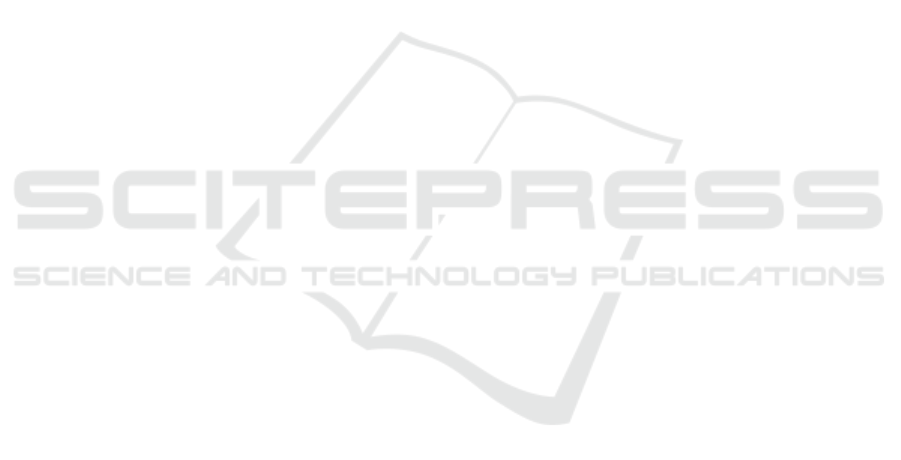
Azeroual, O. and Nacheva, R. (2023). Data mesh for man-
aging complex big data landscapes and enhancing de-
cision making in organizations. In KMIS, pages 202–
212.
Badewitz, W., Kloker, S., and Weinhardt, C. (2020). The
data provision game: researching revenue sharing in
collaborative data networks. In 2020 IEEE 22nd
Conference on Business Informatics (CBI), volume 1,
pages 191–200. IEEE.
Barney, J. (1991). Firm resources and sustained competitive
advantage. Journal of management, 17(1):99–120.
Bertsekas, D. and Gallager, R. (2021). Data networks.
Athena Scientific.
Bi, Y., Wu, Y., Liu, J., Ren, K., and Xiong, L. (2024).
When data pricing meets non-cooperative game the-
ory. In 2024 IEEE 40th International Conference on
Data Engineering (ICDE), pages 5548–5559. IEEE.
Bil
`
o, V., Bove, L., and Vinci, C. (2023a). Utility-sharing
games: How to improve the efficiency with limited
subsidies. In Proceedings of the 24th Italian Confer-
ence on Theoretical Computer Science, 2023, volume
3587, pages 314–327.
Bil
`
o, V., Ferraioli, D., and Vinci, C. (2022). General opin-
ion formation games with social group membership.
In Proceedings of the Thirty-First International Joint
Conference on Artificial Intelligence, IJCAI 2022,
pages 88–94.
Bil
`
o, V., Gourv
`
es, L., and Monnot, J. (2023b). Project
games. Theor. Comput. Sci., 940(Part):97–111.
Bogomolnaia, A. and Jackson, M. O. (2002). The stability
of hedonic coalition structures. Games and Economic
Behavior, 38(2):201–230.
Braganza, A., Brooks, L., Nepelski, D., Ali, M., and Moro,
R. (2017). Resource management in big data initia-
tives: Processes and dynamic capabilities. Journal of
Business Research, 70:328–337.
Catalano, A. A., Catalano, C., Del Vecchio, V., and Lazoi,
M. (2024). Big Data Analytics and Visualization So-
lutions in Industry: a Literature Review. Proceedings
IFKAD 2024.
Cavanillas, J. M., Curry, E., and Wahlster, W. (2016). The
big data value opportunity. New horizons for a data-
driven economy: A roadmap for usage and exploita-
tion of big data in Europe, pages 3–11.
Chen, H.-M., Kazman, R., Garbajosa, J., and Gonzalez,
E. (2017). Big data value engineering for business
model innovation. In 50th Hawaii International Con-
ference on System Sciences, HICSS 2017, Hawaii,
USA. ScholarSpace / AIS Electronic Library (AISeL).
Chen, M., Mao, S., and Liu, Y. (2014). Big data: A survey.
Mobile networks and applications, 19:171–209.
Cheng, W., Lian, W., and Tian, J. (2022). Building the
hospital intelligent twins for all-scenario intelligence
health care. Digital Health, 8:20552076221107894.
Corallo, A., Crespino, A. M., Del Vecchio, V., Gervasi, M.,
Lazoi, M., and Marra, M. (2023). Evaluating maturity
level of big data management and analytics in indus-
trial companies. Technological Forecasting and Social
Change, 196:122826.
Corallo, A., Fortunato, L., Massafra, A., Pasca, P., An-
gelelli, M., Hobbs, M., Al-Nasser, A. D., Al-Omari,
A. I., and Ciavolino, E. (2020). Sentiment analysis of
expectation and perception of MILANO EXPO2015
in twitter data: a generalized cross entropy approach.
Soft Computing, 24:13597–13607.
Coyle, D. and Manley, A. (2024). What is the value of data?
a review of empirical methods. Journal of Economic
Surveys, 38(4):1317–1337.
Dehghani, Z. (2022). Data mesh: Delivering data-driven
value at scale. O’Reilly Media, Inc., 1.ed - preview
version.
Del Vecchio, P., Di Minin, A., Petruzzelli, A. M., Panniello,
U., and Pirri, S. (2018). Big data for open innovation
in smes and large corporations: Trends, opportunities,
and challenges. Creativity and Innovation Manage-
ment, 27(1):6–22.
Dong, J. Q. and Yang, C.-H. (2020). Business value
of big data analytics: A systems-theoretic approach
and empirical test. Information & Management,
57(1):103124.
Faroukhi, A., El Alaoui, I., Gahi, Y., and Amine, A. (2020).
A multi-layer big data value chain approach for secu-
rity issues. Procedia Computer Science, 175:737–744.
The 17th International Conference on Mobile Systems
and Pervasive Computing (MobiSPC),The 15th Inter-
national Conference on Future Networks and Commu-
nications (FNC),The 10th International Conference on
Sustainable Energy Information Technology.
Fast, V., Schnurr, D., and Wohlfarth, M. (2021). Data-
driven competitive advantages in digital markets: An
overview of data value and facilitating factors. In
Ahlemann, F., Sch
¨
utte, R., and Stieglitz, S., editors,
Innovation Through Information Systems, pages 454–
454, Cham. Springer International Publishing.
Geerts, G. and O’Leary, D. (2022). V-matrix: A wave the-
ory of value creation for big data. International Jour-
nal of Accounting Information Systems, 47:100575.
Gervasi, M., Totaro, N. G., Fornaio, A., and Caivano, D.
(2023a). Big data value graph: Enhancing security
and generating new value from big data. In Pro-
ceedings of the Italian conference on cyber security
(ITASEC 2023), volume 3488. CEUR-WS, Bari.
Gervasi, M., Totaro, N. G., Specchia, G., and Latino, M. E.
(2023b). Unveiling the roots of big data project
failure: a critical analysis of the distinguishing fea-
tures and uncertainties in evaluating big data potential
value. In Proceedings of the 2nd Italian conference
on big data and data science (ITADATA 2023), vol-
ume 3606. CEUR-WS, Naples.
Graef, I., Tombal, T., and De Streel, A. (2019). Limits
and enablers of data sharing. an analytical framework
for eu competition, data protection and consumer law.
SSRN Electronic Journal.
Grander, G., Da Silva, L. F., Gonzalez, E. D. R. S., and
Penha, R. (2022). Framework for structuring big data
projects. Electronics, 11(21):3540.
Gupta, M. and George, J. F. (2016). Toward the develop-
ment of a big data analytics capability. Information &
Management, 53(8):1049–1064.
Data Network Game: Enabling Collaboration via Data Mesh
91