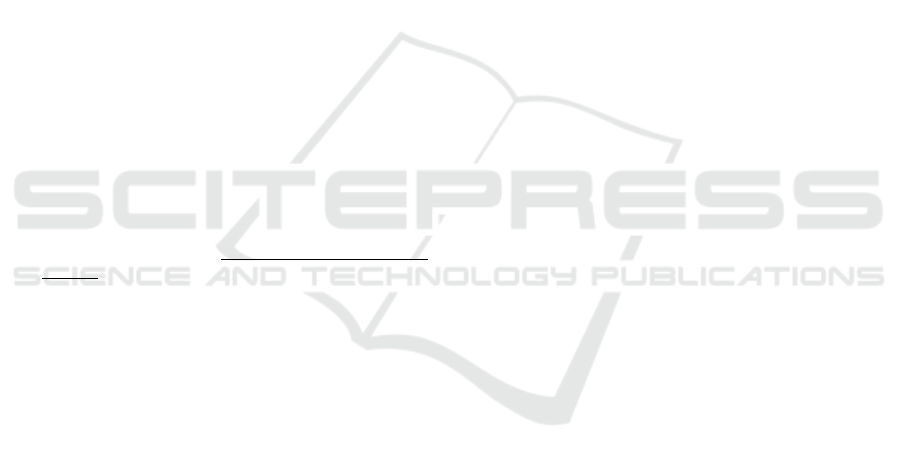
Josko, J. M. B., Ehrlinger, L., and W
¨
oß, W. (2019). To-
wards a knowledge graph to describe and process data
defects. DBKDA 2019, 65.
Kim, W., Choi, B.-J., Hong, E., Kim, S.-K., and Lee, D.
(2003). A taxonomy of dirty data. Data Min. Knowl.
Discov., 7:81–99.
Le Quy, T., Roy, A., Iosifidis, V., Zhang, W., and Ntoutsi, E.
(2022). A survey on datasets for fairness-aware ma-
chine learning. WIREs Data Mining and Knowledge
Discovery, 12(3):e1452.
Li, L., Peng, T., and Kennedy, J. (2011). A rule based tax-
onomy of dirty data. GSTF INTERNATIONAL JOUR-
NAL ON COMPUTING, 1.
Liebchen, G. and Shepperd, M. (2008). Data sets and data
quality in software engineering. Proceedings - Inter-
national Conference on Software Engineering.
Mansouri, T., Moghadam, M. R. S., Monshizadeh, F.,
and Zareravasan, A. (2021). Iot data quality issues
and potential solutions: A literature review. CoRR,
abs/2103.13303.
Melegati, J., Chanin, R., Sales, A., Prikladnicki, R., and
Wang, X. (2020). MVP and experimentation in soft-
ware startups: a qualitative survey. In 46th Euromicro
Conference on Software Engineering and Advanced
Applications, SEAA 2020, Portoroz, Slovenia, August
26-28, 2020, pages 322–325. IEEE.
Melegati, J., Chanin, R., Wang, X., Sales, A., and Prik-
ladnicki, R. (2019). Enablers and inhibitors of ex-
perimentation in early-stage software startups. In
Franch, X., M
¨
annist
¨
o, T., and Mart
´
ınez-Fern
´
andez, S.,
editors, Product-Focused Software Process Improve-
ment - 20th International Conference, PROFES 2019,
Barcelona, Spain, November 27-29, 2019, Proceed-
ings, volume 11915 of Lecture Notes in Computer
Science, pages 554–569. Springer.
Munappy, A., Bosch, J., Olsson, H. H., Arpteg, A., and
Brinne, B. (2019). Data management challenges for
deep learning. In 2019 45th Euromicro Conference
on Software Engineering and Advanced Applications
(SEAA), pages 140–147.
Munappy, A. R., Bosch, J., and Olsson, H. H. (2020). Data
pipeline management in practice: Challenges and op-
portunities. In Product-Focused Software Process Im-
provement: 21st International Conference, PROFES
2020, Turin, Italy, November 25–27, 2020, Proceed-
ings, page 168–184, Berlin, Heidelberg. Springer-
Verlag.
Nascimento, N., Santos, A. R., Sales, A., and Chanin, R.
(2020). Behavior-driven development: A case study
on its impacts on agile development teams. In ICSE
’20: 42nd International Conference on Software En-
gineering, Workshops, Seoul, Republic of Korea, 27
June - 19 July, 2020, pages 109–116. ACM.
Oliveira, P., Rodrigues, F., Rangel Henriques, P., and Gal-
hardas, H. (2005). A taxonomy of data quality prob-
lems. Journal of Data and Information Quality -
JDIQ.
Recupito, G., Pecorelli, F., Catolino, G., Moreschini, S.,
Nucci, D. D., Palomba, F., and Tamburri, D. A.
(2022). A multivocal literature review of mlops tools
and features. In 2022 48th Euromicro Conference
on Software Engineering and Advanced Applications
(SEAA), pages 84–91.
Recupito, G., Rapacciuolo, R., Di Nucci, D., and Palomba,
F. (2024). Unmasking data secrets: An empirical in-
vestigation into data smells and their impact on data
quality. In Proceedings of the IEEE/ACM 3rd Inter-
national Conference on AI Engineering - Software En-
gineering for AI, CAIN ’24, page 53–63, New York,
NY, USA. Association for Computing Machinery.
Roman, D., Pultier, A., Ma, X., Soylu, A., and Ulyashin,
A. G. (2022). Data quality issues in solar panels in-
stallations: a case study. In Proceedings of the 2nd
International Workshop on Software Engineering and
AI for Data Quality in Cyber-Physical Systems/Inter-
net of Things, SEA4DQ 2022, page 24–25, New York,
NY, USA. Association for Computing Machinery.
Santos, J. A. M., Rocha-Junior, J. B., Prates, L.
C. L., Do Nascimento, R. S., Freitas, M. F., and
De Mendonc¸a, M. G. (2018). A systematic review
on the code smell effect. Journal of Systems and Soft-
ware, 144:450–477.
Sato, D., Wider, A., and Windheuser, C. (2019).
Continuous delivery for machine learning.
https://martinfowler.com/articles/cd4ml.html.
Sculley, D., Holt, G., Golovin, D., Davydov, E., Phillips,
T., Ebner, D., Chaudhary, V., Young, M., Crespo, J.-
F., and Dennison, D. (2015). Hidden technical debt in
machine learning systems. In Cortes, C., Lawrence,
N., Lee, D., Sugiyama, M., and Garnett, R., editors,
Advances in Neural Information Processing Systems,
volume 28. Curran Associates, Inc.
Shome, A., Cruz, L., and van Deursen, A. (2022). Data
smells in public datasets. In Proceedings of the 1st In-
ternational Conference on AI Engineering: Software
Engineering for AI, CAIN ’22, page 205–216, New
York, NY, USA. Association for Computing Machin-
ery.
Sukhobok, D., Nikolov, N., and Roman, D. (2017). Tab-
ular data anomaly patterns. In 2017 International
Conference on Big Data Innovations and Applications
(Innovate-Data), pages 25–34.
Ter Hofstede, A. H. M., Koschmider, A., Marrella, A., An-
drews, R., Fischer, D. A., Sadeghianasl, S., Wynn,
M. T., Comuzzi, M., De Weerdt, J., Goel, K., Mar-
tin, N., and Soffer, P. (2023). Process-data quality:
The true frontier of process mining. J. Data and In-
formation Quality, 15(3).
Van Emden, E. and Moonen, L. (2012). Assuring software
quality by code smell detection. In 2012 19th Working
Conference on Reverse Engineering, pages xix–xix.
Citeseer.
Wang, H. and Abraham, Z. (2015). Concept drift detection
for streaming data. In 2015 international joint confer-
ence on neural networks (IJCNN), pages 1–9. IEEE.
Yoon, K.-A. and Bae, D.-H. (2010). A pattern-based out-
lier detection method identifying abnormal attributes
in software project data. Information and Software
Technology, 52(2):137–151.
Zhang, H., Cruz, L., and Van Deursen, A. (2022). Code
smells for machine learning applications. In Proceed-
ings of the 1st international conference on AI engi-
neering: software engineering for AI, pages 217–228.
ICEIS 2025 - 27th International Conference on Enterprise Information Systems
488