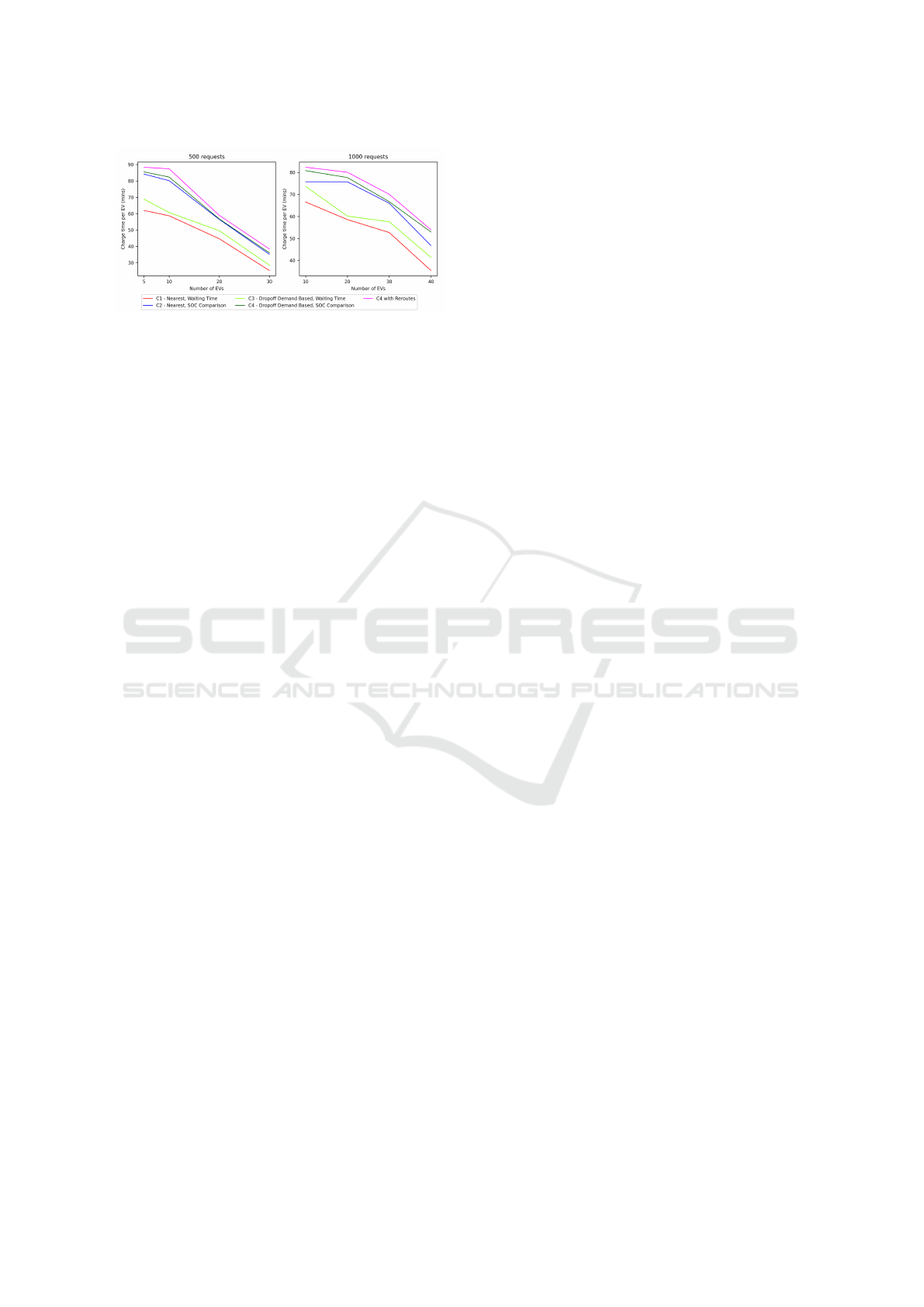
Figure 13: Time spent charging per EV.
Fig. 13 shows the variation in the time spent
charging per EV with the number of EVs. Again, it
can be seen that the average charging time per EV
decreases with an increase in the number of EVs as
expected. Also, time spent charging is higher when
we route the idle EVs because now they have to travel
more, leading to them running out of charge faster and
more frequently, thus leading to more frequent charg-
ing.
6 CONCLUSION
In this paper, we have addressed the problem of man-
agement of an EV fleet for ridesharing to satisfy pas-
senger requests. We presented a set of four algorithms
for the problem and presented detailed simulation re-
sults on a real world dataset to show that the algo-
rithms perform well. The work can be further ex-
tended by considering other objective functions such
as operator profit, passenger’s ride cost etc., and the
use of a set of mobile charging stations owned by the
fleet operator for EV charging to reduce operator cost.
REFERENCES
Chen, T., Zhang, B., Pourbabak, H., Kavousi-Fard, A.,
and Su, W. (2018). Optimal routing and charging of
an electric vehicle fleet for high-efficiency dynamic
transit systems. IEEE Transactions on Smart Grid,
9(4):3563–3572.
Chen, T. D., Kockelman, K. M., and Hanna, J. P. (2016).
Operations of a shared, autonomous, electric vehicle
fleet: Implications of vehicle & charging infrastruc-
ture decisions. Transportation Research Part A: Pol-
icy and Practice, 94:243–254.
Donovan, B. and Work, D. (2016). New
york city taxi trip data (2010-2013).
https://doi.org/10.13012/J8PN93H8.
Guo, G. and Xu, Y. (2022). A deep reinforcement learn-
ing approach to ride-sharing vehicle dispatching in au-
tonomous mobility-on-demand systems. IEEE Intel-
ligent Transportation Systems Magazine, 14(1):128–
140.
Guo, Z., Hao, M., Yu, B., and Yao, B. (2021). Robust
minimum fleet problem for autonomous and human-
driven vehicles in on-demand ride services consider-
ing mixed operation zones. Transportation Research
Part C: Emerging Technologies, 132:103390.
Hua, Y., Zhao, D., Wang, X., and Li, X. (2019). Joint in-
frastructure planning and fleet management for one-
way electric car sharing under time-varying uncertain
demand. Transportation Research Part B: Method-
ological, 128:185–206.
H
¨
all, C., Ceder, A., Ekstr
¨
om, J., and Quttineh, N.-H. (2018).
Adjustments of public transit operations planning pro-
cess for the use of electric buses. Journal of Intelligent
Transportation Systems, 23:1–15.
Kullman, N. D., Cousineau, M., Goodson, J. C., and Men-
doza, J. E. (2022). Dynamic ride-hailing with electric
vehicles. Transportation Science, 56(3):775–794.
Lin, B., Ghaddar, B., and Nathwani, J. (2021). Electric
vehicle routing with charging/discharging under time-
variant electricity prices. Transportation Research
Part C: Emerging Technologies, 130:103285.
Lin, K., Zhao, R., Xu, Z., and Zhou, J. (2018). Efficient
large-scale fleet management via multi-agent deep re-
inforcement learning. In 24th ACM SIGKDD Interna-
tional Conference on Knowledge Discovery & Data
Mining, page 1774–1783.
Lu, J.-L., Yeh, M.-Y., Hsu, Y.-C., Yang, S.-N., Gan, C.-H.,
and Chen, M.-S. (2012). Operating electric taxi fleets:
A new dispatching strategy with charging plans. In
2012 IEEE International Electric Vehicle Conference,
pages 1–8.
Ma, T.-Y. and Fang, Y. (2022). Survey of charging man-
agement and infrastructure planning for electrified
demand-responsive transport systems: Methodologies
and recent developments. European Transport Re-
search Review, 14.
Ma, T.-Y. and Xie, S. (2021). Optimal fast charging sta-
tion locations for electric ridesharing with vehicle-
charging station assignment. Transportation Research
Part D: Transport and Environment, 90:102682.
Mor, A. and Speranza, M. (2020). Vehicle routing problems
over time: a survey. 4OR, 18.
Nissan (2023). Nissan leaf features.
https://www.nissanusa.com/vehicles/electric-
cars/leaf/features.html.
Schiffer, M. and Walther, G. (2017). The electric loca-
tion routing problem with time windows and partial
recharging. European Journal of Operational Re-
search, 260(3):995–1013.
Schiffer, M. and Walther, G. (2018). Strategic planning
of electric logistics fleet networks: A robust location-
routing approach. Omega, 80:31–42.
Shehadeh, K. S., Wang, H., and Zhang, P. (2021). Fleet
sizing and allocation for on-demand last-mile trans-
portation systems. Transportation Research Part C:
Emerging Technologies, 132:103387.
Shi, J., Gao, Y., Wang, W., Yu, N., and Ioannou, P. A.
(2020). Operating electric vehicle fleet for ride-
VEHITS 2025 - 11th International Conference on Vehicle Technology and Intelligent Transport Systems
86