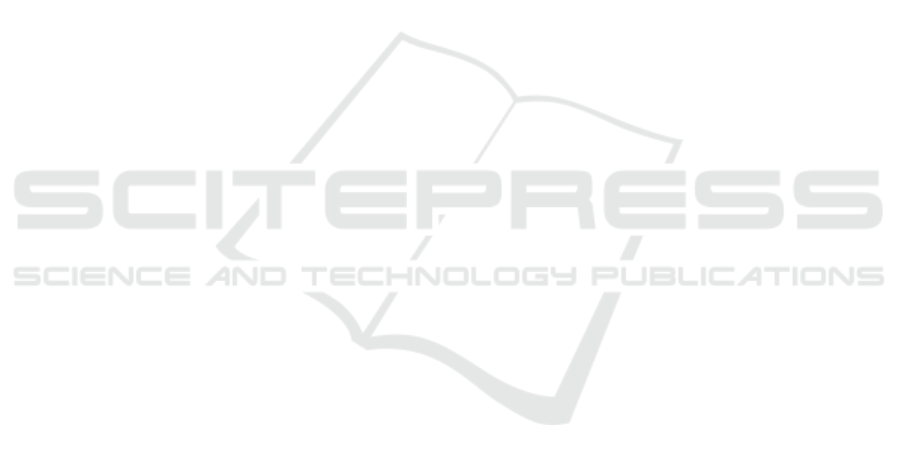
the 32nd International ACM SIGIR Conference on Re-
search and Development in Information Retrieval, SI-
GIR ’09, page 758–759, New York, NY, USA. Asso-
ciation for Computing Machinery.
De Oliveira, A. B., Baptista, C. d. S., Firmino, A. A., and
De Paiva, A. C. (2024). A large language model ap-
proach to detect hate speech in political discourse us-
ing multiple language corpora. In Proceedings of the
39th ACM/SIGAPP Symposium on Applied Comput-
ing, SAC ’24, page 1461–1468, New York, NY, USA.
Association for Computing Machinery.
de Santana, M. A., de Souza Baptista, C., Alves, A.
L. F., Firmino, A. A., da Silva Janu
´
ario, G., and
da Silva Caldera, R. W. (2023). Using machine
learning and NLP for the product matching problem.
In Intelligent Sustainable Systems, pages 439–448.
Springer Nature Singapore.
Ebraheem, M., Thirumuruganathan, S., Joty, S. R., Ouz-
zani, M., and Tang, N. (2017). Deeper - deep entity
resolution. CoRR, abs/1710.00597.
Embar, V., Sisman, B., Wei, H., Dong, X. L., Faloutsos,
C., and Getoor, L. (2020). Contrastive entity linkage:
Mining variational attributes from large catalogs for
entity linkage. In Automated Knowledge Base Con-
struction.
G
¨
oz
¨
ukara, F. and
¨
Ozel, S. A. (2021). An incremental
hierarchical clustering based system for record link-
age in e-commerce domain. The Computer Journal,
66(3):581–602.
Hambarde, K. A. and Proenc¸a, H. (2023). Information re-
trieval: Recent advances and beyond. IEEE Access,
11:76581–76604.
Han, J., Pei, J., and Tong, H., editors (2023). Data Mining:
Concepts and Techniques. Morgan Kaufmann, fourth
edition edition.
Konda, P. V. (2018). Magellan: Toward building entity
matching management systems. The University of
Wisconsin-Madison.
K
¨
opcke, H., Thor, A., and Rahm, E. (2010). Evaluation
of entity resolution approaches on real-world match
problems. Proceedings of the VLDB Endowment, 3(1-
2):484–493.
Li, Y., Li, J., Suhara, Y., Doan, A., and Tan, W.-C. (2020).
Deep entity matching with pre-trained language mod-
els. Proceedings of the VLDB Endowment, 14(1):50–
60.
Mudgal, S., Li, H., Rekatsinas, T., Doan, A., Park, Y., Kr-
ishnan, G., Deep, R., Arcaute, E., and Raghavendra,
V. (2018). Deep Learning for Entity Matching. In
Proceedings of the 2018 International Conference on
Management of Data, pages 19–34, New York, NY,
USA. ACM.
Papadakis, G., Skoutas, D., Thanos, E., and Palpanas, T.
(2021). Blocking and Filtering Techniques for Entity
Resolution. ACM Computing Surveys, 53(2):1–42.
Peeters, R. and Bizer, C. (2022). Supervised contrastive
learning for product matching. In Companion Pro-
ceedings of the Web Conference 2022, WWW ’22,
page 248–251, New York, NY, USA. Association for
Computing Machinery.
Peeters, R., Bizer, C., and Glava
ˇ
s, G. (2020). Interme-
diate training of bert for product matching. small,
745(722):2–112.
Pikuliak, M.,
ˇ
Simko, M., and Bielikova, M. (2021). Cross-
lingual learning for text processing: A survey. Expert
Systems with Applications, 165:113765.
Primpeli, A., Peeters, R., and Bizer, C. (2019). The WDC
training dataset and gold standard for large-scale prod-
uct matching. In Companion Proceedings of The 2019
World Wide Web Conference. ACM.
Rateria, S. and Singh, S. (2024). Transparent, low resource,
and context-aware information retrieval from a closed
domain knowledge base. IEEE Access, 12:44233–
44243.
Reimers, N. and Gurevych, I. (2019). Sentence-bert: Sen-
tence embeddings using siamese bert-networks.
Ristoski, P., Petrovski, P., Mika, P., and Paulheim, H.
(2018). A machine learning approach for prod-
uct matching and categorization. Semantic web,
9(5):707–728.
Tracz, J., W
´
ojcik, P. I., Jasinska-Kobus, K., Belluzzo, R.,
Mroczkowski, R., and Gawlik, I. (2020). BERT-based
similarity learning for product matching. Proceedings
of Workshop on Natural Language Processing in E-
Commerce, pages 66–75.
Traeger, L., Behrend, A., and Karabatis, G. (2024). Scop-
ing: Towards streamlined entity collections for multi-
sourced entity resolution with self-supervised agents.
In Proceedings of the 26th International Conference
on Enterprise Information Systems - Volume 1: ICEIS,
pages 107–115. INSTICC, SciTePress.
Xiao, C., Wang, W., Lin, X., Yu, J. X., and Wang, G. (2011).
Efficient similarity joins for near-duplicate detection.
ACM Trans. Database Syst., 36(3).
ICEIS 2025 - 27th International Conference on Enterprise Information Systems
74