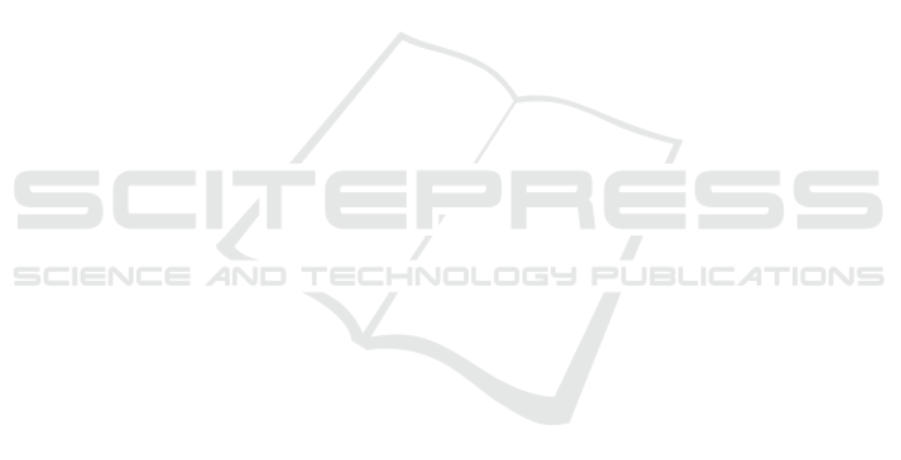
ACKNOWLEDGEMENTS
Kanij and Grundy are supported by ARC Laureate
Fellowship FL190100035. Research funding is also
provided by the Empowerment Charitable Trust and
the Whyte Fund. We thank all the participants and
local collaborators.
REFERENCES
Chen, H. and Nunes, M. B. (2019). Retaining professional
tacit knowledge and evidence of experience through
electronic records management. IADIS International
Journal on Computer Science & Information Systems,
14(2).
Clarke, T. (2010). The Development of a Tacit Knowl-
edge Spectrum based on the Interrelationships be-
tween Tacit and Explicit Knowledge. PhD thesis,
Cardiff Metropolitan University.
Dalkir, K. (2013). Knowledge Management in Theory and
Practice. Routledge.
Das, M., Ray, S., Kumar, U., Begum, S., and Tarafdar,
S. (2015). Livelihood assessment of the fishermen
community in the South West region of Bangladesh.
Journal of Experimental Biology and Agricultural Sci-
ences, 3:353–361.
Fensel, D., S¸ims¸ek, U., Angele, K., Huaman, E., K
¨
arle, E.,
Panasiuk, O., Toma, I., Umbrich, J., and Wahler, A.
(2020). Introduction: What Is a Knowledge Graph?,
chapter 1, pages 1–10. Springer International Publish-
ing, Cham.
Grandinetti, R. (2014). The explicit dimension: What we
could not learn from polanyi. The Learning Organi-
zation, 21(5):333–346.
Han, D. and Han, M. (2023). Unsloth AI: Making AI ac-
cessible to everyone.
Hossain, I., Chamhuri, S., Mokhtar, M., Madan, M., Dey,
M., and Jaafar, A. (2009). Socio-economic condition
of fishermen in seasonal floodplain beels in Rajshahi
District, Bangladesh. Research Journal of Social Sci-
ences, 4:74–81.
Jiang, A. Q., Sablayrolles, A., Mensch, A., Bamford, C.,
Chaplot, D. S., Casas, D. d. l., Bressand, F., Lengyel,
G., Lample, G., Saulnier, L., et al. (2023). Mistral 7b.
Kabir, K., Adhikary, R., Hossain, M. B., and Minar, M. H.
(2012). Livelihood Status of Fishermen of the Old
Brahmaputra River, Bangladesh. World Applied Sci-
ences Journal, 16:869–873.
Kanij, T., Anwar, M., Oliver, G., and Hossain, M. K.
(2023). Developing software for low socio-economic
end users: Lessons learned from a case study of fisher-
folk communities in bangladesh. In 2023 IEEE/ACM
45th International Conference on Software Engineer-
ing: Software Engineering in Society (ICSE-SEIS),
pages 96–107. IEEE.
Ladeinde, A., Arora, C., Khalajzadeh, H., Kanij, T., and
Grundy, J. (2023). Extracting queryable knowledge
graphs from user stories: An empirical evaluation. In
International Conference on Evaluation of Novel Ap-
proaches to Software Engineering 2023, pages 684–
692. Scitepress.
Leonard, D. and Sensiper, S. (1998). The role of tacit
knowledge in group innovation. California manage-
ment review, 40(3):112–132.
Mahmud, S., Ali, M. L., and Ali, M. M. (2015). Present
scenario on livelihood status of the fishermen in the
Paira River, Southern Bangladesh: Constraints and
Recommendation. International Journal of Fisheries
and Aquatic Studies, 2(4):23–30.
Miah, M. and Islam, M. (2020). Defending fishing space:
Conflicts and marginalization of small-scale fisheries
in the Saint Martin’s Island of Bangladesh. In Blue
Justice For Small-Scale Fisheries: A Global Scan,
pages 1–3. TBTI Global Publication Series, St. John’s,
NL, Canada.
Nonaka, I. (2009). The knowledge-creating company. In
The economic impact of knowledge, pages 175–187.
Routledge.
Nonaka, I. and Takeuchi, H. (1995). The Knowledge-
Creating Company: How Japanese Companies Cre-
ate the Dynamics of Innovation. Oxford University
Press.
Nowell, L. S., Norris, J. M., White, D. E., and Moules, N. J.
(2017). Thematic analysis: Striving to meet the trust-
worthiness criteria. International Journal of Qualita-
tive Methods, 16(1):1–13.
Pathirage, C., Amaratunga, D., and Haigh, R. (2008). Tacit
knowledge generation and utilisation in the construc-
tion industry: from process perspective. In Proceed-
ings of the RICS Construction and Building Research
Conference (COBRA 2008).
Polanyi, M. (2009). The tacit dimension. Routledge.
Prasetyo, T., Widodo, P., Saragih, H., Suwarno, P., and Said,
B. (2023). Poverty Reduction For Coastal Communi-
ties In Indonesia Through Community Empowerment
Training. International Journal Of Humanities Edu-
cation and Social Sciences (IJHESS), 2.
Sanderson, M. (2001). Records management and the cap-
ture of tacit knowledge. Records Management Jour-
nal, 11:7–17.
Shuva, N. Z. (2017). The Information Practices of the Fish-
ermen in the Bay of Bengal, Bangladesh. Open Infor-
mation Science, 1(1):21–40.
Supriati, A. and Umar, M. (2020). The role of coastal com-
munities to preserve local wisdom (a study in com-
munity of bunaken island, indonesia). In 2nd Annual
Civic Education Conference (ACEC 2019).
Tran, N., Rodriguez, U.-P., Chan, C. Y., Aung, Y. M., Chu,
L., Islam, A. H. M. S., Barman, B. K., and Phillips,
M. J. (2023). Future scenarios of fish supply and de-
mand for food and nutrition security in Bangladesh:
An analysis with the AsiaFish model. Aquaculture,
568:739288.
An Innovative Approach to Represent Tacit Knowledge of Fishing with Knowledge Graphs
507