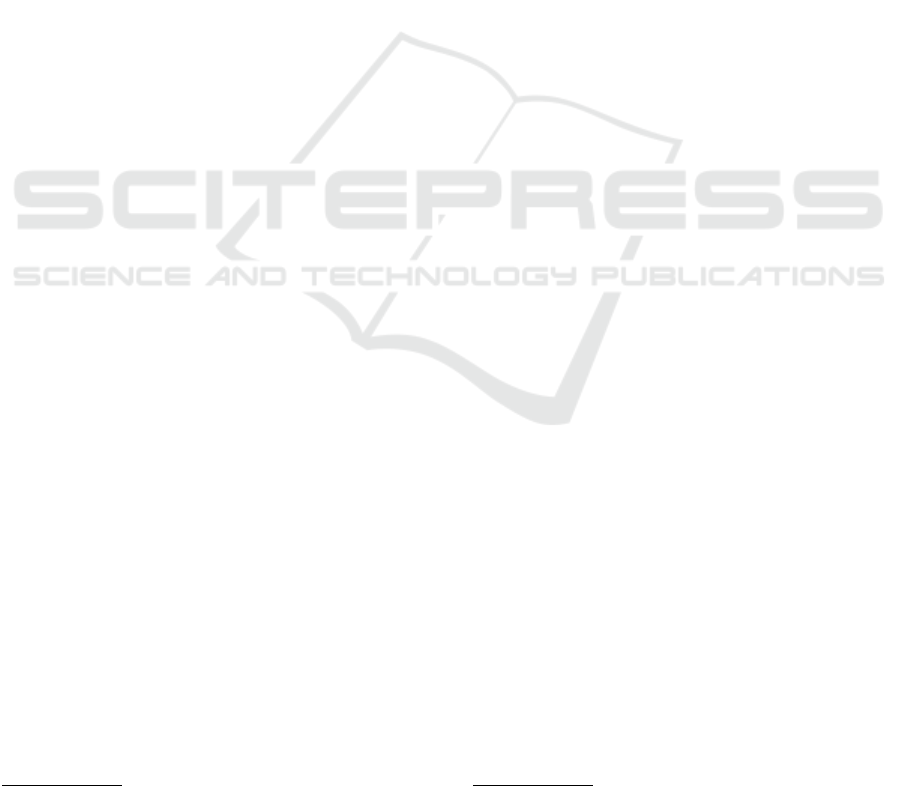
3 RELATED WORK
3.1 Well-Being
Various disciplines (e.g., medicine, psychology, soci-
ology, economics) include research on ”Well-being”.
They mainly focuses on how a single perspective af-
fects the situation of ”Well-being” statistically. From
the education perspective, researchers reported indi-
cators showing that early education can positively im-
pact future well-being (Arthur J. Reynolds, 2011).
From the urbanise perspective, researchers clarified
the inter-relationships between various fundamental
parameters in the design of an urban layout to im-
prove our understanding of urban layouts and the
complicated trade-offs between desirable features and
another (Patel, 2011). From the transport perspective,
researchers built a dynamic model that provides the
most comprehensive and integrated discussion of the
current well-being literature from a transport perspec-
tive (Reardon and Abdallah, 2013). From the medi-
cal perspective, researchers outlined roles that public
health could fulfil, in collaboration with ageing ser-
vices, to address the challenges and opportunities of
an ageing society (Patel, 2011).
However, little research focuses on a multi-
perspective analysis of ”Well-being” from the view of
data analytics. No analysis system adapts well to var-
ious ”Well-being” dimensions or provides decision-
makers with readable, visual reports on current and
future trends. Our research aims to address this by
integrating different datasets related to dimensions of
”Well-being”, such as demographics, population dis-
tribution, land use, transport, infrastructure, and so-
cial and business services. This integration will en-
able comprehensive analyses from multiple perspec-
tives.
3.2 Integration of Spatio-Temporal
Data
Well-being data are generally characterized as spatio-
temporal. The systems analyzing these types of data
are organized into three main modules (Md Mah-
bub Alam and Bifet, 2022): (1) data storage, which
includes both spatial relational database management
system and NoSQL databases (Felix Gessert and Rit-
ter, 2017); (2) data processing, which encompasses
big data infrastructure sorted by architecture types
(e.g., Hadoop
5
, Spark
6
, NoSQL (Ali Davoudian and
Liu, 2018)) and data processing systems (e.g., spatial
5
https://hadoop.apache.org/
6
http://spark.apache.org/
(Ahmed Eldawy and Mokbel, 2017), spatio-temporal
(Nidzwetzki and G
¨
uting, 2019), trajectory (Xin Ding
and Bao, 2018)); and (3) data programming and
software tools, covering libraries and software like
R, Python (Zhang and Eldawy, 2020), ArcGIS
7
and
QGIS
8
that support processing of spatial and spatio-
temporal data.
Considering the integration of spatio-temporal
data, data from different sources could have distinct
spatial and temporal resolutions, which leads to dif-
ferent spatial and temporal granularity. In terms
of space, new data are usually at a higher resolu-
tion than old data due to technological developments,
e.g., aerial photographs, satellite imagery or other re-
motely sensed data. At the same time, the spatial res-
olution of different data sources may vary, for exam-
ple, highway data are usually specific to geographic
points, while weather-related data are mostly by city.
In terms of time, data such as rivers and lakes, admin-
istrative boundaries, and roads have a relatively low
temporal resolution and can be considered static; data
such as weather is usually updated hourly; and traffic
conditions, for example, may change within seconds
(Le, 2012). The data that will be used for analyses of
”Well-being” include structured data, semi-structured
data and non-structured data. Meanwhile, since we
are in real-world applications, there is a large amount
of spatio-temporal information which is often vague
or ambiguous with low quality due to missing values,
high data redundancy, and untruthfulness (Luyi Bai
and Bai, 2021). Therefore, we can conclude that we
are dealing with standard heterogeneous data (Wang,
2017).
Considering the big data scenario for ”Well-
being” data, data lakes (DL) are considered a use-
ful data storage method. Data lakes emerge as a big
data repository that stores raw data and provides a rich
list of features with the help of metadata descriptions
(Khine and Wang, 2018). Data ingestion is simple as
there is no need for a data schema or ETL (Extract-
transform-load) process design. It is also horizon-
tally and vertically scalable as there is no fixed data
schema. Therefore, Data Lake is a perfect solution
for heterogeneous data with various types and granu-
larity.
7
https://www.arcgis.com/index.html
8
https://www.qgis.org
ENASE 2025 - 20th International Conference on Evaluation of Novel Approaches to Software Engineering
82