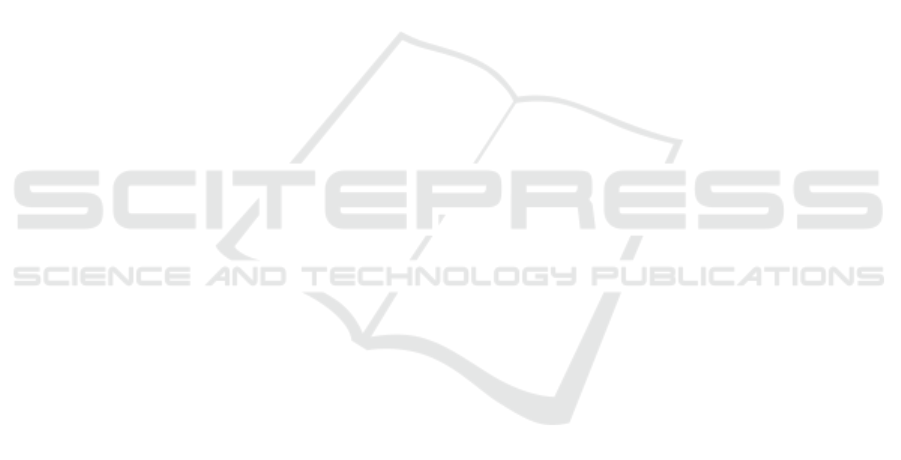
capabilities of a SuT and the purpose of a test. It is es-
sential to carefully choose and adequately document
the parametrization approach to allow for a reliable
statement within a safety assessment or safety assur-
ance argumentation. These recommendations could
be detailed for specific use cases.
ACKNOWLEDGEMENTS
The work of this paper has been done in the context
of the V4SAFETY project which is funded by the Eu-
ropean Commission’s Horizon Europe Research and
Innovation Programme under grant agreement num-
ber 101075068. Views and opinions expressed, are
those of the author(s) only and do not necessarily re-
flect those of the European Union or the European
Climate, Infrastructure and Environment Executive
Agency (CINEA). Neither the European Union nor
the granting authority can be held responsible for
them.
REFERENCES
ASAM e.V. (2024). ASAM OpenSCENARIO® XML Ver-
sion 1.3.0.
Bach, J., Otten, S., and Sax, E. (2016). Model based sce-
nario specification for development and test of auto-
mated driving functions. In 2016 IEEE Intelligent Ve-
hicles Symposium (IV), pages 1149–1155.
Bock, J., Krajewski, R., Moers, T., Runde, S., Vater, L.,
and Eckstein, L. (2020). The ind dataset: A drone
dataset of naturalistic road user trajectories at german
intersections. In 2020 IEEE Intelligent Vehicles Sym-
posium (IV), pages 1929–1934.
de Gelder, E. and Camp, O. O. d. (2024). Scenario-
based assessment of automated driving systems: How
(not) to parameterize scenarios? arXiv preprint
arXiv:2409.01117.
de Gelder, E., Paardekooper, J.-P., Saberi, A. K., Elrofai, H.,
Camp, O. O. d., Kraines, S., Ploeg, J., and De Schut-
ter, B. (2022). Towards an ontology for scenario def-
inition for the assessment of automated vehicles: An
object-oriented framework. IEEE Transactions on In-
telligent Vehicles, 7(2):300–314.
Fahrenkrog, F., Fries, A., Das, A., Kalisvaart, S., Cha-
roniti, E., Op den Camp, O., et al. (2024). Draft
prospective safety assessment framework - method.
Milestone report MS10 of the Horizon Europe project
V4SAFETY.
Galbas, R., Reich, J., Schittenhelm, H., and Wagener, N.
(2022). Safeguarding methods for complex traffic sce-
narios for approval of automated driving functions.
ATZ worldwide, 124(9):56–61.
Geller, C., Haas, B., Kloeker, A., Hermens, J., Lampe, B.,
Beemelmanns, T., and Eckstein, L. (2024). Carlos: An
open, modular, and scalable simulation framework for
the development and testing of software for c-its. In
2024 IEEE Intelligent Vehicles Symposium (IV), pages
3100–3106. IEEE.
Glasmacher, C., Schuldes, M., Topalakatti, P., Hristov, P.,
Weber, H., and Eckstein, L. (2023a). Scenario-based
model of the odd through scenario databases.
Glasmacher, C., Weber, H., Schuldes, M., Wagener, N., and
Eckstein., L. (2023b). Generation of concrete param-
eters from logical urban driving scenarios based on
hybrid graphs. In Proceedings of the 9th Interna-
tional Conference on Vehicle Technology and Intel-
ligent Transport Systems - VEHITS, pages 215–222.
INSTICC, SciTePress.
Hu, M. and Li, Y. (2017). Drivers’ avoidance patterns in
near-collision intersection conflicts. In 2017 IEEE
20th International Conference on Intelligent Trans-
portation Systems (ITSC), pages 1–6.
International Organization for Standardization (2022a).
Road vehicles - safety of the intended functionality.
International Organization for Standardization (2022b).
Road vehicles - test scenarios for automated driving
systems - vocabulary.
Mercedes-Benz AG (2024). Drive pilot technology by
mercedes-benz. Accessed: 2024-11-08.
PEGASUS Project Consortium (2019). Pegasus method -
an overview. Technical report. Accessed: 2024-11-
04.
UNECE (2021). UN Regulation No. 157 on Automated
Lane-Keeping Systems (ALKS). Revision as of Jan-
uary 2023, adopted by UNECE’s World Forum for
Harmonization of Vehicle Regulations.
Waymo LLC (2024). Waymo driver. Accessed: 2024-11-
08.
Weber, H., Glasmacher, C., Schuldes, M., Wagener, N., and
Eckstein, L. (2023a). Holistic driving scenario con-
cept for urban traffic. In 2023 IEEE Intelligent Vehi-
cles Symposium (IV), pages 1–8.
Weber, H., Glasmacher, C., Schuldes, M., Wagener, N., and
Eckstein, L. (2023b). Holistic driving scenario con-
cept for urban traffic. pages 1–8.
Winner, H., Lemmer, K., Form, T., and Mazzega, J. (2018).
PEGASUS—first steps for the safe introduction of au-
tomated driving. In Lecture Notes in Mobility, pages
185–195. Springer International Publishing.
Comparison of Parametrization Approaches for Scenario-Based Testing
447