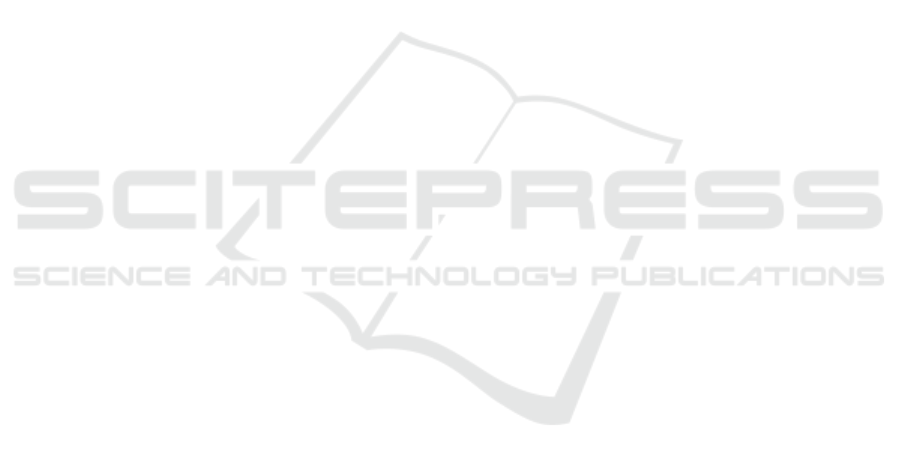
ternational Journal of Human–Computer Interaction,
40(13):3365–3380.
Blanco-R
´
ıos, M. A., Candela-Leal, M. O., Orozco-Romo,
C., Remis-Serna, P., V
´
elez-Saboy
´
a, C. S., Lozoya-
Santos, J. d. J., Cebral-Loureda, M., and Ram
´
ırez-
Moreno, M. A. (2024). Real-time eeg-based emo-
tion recognition for neurohumanities: perspectives
from principal component analysis and tree-based
algorithms. Frontiers in Human Neuroscience,
18:1319574.
Duarte, E. F., Mendoza, Y. L. M., de Queiroz, M. J. N.,
de Oliveira Gaia Pimenta, J., da Silva, J. V., and
Baranauskas, M. C. C. (2022). Aquarela virtual: De-
sign e desenvolvimento de um sistema socioenativo
em contexto de isolamento social. Relat
´
orio t
´
ecnico.
Espinoza Taype, G. E., Dos Reis, J. C., and
Calani Baranauskas, M. C. (2023). A socioen-
active perspective on emotion contagion and senses:
Analyzing the phenomenon via eeg signals. In
Proceedings of the XXII Brazilian Symposium on
Human Factors in Computing Systems, pages 1–11.
Fellous, J.-M. (2004). From human emotions to robot emo-
tions. Architectures for Modeling Emotion: Cross-
Disciplinary Foundations, American Association for
Artificial Intelligence, pages 39–46.
Foody, G. M. and Mathur, A. (2004). A relative evalua-
tion of multiclass image classification by support vec-
tor machines. IEEE Transactions on geoscience and
remote sensing, 42(6):1335–1343.
Gee, N. R., Fine, A. H., and McCardle, P. (2017). How
animals help students learn: Research and practice
for educators and mental-health professionals. Taylor
& Francis.
Gonc¸alves, D. A., Caceffo, R. E., Valente, J. A., and
Baranauskas, M. C. C. (2021). Design of socioe-
nactive systems based on physiological sensors and
robot behavior in educational environments. Revista
Brasileira de Inform
´
atica na Educac¸
˜
ao, 29:1356–
1376.
Huang, Q., Wang, C., Ye, Y., Wang, L., and Xie, N. (2023).
Recognition of eeg based on improved black widow
algorithm optimized svm. Biomedical Signal Process-
ing and Control, 81:104454.
Ivonin, L., Chang, H.-M., Chen, W., and Rauterberg, M.
(2013). Automatic recognition of the unconscious re-
actions from physiological signals. In Human Factors
in Computing and Informatics: First International
Conference, SouthCHI 2013, Maribor, Slovenia, July
1-3, 2013. Proceedings, pages 16–35. Springer.
Jianbiao, M., Xinzui, W., Zhaobo, L., Juan, L., Zhongwei,
Z., and Hui, F. (2023). Eeg signal classification of
tinnitus based on svm and sample entropy. Computer
Methods in Biomechanics and Biomedical Engineer-
ing, 26(5):580–594.
Jiang, L., Stocco, A., Losey, D. M., Abernethy, J. A., Prat,
C. S., and Rao, R. P. (2019). Brainnet: a multi-
person brain-to-brain interface for direct collaboration
between brains. Scientific reports, 9(1):6115.
Jiang, T., Sun, Z., Fu, S., and Lv, Y. (2024). Human-ai inter-
action research agenda: A user-centered perspective.
Data and Information Management, page 100078.
Kaipainen, M., Ravaja, N., Tikka, P., Vuori, R., Pugliese,
R., Rapino, M., and Takala, T. (2011). Enactive sys-
tems and enactive media: embodied human-machine
coupling beyond interfaces. Leonardo, 44(5):433–
438.
Ke, T., Ge, X., Yin, F., Zhang, L., Zheng, Y., Zhang,
C., Li, J., Wang, B., and Wang, W. (2024). A
general maximal margin hyper-sphere svm for multi-
class classification. Expert Systems with Applications,
237:121647.
Lai, Z., Liang, G., Zhou, J., Kong, H., and Lu, Y. (2024).
A joint learning framework for optimal feature ex-
traction and multi-class svm. Information Sciences,
671:120656.
Mathur, A. and Foody, G. M. (2008). Multiclass and binary
svm classification: Implications for training and clas-
sification users. IEEE Geoscience and remote sensing
letters, 5(2):241–245.
Nie, F., Hao, Z., and Wang, R. (2024). Multi-class support
vector machine with maximizing minimum margin. In
Proceedings of the AAAI Conference on Artificial In-
telligence, volume 38, pages 14466–14473.
Pollreisz, D. and TaheriNejad, N. (2017). A simple al-
gorithm for emotion recognition, using physiological
signals of a smart watch. In 2017 39th annual interna-
tional conference of the ieee engineering in medicine
and biology society (EMBC), pages 2353–2356. IEEE.
Rodrigues Filho, C. R. and Nogueira, Y. L. B. (2022). An-
alyzing enactive ai implementations on enactive ai
terms.
Sacc
´
a, V., Campolo, M., Mirarchi, D., Gambardella, A.,
Veltri, P., and Morabito, F. C. (2018). On the clas-
sification of eeg signal by using an svm based algo-
rithm. Multidisciplinary approaches to neural com-
puting, pages 271–278.
Sai, C. Y., Mokhtar, N., Arof, H., Cumming, P., and
Iwahashi, M. (2017). Automated classification and
removal of eeg artifacts with svm and wavelet-ica.
IEEE journal of biomedical and health informatics,
22(3):664–670.
Smith, R. and Lane, R. D. (2015). The neural basis
of one’s own conscious and unconscious emotional
states. Neuroscience & Biobehavioral Reviews, 57:1–
29.
Stenberg, G. (1992). Personality and the eeg: Arousal
and emotional arousability. Personality and Individ-
ual Differences, 13(10):1097–1113.
Uc¸ar, M. K., Nour, M., Sindi, H., and Polat, K. (2020).
The effect of training and testing process on machine
learning in biomedical datasets. Mathematical Prob-
lems in Engineering, 2020(1):2836236.
Wang, K.-J., Zheng, C. Y., Shidujaman, M., Wairagkar,
M., and von Mohr, M. (2020). Jean joseph v2. 0
(remotion): make remote emotion touchable, seeable
and thinkable by direct brain-to-brain telepathy neuro-
haptic interface empowered by generative adversarial
network. In 2020 IEEE International Conference on
Systems, Man, and Cybernetics (SMC), pages 3488–
3493. IEEE.
Remote Emotional Interactions via AI-Enhanced Brain-to-Body Neurophysiological Interface
543