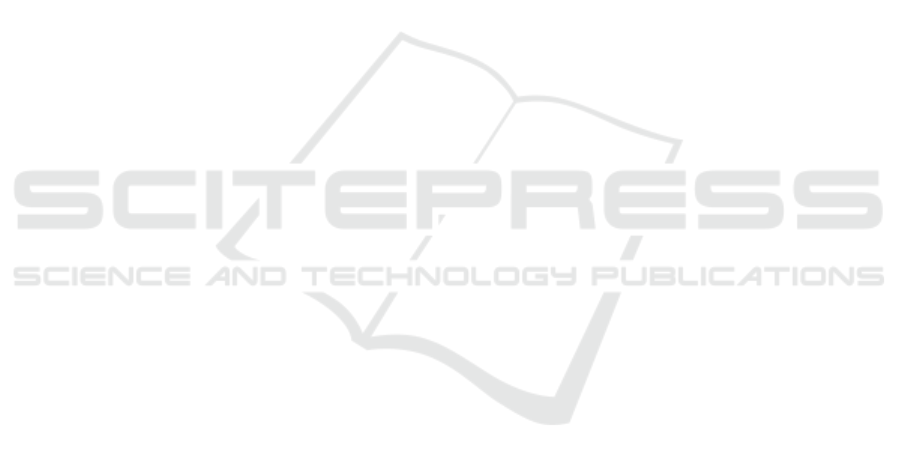
ditional product information, such as manufacturer-
provided technical details, could improve description
accuracy and detail while helping to verify generated
content.
ACKNOWLEDGMENTS
This study was supported by the Coordenac¸
˜
ao de
Aperfeic¸oamento de Pessoal de N
´
ıvel Superior -
Brasil (CAPES) - Finance Code 001.
REFERENCES
Amidei, J., Piwek, P., and Willis, A. (2019). The use of
rating and likert scales in natural language generation
human evaluation tasks: A review and some recom-
mendations.
Amplayo, R. K., Angelidis, S., and Lapata, M.
(2021). Aspect-controllable opinion summarization.
http://arxiv.org/abs/2109.03171.
Brown, T. B. (2020). Language models are few-shot learn-
ers. arXiv preprint arXiv:2005.14165.
Chen, Q., Lin, J., Zhang, Y., Yang, H., Zhou, J., and Tang, J.
(2019). Towards knowledge-based personalized prod-
uct description generation in e-commerce. In Proceed-
ings of the 25th ACM SIGKDD International Confer-
ence on Knowledge Discovery & Data Mining, pages
3040–3050.
Christodoulou, E., Gregoriades, A., Pampaka, M.,
Herodotou, H., Filipe, J., Smialek, M., Brodsky, A.,
and Hammoudi, S. (2021). Application of classifi-
cation and word embedding techniques to evaluate
tourists’ hotel-revisit intention. In ICEIS (1), pages
216–223.
Elad, G., Guy, I., Novgorodov, S., Kimelfeld, B., and
Radinsky, K. (2019). Learning to generate person-
alized product descriptions. In Proceedings of the
28th ACM International Conference on Information
and Knowledge Management, pages 389–398.
Erkan, G. and Radev, D. R. (2004). Lexrank: Graph-based
lexical centrality as salience in text summarization.
Journal of Artificial Intelligence Research, 22:457–
479.
H
¨
utsch, M. and Wulfert, T. (2022). A structured literature
review on the application of machine learning in retail.
ICEIS (1), pages 332–343.
Liang, Y.-S., Chen, C.-Y., Li, C.-T., and Chang, S.-M.
(2024). Personalized product description generation
with gated pointer-generator transformer. IEEE Trans-
actions on Computational Social Systems.
Liu, Y. and Lapata, M. (2019). Hierarchical trans-
formers for multi-document summarization.
http://arxiv.org/abs/1905.13164.
Muennighoff, N., Tazi, N., Magne, L., and Reimers, N.
(2022). Mteb: Massive text embedding benchmark.
EACL 2023 - 17th Conference of the European Chap-
ter of the Association for Computational Linguistics,
Proceedings of the Conference, pages 2006–2029.
Nachar, N. et al. (2008). The mann-whitney u: A test for as-
sessing whether two independent samples come from
the same distribution. Tutorials in quantitative Meth-
ods for Psychology, 4(1):13–20.
Nguyen, M.-T., Nguyen, P.-T., Nguyen, V.-V., and Nguyen,
Q.-M. (2021). Generating product description with
generative pre-trained transformer 2. In 2021 6th in-
ternational conference on innovative technology in in-
telligent system and industrial applications (CITISIA),
pages 1–7. IEEE.
Ni, J., Li, J., and McAuley, J. (2019). Justifying recom-
mendations using distantly-labeled reviews and fine-
grained aspects. EMNLP-IJCNLP 2019 - 2019 Con-
ference on Empirical Methods in Natural Language
Processing and 9th International Joint Conference
on Natural Language Processing, Proceedings of the
Conference, pages 188–197.
Novgorodov, S., Guy, I., Elad, G., and Radinsky, K. (2019).
Generating product descriptions from user reviews. In
The World Wide Web Conference, pages 1354–1364.
ACM.
Novgorodov, S., Guy, I., Elad, G., and Radinsky, K. (2020).
Descriptions from the customers: comparative anal-
ysis of review-based product description generation
methods. ACM Transactions on Internet Technology
(TOIT), 20(4):1–31.
Pedrosa., G., Gardenghi., J., Dias., P., Felix., L., Serafim.,
A., Horinouchi., L., and Figueiredo., R. (2023). A
user-centered approach to analyze public service apps
based on reviews. In Proceedings of the 25th Inter-
national Conference on Enterprise Information Sys-
tems - Volume 1: ICEIS, pages 453–459. INSTICC,
SciTePress.
Puduppully, R., Dong, L., and Lapata, M. (2019). Data-to-
text generation with content selection and planning.
Proceedings of the AAAI Conference on Artificial In-
telligence, 33:6908–6915.
Wang, J., Hou, Y., Liu, J., Cao, Y., and Lin, C.-Y. (2017). A
statistical framework for product description genera-
tion. In Proceedings of the Eighth International Joint
Conference on Natural Language Processing (Volume
2: Short Papers), pages 187–192.
Wang, Z., Zou, Y., Fang, Y., Chen, H., Ma, M., Ding, Z.,
and Long, B. (2022). Interactive latent knowledge
selection for e-commerce product copywriting gen-
eration. In Proceedings of the Fifth Workshop on e-
Commerce and NLP (ECNLP 5), pages 8–19.
Zhan, H., Zhang, H., Chen, H., Shen, L., Ding, Z., Bao,
Y., Yan, W., and Lan, Y. (2021). Probing product
description generation via posterior distillation. Pro-
ceedings of the AAAI Conference on Artificial Intelli-
gence, 35:14301–14309.
Zhang, X., Zou, Y., Zhang, H., Zhou, J., Diao, S., Chen, J.,
Ding, Z., He, Z., He, X., Xiao, Y., Long, B., Yu, H.,
and Wu, L. (2022). Automatic product copywriting
for e-commerce. Proceedings of the AAAI Conference
on Artificial Intelligence, 36:12423–12431.
ICEIS 2025 - 27th International Conference on Enterprise Information Systems
770