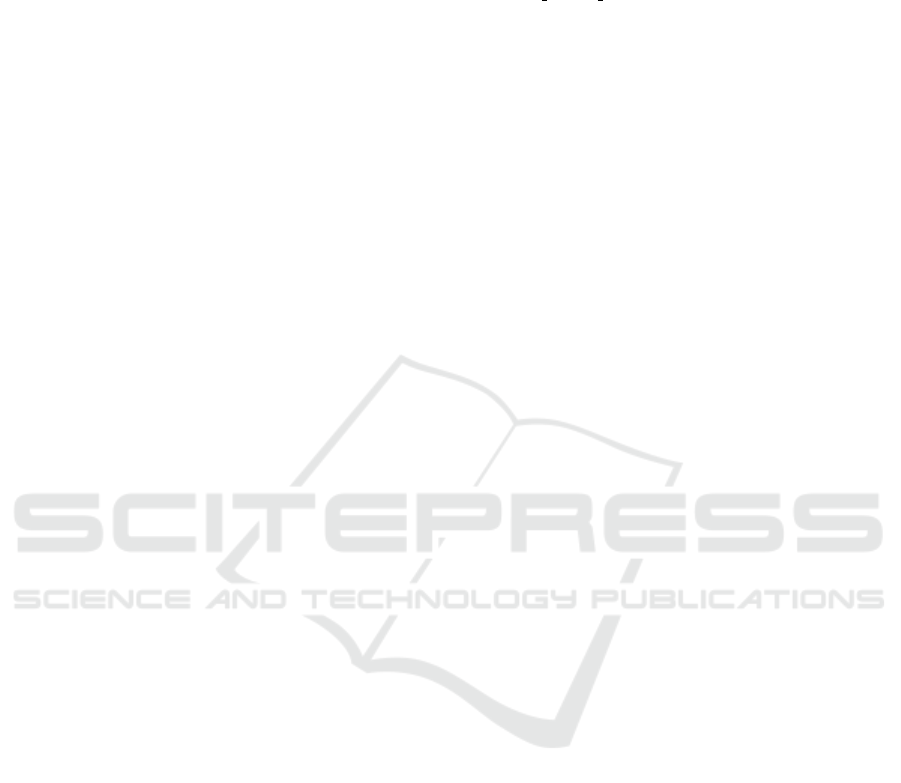
validity analysis to ensure alignment with the target
domain.
Given the process’s reliance on document tran-
scription, we also aim to investigate the impact of
transcription errors on question generation. This anal-
ysis will provide insights into model performance un-
der such conditions and inform strategies to mitigate
these effects. Ultimately, we aim to produce a fully
annotated dataset using the proposed process, estab-
lish baselines with state-of-the-art DocVQA models,
and evaluate the process’s strengths and limitations
for further refinement.
ACKNOWLEDGMENTS
This study was supported by the Coordenac¸
˜
ao de
Aperfeic¸oamento de Pessoal de N
´
ıvel Superior -
Brasil (CAPES) - Finance Code 001.
REFERENCES
Agrawal, M., Hegselmann, S., Lang, H., Kim, Y., and
Sontag, D. (2022). Large language models are few-
shot clinical information extractors. In Goldberg, Y.,
Kozareva, Z., and Zhang, Y., editors, Proceedings of
the 2022 Conference on Empirical Methods in Natural
Language Processing, pages 1998–2022, Abu Dhabi,
United Arab Emirates. Association for Computational
Linguistics.
Analysis, A. (2024). Independent analysis of ai models and
api providers. https://artificialanalysis.ai/. Accessed:
2024-08-20.
Bai, Y., Ying, J., Cao, Y., Lv, X., He, Y., Wang, X., Yu,
J., Zeng, K., Xiao, Y., Lyu, H., et al. (2024). Bench-
marking foundation models with language-model-as-
an-examiner. Advances in Neural Information Pro-
cessing Systems, 36.
Banerjee, A., Biswas, S., Llad
´
os, J., and Pal, U. (2023).
Swindocsegmenter: An end-to-end unified domain
adaptive transformer for document instance segmenta-
tion. In International Conference on Document Anal-
ysis and Recognition, pages 307–325. Springer.
Chen, Y., Zhang, J., Peng, K., Zheng, J., Liu, R., Torr, P.,
and Stiefelhagen, R. (2024). Rodla: Benchmarking
the robustness of document layout analysis models.
arXiv preprint arXiv:2403.14442.
G
¨
obel, M., Hassan, T., Oro, E., and Orsi, G. (2013). Icdar
2013 table competition. In 2013 12th International
Conference on Document Analysis and Recognition,
pages 1449–1453. IEEE.
Kittur, A., Chi, E. H., and Suh, B. (2008). Crowdsourcing
user studies with mechanical turk. In Proceedings of
the SIGCHI conference on human factors in comput-
ing systems, pages 453–456.
Ly, N. T. and Takasu, A. (2023). An end-to-end multi-
task learning model for image-based table recogni-
tion. pages 626–634.
Maik Thiele (2024). documentlayoutsegmenta-
tion yolov8 ondoclaynet (revision 25486d5).
Malaysia-AI (2024). Yolov8x-doclaynet-full-1024-42.
Mathew, M., Bagal, V., Tito, R., Karatzas, D., Valveny, E.,
and Jawahar, C. (2022). Infographicvqa. In Proceed-
ings of the IEEE/CVF Winter Conference on Applica-
tions of Computer Vision, pages 1697–1706.
Mathew, M., Kondreddi, V. K., Biten, A. F., Mafla, A.,
Matas, J., Jawahar, C. V., Valveny, E., and Karatzas,
D. (2021). Docvqa: A dataset for vqa on document
images. In Proceedings of the IEEE/CVF Conference
on Computer Vision and Pattern Recognition (CVPR),
pages 2200–2209.
Nguyen, P., Ly, N. T., Takeda, H., and Takasu, A. (2023).
Tabiqa: Table questions answering on business docu-
ment images. arXiv preprint arXiv:2303.14935.
Pfitzmann, B., Auer, C., Dolfi, M., Nassar, A. S., and Staar,
P. (2022). Doclaynet: a large human-annotated dataset
for document-layout segmentation. In Proceedings
of the 28th ACM SIGKDD Conference on Knowledge
Discovery and Data Mining, pages 3743–3751.
Qiao, L., Li, Z., Cheng, Z., Zhang, P., Pu, S., Niu, Y., Ren,
W., Tan, W., and Wu, F. (2021). Lgpma: compli-
cated table structure recognition with local and global
pyramid mask alignment. In International conference
on document analysis and recognition, pages 99–114.
Springer.
Santos, Y., Silva, M., and Reis, J. C. S. (2023). Evaluation
of optical character recognition (ocr) systems dealing
with misinformation in portuguese. In 2023 36th SIB-
GRAPI Conference on Graphics, Patterns and Images
(SIBGRAPI), pages 223–228.
Smock, B., Pesala, R., and Abraham, R. (2022). Pubtables-
1m: Towards comprehensive table extraction from
unstructured documents. In Proceedings of the
IEEE/CVF Conference on Computer Vision and Pat-
tern Recognition, pages 4634–4642.
Wang, S., Liu, Y., Xu, Y., Zhu, C., and Zeng, M. (2021).
Want to reduce labeling cost? GPT-3 can help. In
Findings of the Association for Computational Lin-
guistics: EMNLP 2021, pages 4195–4205, Punta
Cana, Dominican Republic. Association for Compu-
tational Linguistics.
Ye, J., Qi, X., He, Y., Chen, Y., Gu, D., Gao, P., and
Xiao, R. (2021). Pingan-vcgroup’s solution for ic-
dar 2021 competition on scientific literature parsing
task b: table recognition to html. arXiv preprint
arXiv:2105.01848.
Zheng, X., Burdick, D., Popa, L., Zhong, X., and Wang, N.
X. R. (2021). Global table extractor (gte): A frame-
work for joint table identification and cell structure
recognition using visual context. In Proceedings of
the IEEE/CVF winter conference on applications of
computer vision, pages 697–706.
Zhong, X., ShafieiBavani, E., and Jimeno Yepes, A. (2020).
Image-based table recognition: data, model, and eval-
uation. In European conference on computer vision,
pages 564–580. Springer.
A Data Annotation Approach Using Large Language Models
755